Symmetry Detection in Trajectory Data for More Meaningful Reinforcement Learning Representations
CoRR(2022)
摘要
Knowledge of the symmetries of reinforcement learning (RL) systems can be used to create compressed and semantically meaningful representations of a low-level state space. We present a method of automatically detecting RL symmetries directly from raw trajectory data without requiring active control of the system. Our method generates candidate symmetries and trains a recurrent neural network (RNN) to discriminate between the original trajectories and the transformed trajectories for each candidate symmetry. The RNN discriminator's accuracy for each candidate reveals how symmetric the system is under that transformation. This information can be used to create high-level representations that are invariant to all symmetries on a dataset level and to communicate properties of the RL behavior to users. We show in experiments on two simulated RL use cases (a pusher robot and a UAV flying in wind) that our method can determine the symmetries underlying both the environment physics and the trained RL policy.
更多查看译文
关键词
symmetry,trajectory data,reinforcement
AI 理解论文
溯源树
样例
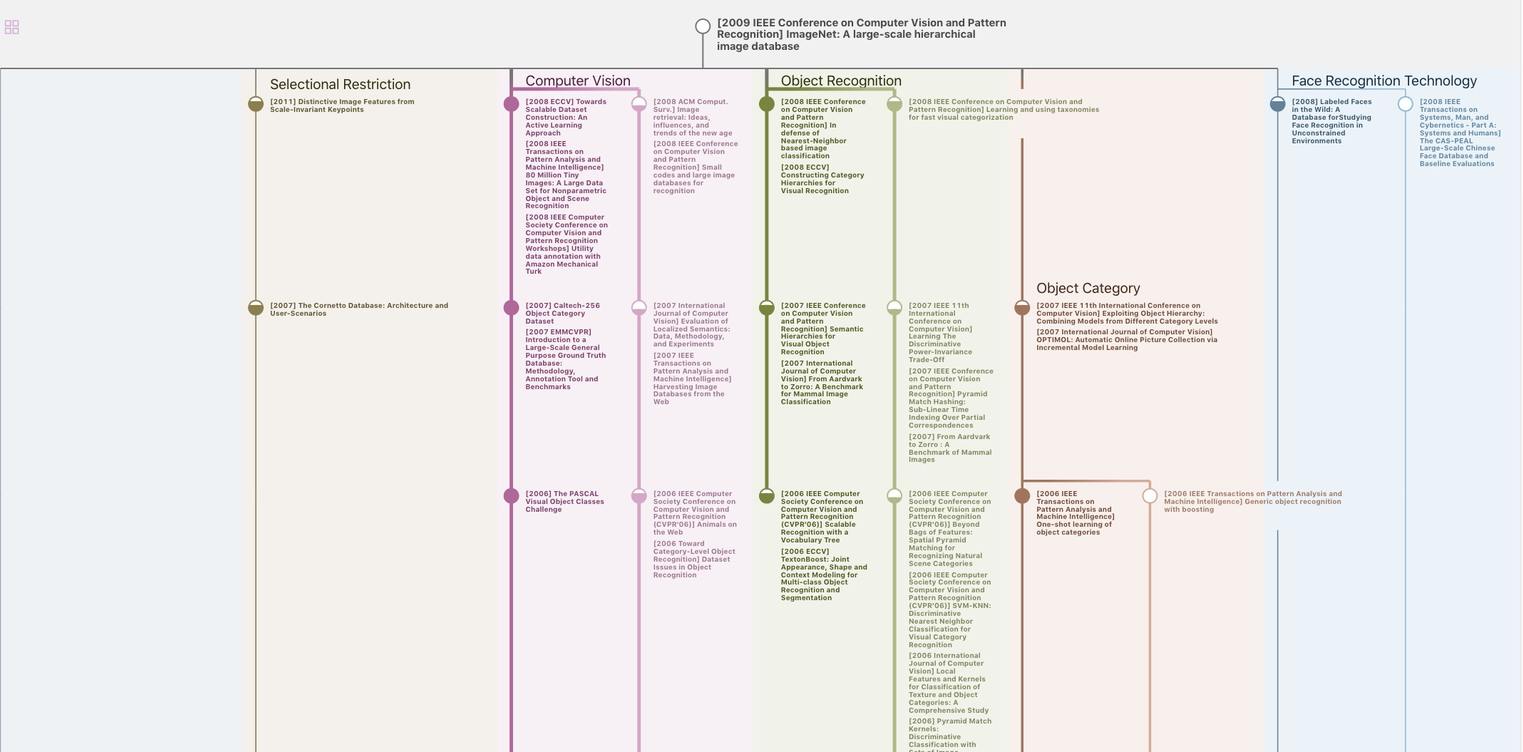
生成溯源树,研究论文发展脉络
Chat Paper
正在生成论文摘要