Small Data Least-Squares Transformation (sd-LST) for Fast Calibration of SSVEP-Based BCIs
IEEE Transactions on Neural Systems and Rehabilitation Engineering(2023)
摘要
Steady-state visual evoked potential (SSVEP) is one of the most popular brain-computer interface (BCI) paradigms, with high information transmission rate and signal-to-noise ratio. Many calibration-free and calibration-based approaches have been proposed to improve the performance of SSVEP-based BCIs. This paper considers a quick calibration scenario, where there are plenty of data from multiple source subjects, but only a small number of calibration trials from a subset of stimulus frequencies for the new subject. We propose small data least-squares transformation (sd-LST) to solve this problem. Experiments on three publicly available SSVEP datasets demonstrated that sd-LST outperformed several classical or state-of-the-art approaches, with only about 10 calibration trials for 40-target SSVEP-based BCI spellers.
更多查看译文
关键词
Calibration,Electroencephalography,Correlation,Visualization,Task analysis,Time-frequency analysis,Standards,Brain-computer interface,steady-state visual evoked potential,transfer learning,least-squares transformation
AI 理解论文
溯源树
样例
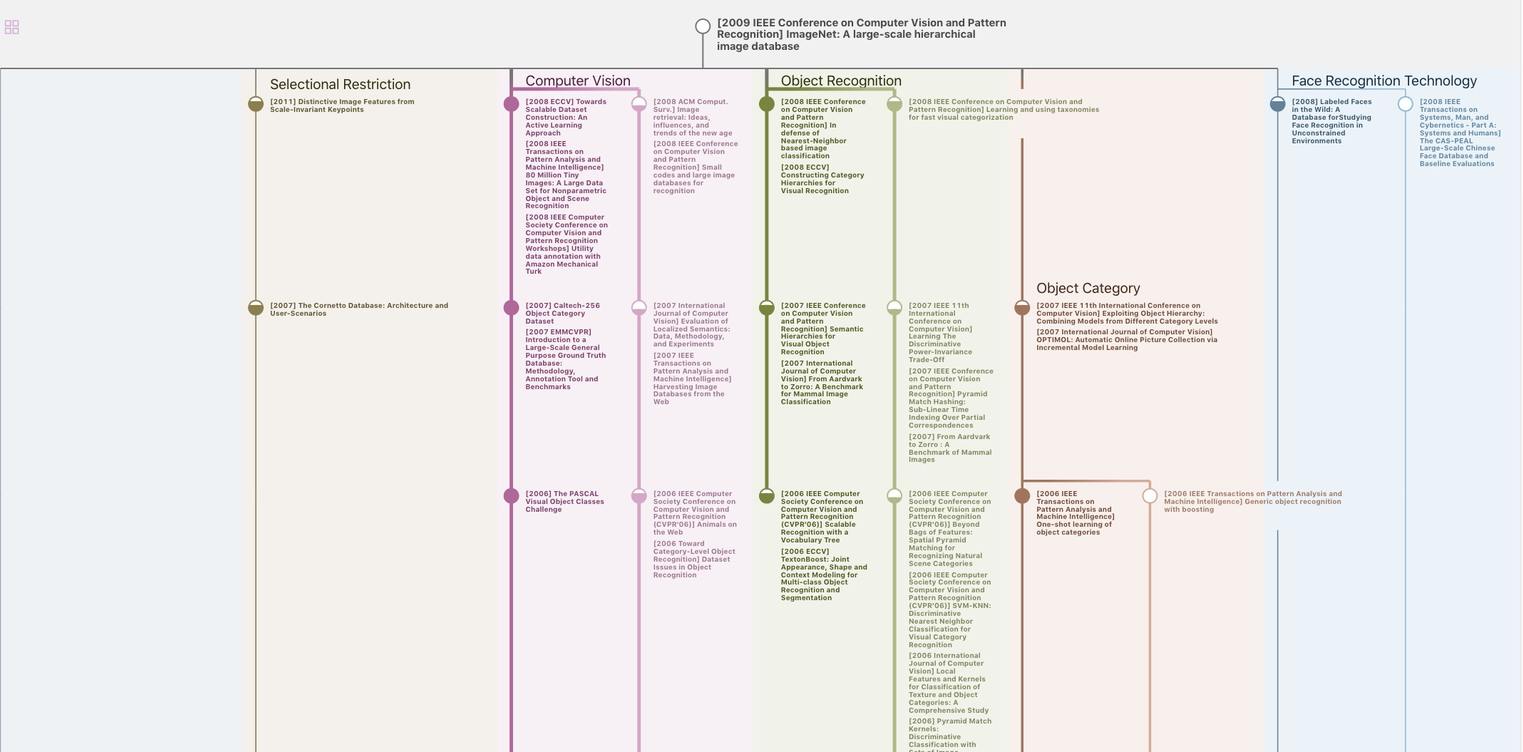
生成溯源树,研究论文发展脉络
Chat Paper
正在生成论文摘要