A FCEEMD Energy Kurtosis Mean Filtering-Based Fault Feature Extraction Method
COATINGS(2022)
Abstract
Aiming at the problem that fault feature extraction is susceptible to background noises and burrs, we proposed a new feature extraction method based on a new decomposition method and an effective intrinsic mode function (IMF) selection method. Firstly, pairs of white noises with opposite signs were introduced to neutralize the residual noises in ensemble empirical mode decomposition (EEMD) and suppress mode mixing. Both the reconstruction error (1.8445 x 10(-17)) and decomposition time (0.01 s) were greatly reduced through fast, complementary ensemble empirical mode decomposition (FCEEMD). Secondly, we integrated the energy and kurtosis of the IMF and proposed an effective IMF selection method based on energy kurtosis mean filtering, and the background noise of the signal was greatly suppressed. Finally, the periodic impacts were extracted from the IMF reconstruction signal by multipoint optimal minimum entropy deconvolution adjusted (MOMEDA). The fault frequencies were extracted from the periodic impacts through Hilbert demodulation, and the relative errors between the measured values and the theoretical values were all less than 0.05. The experimental results show that the proposed method can extract fault features more efficiently and provide a novel method for the fault diagnosis of rotating machinery.
MoreTranslated text
Key words
bearing, fast complementary ensemble empirical mode decomposition, energy kurtosis mean filtering, multipoint optimal minimum entropy deconvolution adjusted, feature extraction
AI Read Science
Must-Reading Tree
Example
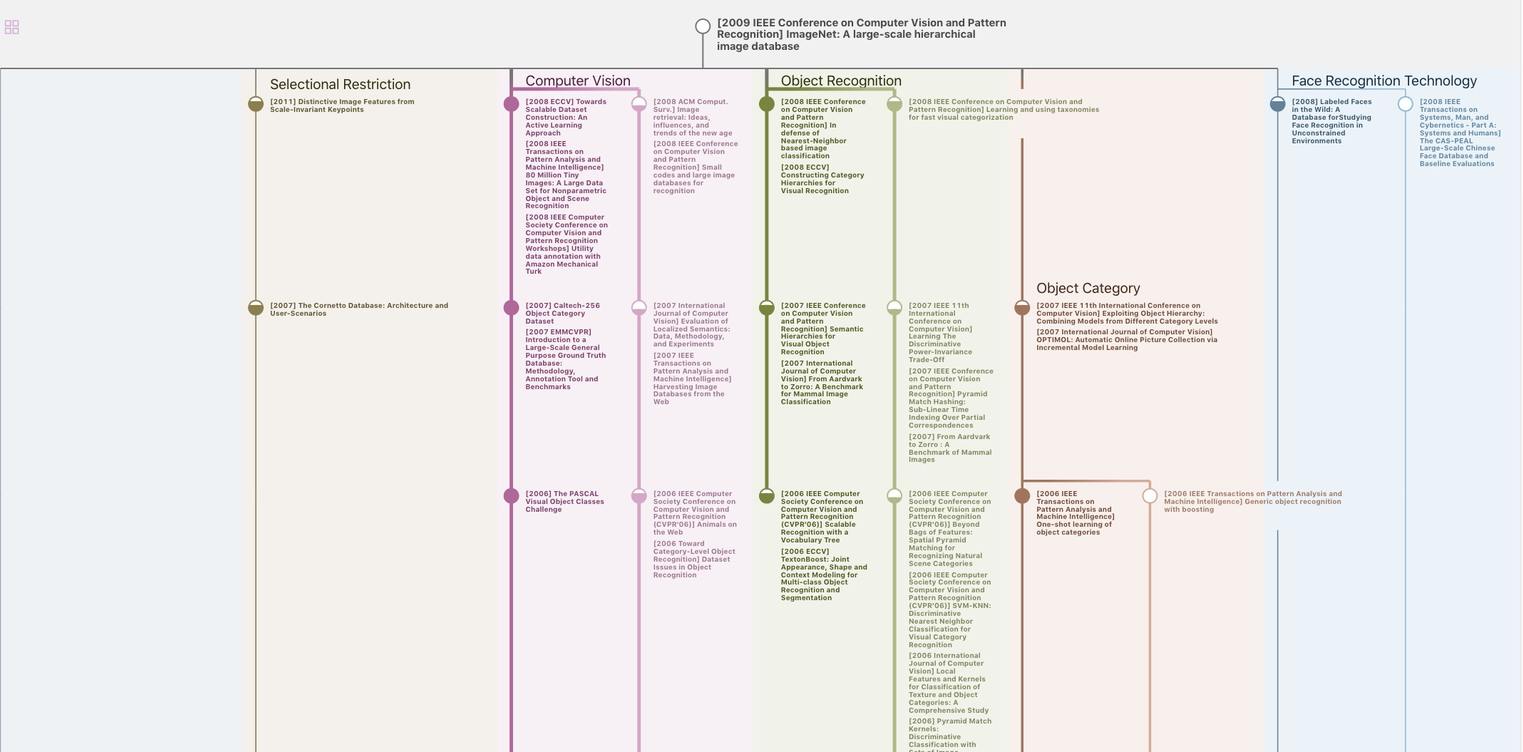
Generate MRT to find the research sequence of this paper
Chat Paper
Summary is being generated by the instructions you defined