Distribution-free predictive inference for partial least squares regression with applications to molecular descriptors datasets
Journal of Chemometrics(2022)
Abstract
In statistical modeling, partial least squares (PLS) regression is one of the most popular techniques for prediction problems. An important but often overlooked problem is that the estimation of prediction confidence intervals always contains an unobserved response value with a specified probability. Therefore, in the present work, we studied how to estimate prediction intervals in PLS regression without any distributional assumptions on data. First, a recently proposed method, jackknife+, is introduced to PLS regression for uncertainty quantification. Second, a novel approach is developed to construct distribution-free prediction intervals. Finally, empirical studies on simulations and three real-world datasets show that the proposed method has better coverage properties than other state-of-the-art methods.
MoreTranslated text
Key words
cross-validation,distribution-free,jackknife,PLS,prediction intervals
AI Read Science
Must-Reading Tree
Example
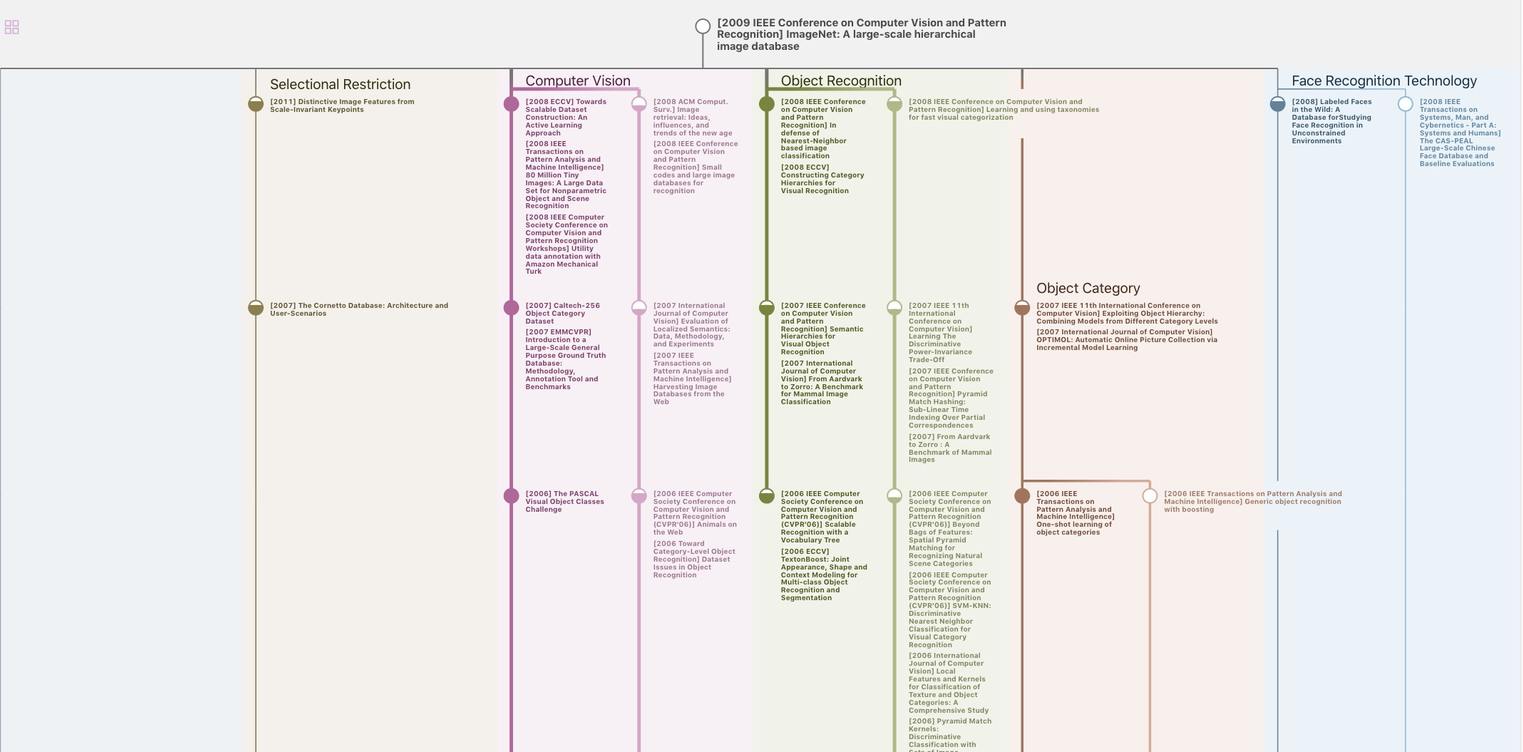
Generate MRT to find the research sequence of this paper
Chat Paper
Summary is being generated by the instructions you defined