Color Separated Restoration for Lightweight Single Image Super-Resolution.
Artificial Intelligence and Cloud Computing Conference (AICCC)(2021)
摘要
Recently, single image super-resolution (SISR) has remarkably progressed because of deep convolutional neural network (CNN) based methods. AsCNNarchitectures growdeeper and wider, they achieve considerable reconstruction quality of super-resolved image. However, these very deep CNN have high computational costs, including large memory usage and slow inference speed. To address these issues, numerous lightweight and efficient SISR methods have been proposed, but they have limitation because of small number of network parameters. To improve the capability of lightweight networks, we introduce a new framework (color separated restoration framework) that separately reconstructs each color channel. However, this separation constrains the full use of information in an image; thus, we develop a color content fusion (CCF) layer to solve this problem. The CCF layer efficiently fuses separated features and generates favorable features for each color from the fused features. We also propose attention-based feature decomposition, which enables effectively to reduce the number of parameters by dividing features into two parts while enriching feature representation with an attention mechanism. Extensive experimental results show the superiority of our methods over other state-of-the-art lightweight SR methods.
更多查看译文
关键词
single image super-resolution, convolutional neural networks, lightweight networks
AI 理解论文
溯源树
样例
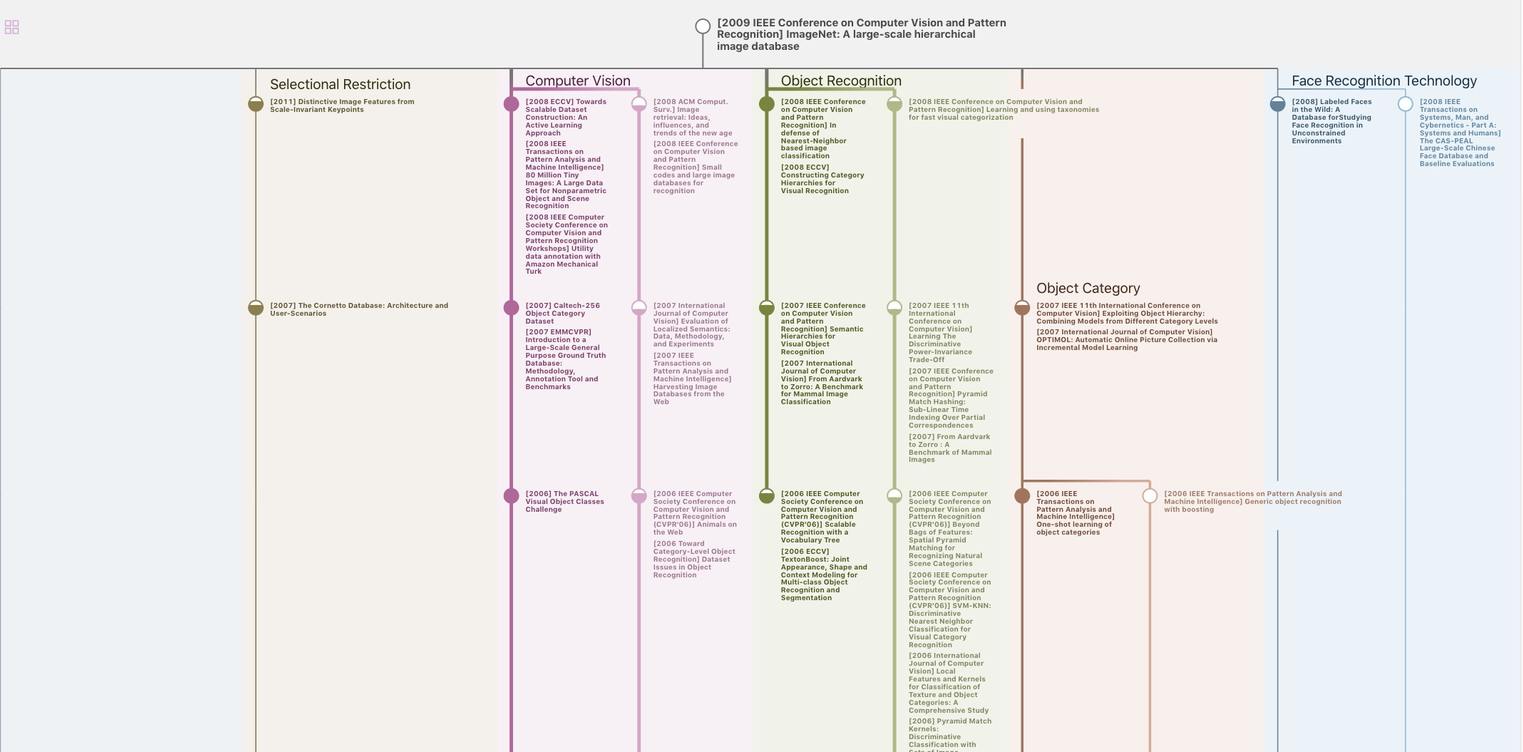
生成溯源树,研究论文发展脉络
Chat Paper
正在生成论文摘要