CASVM: an Efficient Deep Learning Image Classification Method Combined with SVM
Applied sciences(2022)
摘要
Recent advances in convolutional neural networks (CNNs) for image feature extraction have achieved extraordinary performance, but back-propagation algorithms tend to fall into local minima. To alleviate this problem, this paper proposes a coordinate attention-support vector machine-convolutional neural network (CASVM). This proposed to enhance the model’s ability by introducing coordinate attention while obtaining enhanced image features. Training is carried out by back-propagating the loss function of support vector machines (SVMs) to improve the generalization capability, which can effectively avoid falling into local optima. The image datasets used in this study for benchmark experiments are Fashion-MNIST, Cifar10, Cifar100, and Animal10. Experimental results show that compared with softmax, CASVM can improve the image classification accuracy of the original model under different image resolution datasets. Under the same structure, CASVM shows better performance and robustness and has higher accuracy. Under the same network parameters, the loss function of CASVM enables the model to realize a lower loss value. Among the standard CNN models, the highest accuracy rate can reach 99%, and the optimal number of accuracy indicators is 5.5 times that of softmax, whose accuracy rate can be improved by up to 56%.
更多查看译文
关键词
CASVM models,coordinate attention,feature learning method
AI 理解论文
溯源树
样例
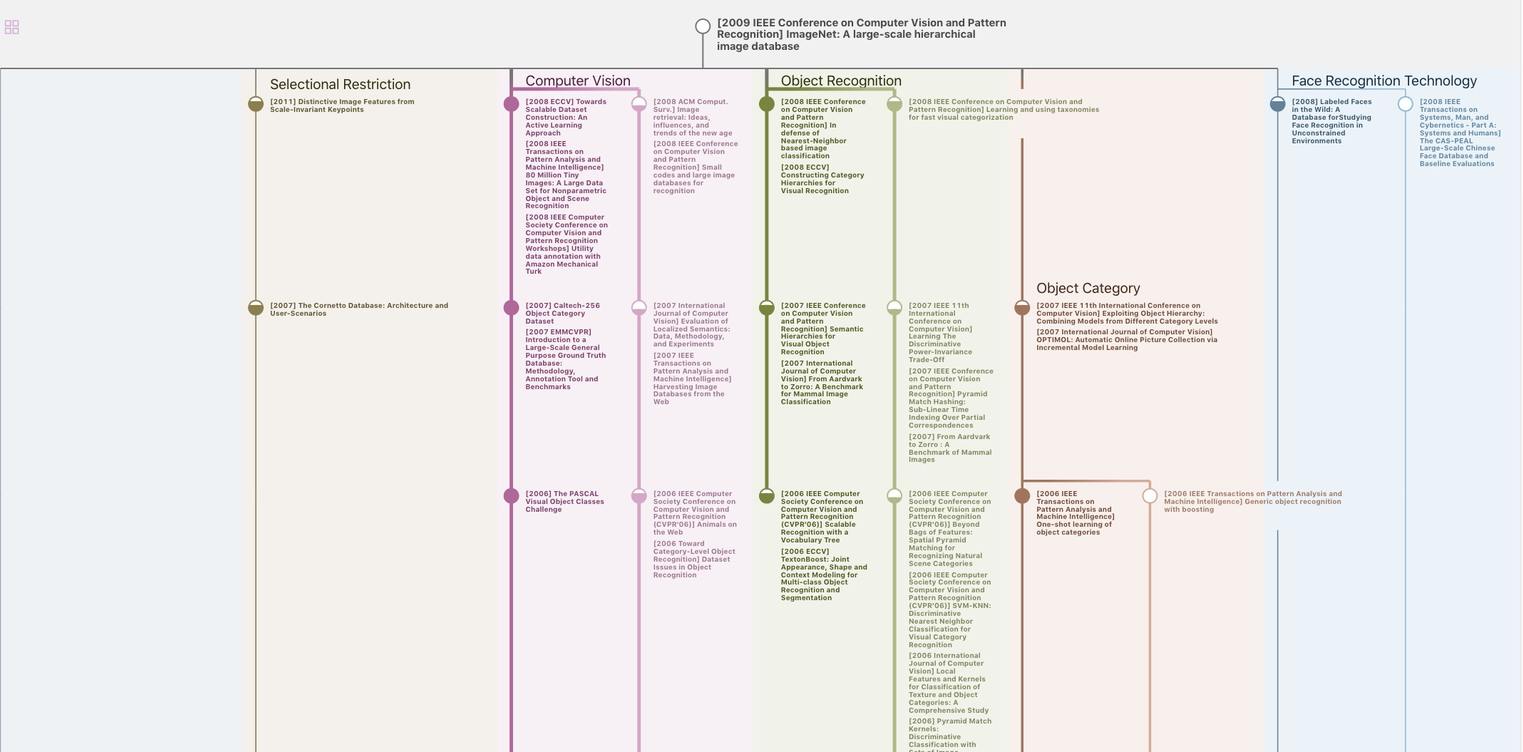
生成溯源树,研究论文发展脉络
Chat Paper
正在生成论文摘要