Scalable Diffusion Models with Transformers
Proceedings of the IEEE/CVF International Conference on Computer Vision (ICCV)(2023)
摘要
We explore a new class of diffusion models based on the transformer architecture. We train latent diffusion models of images, replacing the commonly-used U-Net backbone with a transformer that operates on latent patches. We analyze the scalability of our Diffusion Transformers (DiTs) through the lens of forward pass complexity as measured by Gflops. We find that DiTs with higher Gflops---through increased transformer depth/width or increased number of input tokens---consistently have lower FID. In addition to possessing good scalability properties, our largest DiT-XL/2 models outperform all prior diffusion models on the class-conditional ImageNet 512x512 and 256x256 benchmarks, achieving a state-of-the-art FID of 2.27 on the latter.
更多查看译文
关键词
Diffusion MRI,Fiber Tractography
AI 理解论文
溯源树
样例
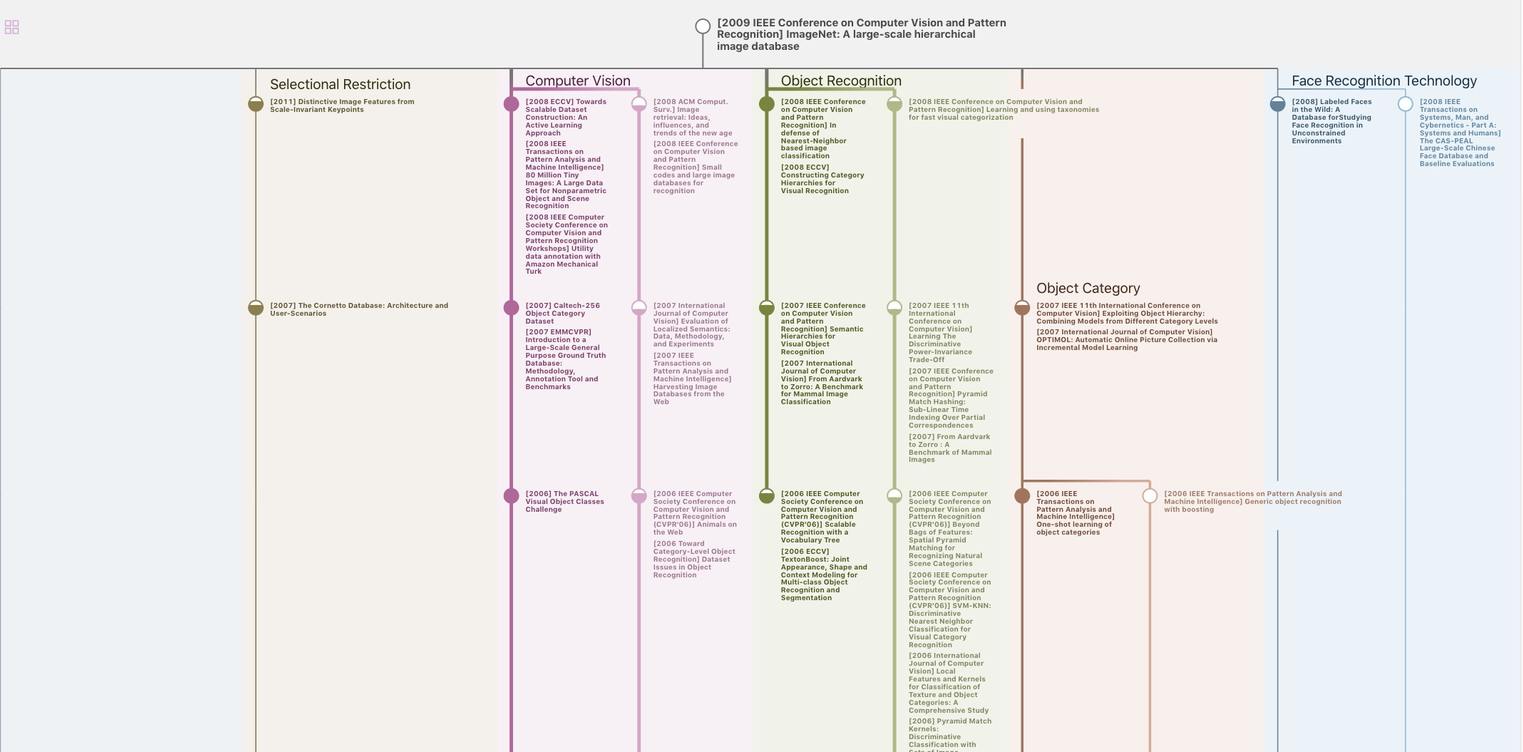
生成溯源树,研究论文发展脉络
Chat Paper
正在生成论文摘要