CMS: A Computational Memory Solution for High-Performance and Power-Efficient Recommendation System
2022 IEEE 4th International Conference on Artificial Intelligence Circuits and Systems (AICAS)(2022)
摘要
This paper proposes a cost-effective and scalable memory solution for high-performance and power-efficient recommendation systems, called computational memory solution (CMS). To address the memory bandwidth challenges of the deep learning-based recommendation system, CMS offloads memory-intensive embedding operations to near-data processors equipped with large capacity and high bandwidth memory. In contrast to the other state-of-the-art near-memory processing accelerators that only support inference and have scalability restrictions, CMS supports training and scales as much as the high-speed serial interface allows. Our evaluation results show that CMS achieves up to $7.5\times$ higher throughput and $12.2\times$ higher power efficiency for training than state-of-the-art accelerators.
更多查看译文
关键词
deep learning,heterogeneous system,memory solution,near data processing,recommendation system
AI 理解论文
溯源树
样例
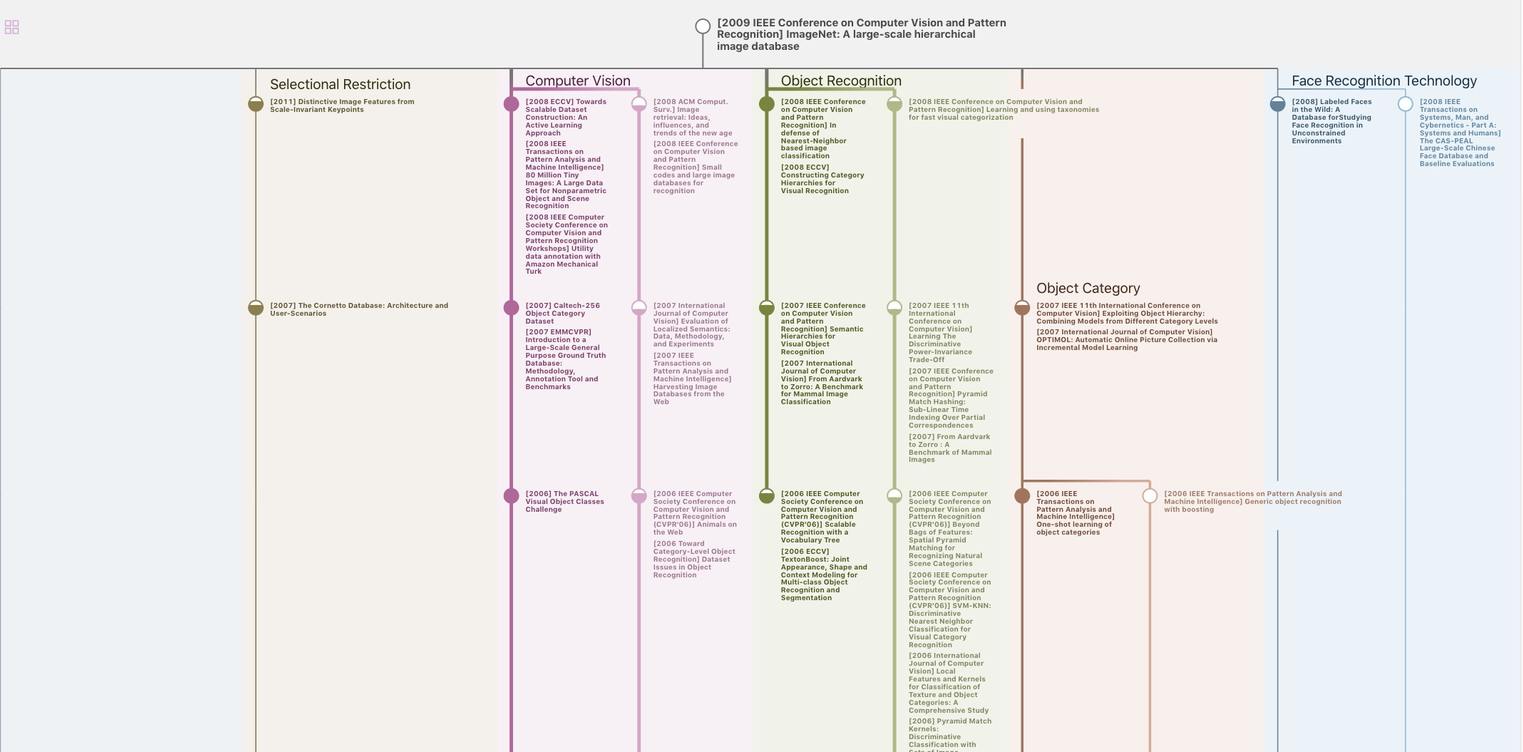
生成溯源树,研究论文发展脉络
Chat Paper
正在生成论文摘要