Improving Risk Stratification Using AI and Social Determinants of Health.
The American journal of managed care(2022)
摘要
OBJECTIVES:To determine whether a risk prediction model using artificial intelligence (AI) to combine multiple data sources, including claims data, demographics, social determinants of health (SDOH) data, and admission, discharge, and transfer (ADT) alerts, more accurately identifies high-cost members than traditional models.STUDY DESIGN:The study used data from a Medicaid accountable care organization and included a population of 61,850 members continuously enrolled between May 2018 and April 2019.METHODS:Risk scores generated by 2 models were estimated for each member. One model, developed by Medical Home Network, used AI to analyze SDOH data, ADT activity, and claims and demographic characteristics, whereas the other model (Chronic Illness and Disability Payment System [CDPS]) relied only on demographic and claims information. To compare models, we calculated mean, median, and total spending for members with the highest 5% of AI risk scores and compared these with spending metrics for members with the highest 5% of CDPS scores. We also compared the number of members with the highest 5% of costs prospectively identified by each model as highest risk. We segmented the population by length of prior enrollment to control for varying levels of claims experience.RESULTS:The AI model consistently identified a higher proportion of the highest-spending members. Members deemed highest risk by the AI model also had higher spending than members deemed highest risk by the CDPS model.CONCLUSIONS:Identification of high-cost members can be improved by using AI to combine traditional sources of data (eg, claims and demographic information) with nontraditional sources (eg, SDOH, admission alerts).
更多查看译文
AI 理解论文
溯源树
样例
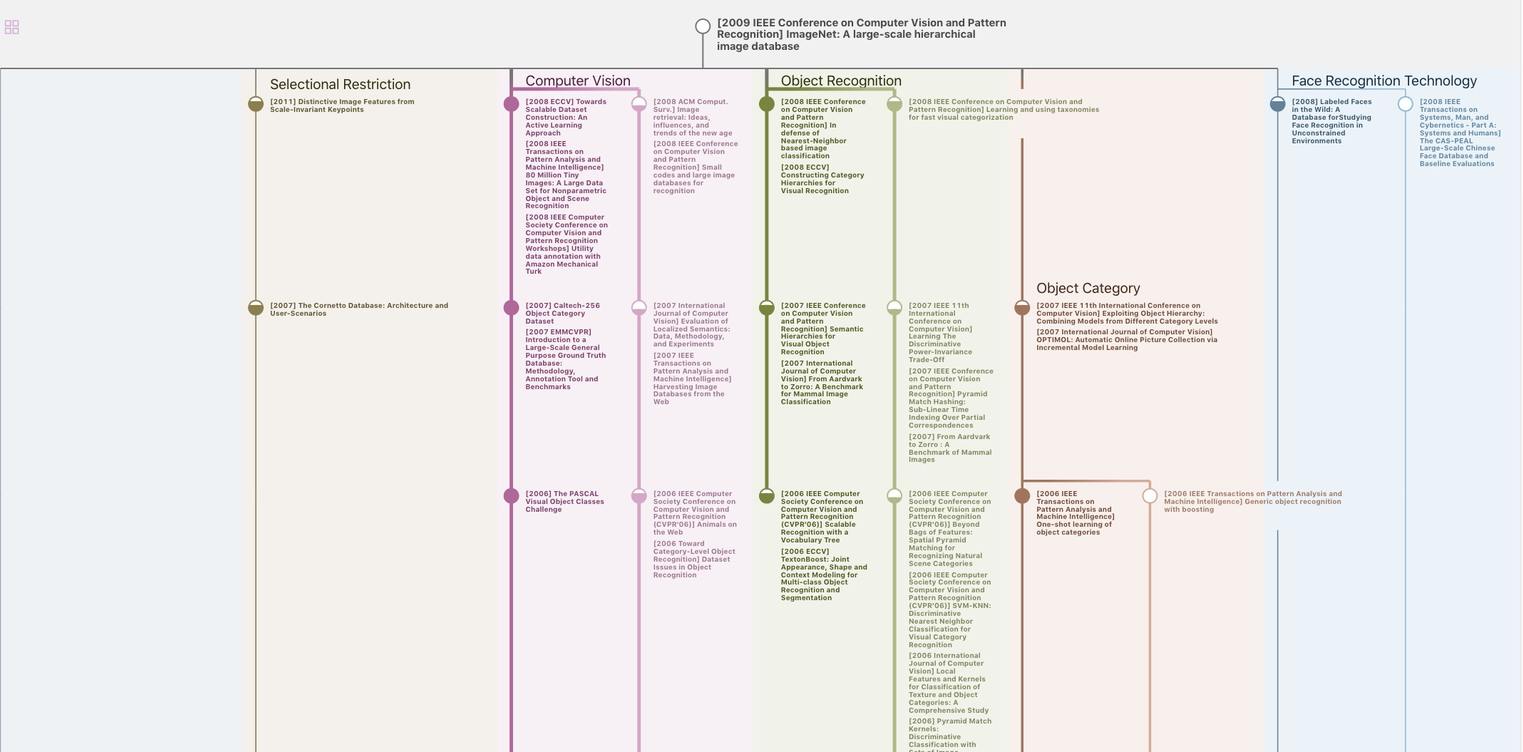
生成溯源树,研究论文发展脉络
Chat Paper
正在生成论文摘要