Class-Guided Swin Transformer for Semantic Segmentation of Remote Sensing Imagery
IEEE GEOSCIENCE AND REMOTE SENSING LETTERS(2022)
摘要
Semantic segmentation of remote sensing images plays a crucial role in a wide variety of practical applications, including land cover mapping, environmental protection, and economic assessment. In the last decade, convolutional neural network (CNN) is the mainstream deep learning-based method of semantic segmentation. Compared with conventional methods, CNN-based methods learn semantic features automatically, thereby achieving strong representation capability. However, the local receptive field of the convolution operation limits CNN-based methods from capturing long-range dependencies. In contrast, Vision Transformer (ViT) demonstrates its great potential in modeling long-range dependencies and obtains superior results in semantic segmentation. Inspired by this, in this letter, we propose a class-guided Swin Transformer (CG-Swin) for semantic segmentation of remote sensing images. Specifically, we adopt a Transformer-based encoder-decoder structure, which introduces the Swin Transformer backbone as the encoder and designs a class-guided Transformer block to construct the decoder. The experimental results on ISPRS Vaihingen and Potsdam datasets demonstrate the significant breakthrough of the proposed method over ten benchmarks, outperforming both advanced CNN-based and recent Transformer-based approaches.
更多查看译文
关键词
Transformers,Semantics,Remote sensing,Decoding,Feature extraction,Convolution,Residual neural networks,Class-guided mechanism,fully transformer network,remote sensing,semantic segmentation
AI 理解论文
溯源树
样例
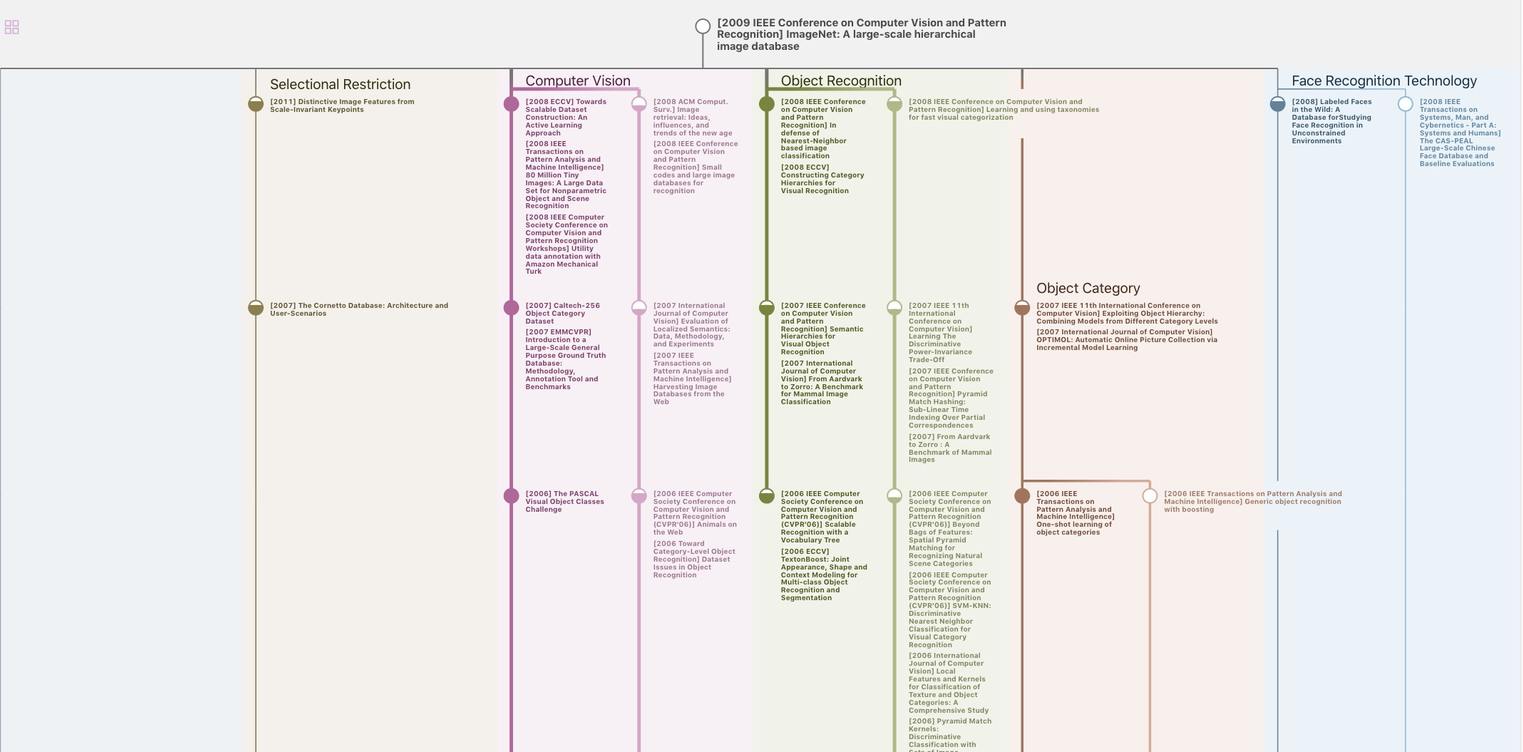
生成溯源树,研究论文发展脉络
Chat Paper
正在生成论文摘要