Significant Ties Graph Neural Networks for Continuous-Time Temporal Networks Modeling
SmartWorld/UIC/ScalCom/DigitalTwin/PriComp/Meta(2022)
摘要
Temporal networks are suitable for modeling complex evolving systems. It has a wide range of applications, such as social network analysis, recommender systems, and epidemiology. Recently, modeling such dynamic systems has drawn great attention in many domains. However, most existing approaches resort to taking discrete snapshots of the temporal networks and modeling all events with equal importance. This paper proposes Significant Ties Graph Neural Networks (STGNN), a novel framework that captures and describes significant ties. To better model the diversity of interactions, STGNN introduces a novel aggregation mechanism to organize the most significant historical neighbors' information and adaptively obtain the significance of node pairs. Experimental results on four real networks demonstrate the effectiveness of the proposed framework.
更多查看译文
关键词
temporal network,graph neural network,significant ties,continuous-time
AI 理解论文
溯源树
样例
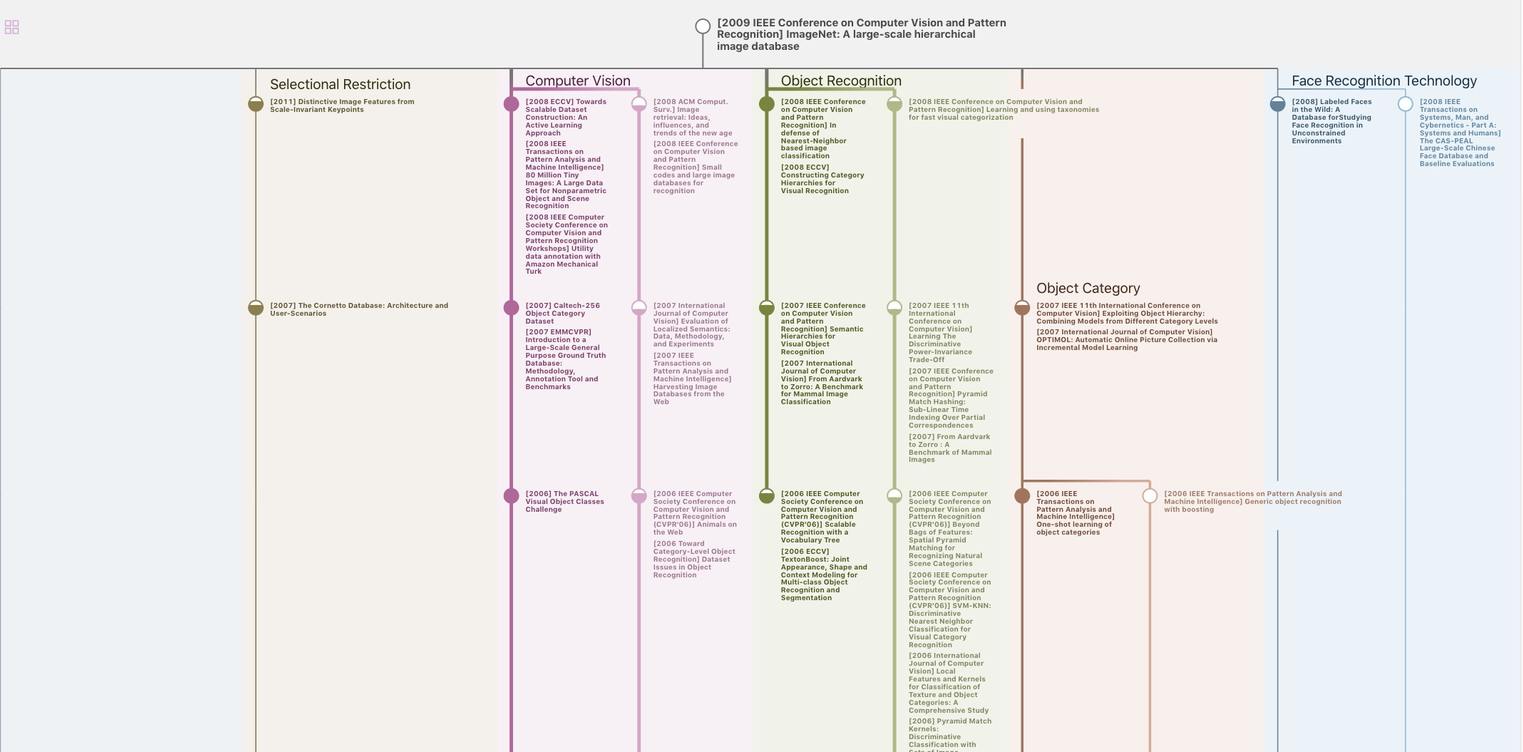
生成溯源树,研究论文发展脉络
Chat Paper
正在生成论文摘要