Swin MAE: Masked Autoencoders for Small Datasets
Computers in Biology and Medicine(2022)
Abstract
The development of deep learning models in medical image analysis is majorly limited by the lack of large-sized and well-annotated datasets. Unsupervised learning does not require labels and is more suitable for solving medical image analysis problems. However, most of the current unsupervised learning methods need to be applied to large datasets. To make unsupervised learning applicable to small datasets, we proposed Swin MAE, which is a masked autoencoder with Swin Transformer as its backbone. Even on a dataset of only a few thousand medical images and without using any pre-trained models, Swin MAE is still able to learn useful semantic features purely from images. It can equal or even slightly outperform the supervised model obtained by Swin Transformer trained on ImageNet in terms of the transfer learning results of downstream tasks. The code will be publicly available soon.
MoreTranslated text
Key words
autoencoders
AI Read Science
Must-Reading Tree
Example
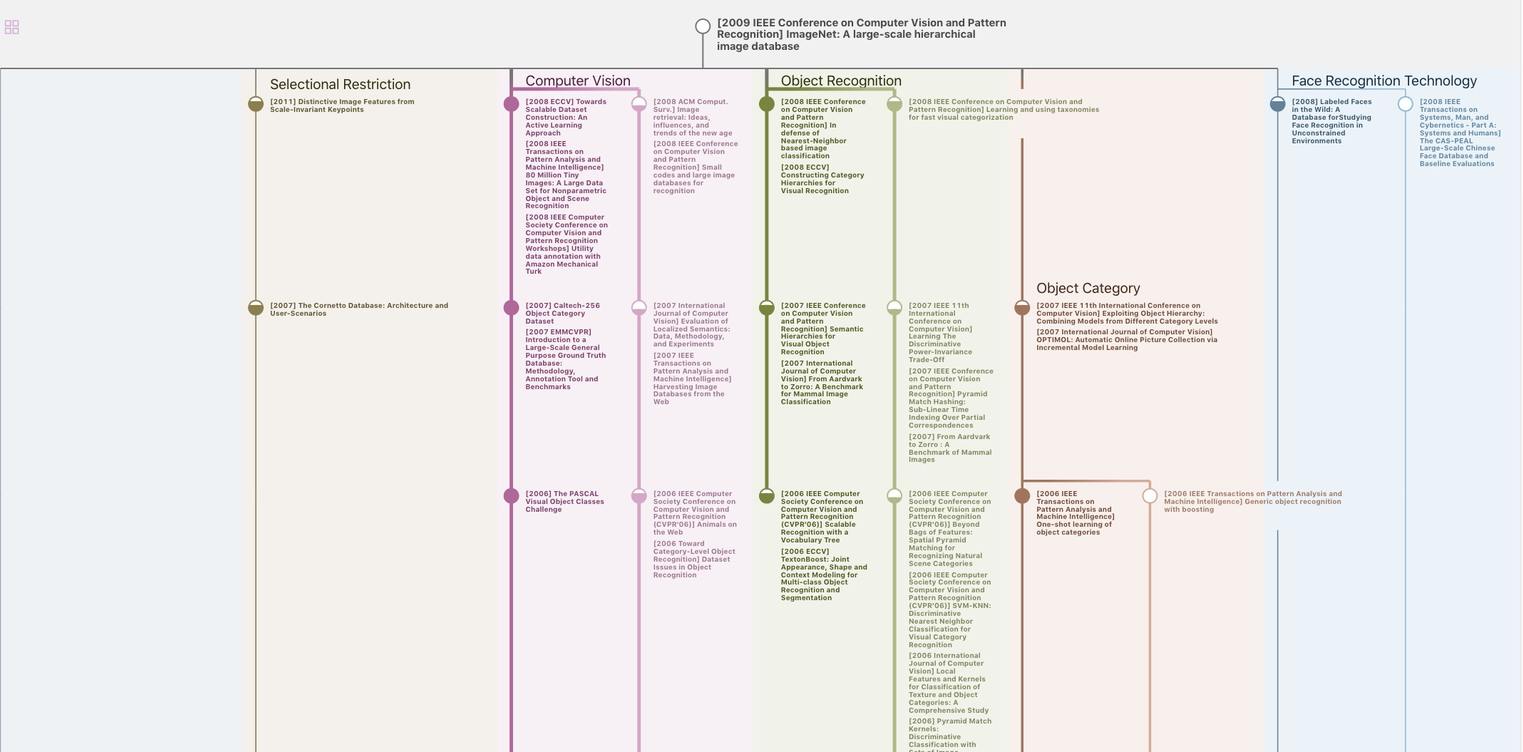
Generate MRT to find the research sequence of this paper
Chat Paper
Summary is being generated by the instructions you defined