Atrial Fibrillation Detection Based on a Residual CNN Using BCG Signals
ELECTRONICS(2022)
摘要
Atrial fibrillation (AF) is the most common arrhythmia and can seriously threaten patient health. Research on AF detection carries important clinical significance. This manuscript proposes an AF detection method based on ballistocardiogram (BCG) signals collected by a noncontact sensor. We first constructed a BCG signal dataset consisting of 28,214 ten-second nonoverlapping segments collected from 45 inpatients during overnight sleep, including 9438 for AF, 9570 for sinus rhythm (SR), and 9206 for motion artifacts (MA). Then, we designed a residual convolutional neural network (CNN) for AF detection. The network has four modules, namely a downsampling convolutional module, a local feature learning module, a global feature learning module, and a classification module, and it extracts local and global features from BCG signals for AF detection. The model achieved precision, sensitivity, specificity, F1 score, and accuracy of 96.8%, 93.7%, 98.4%, 95.2%, and 96.8%, respectively. The results indicate that the AF detection method proposed in this manuscript could serve as a basis for long-term screening of AF at home based on BCG signal acquisition.
更多查看译文
关键词
ballistocardiogram, atrial fibrillation, residual neural network, classification
AI 理解论文
溯源树
样例
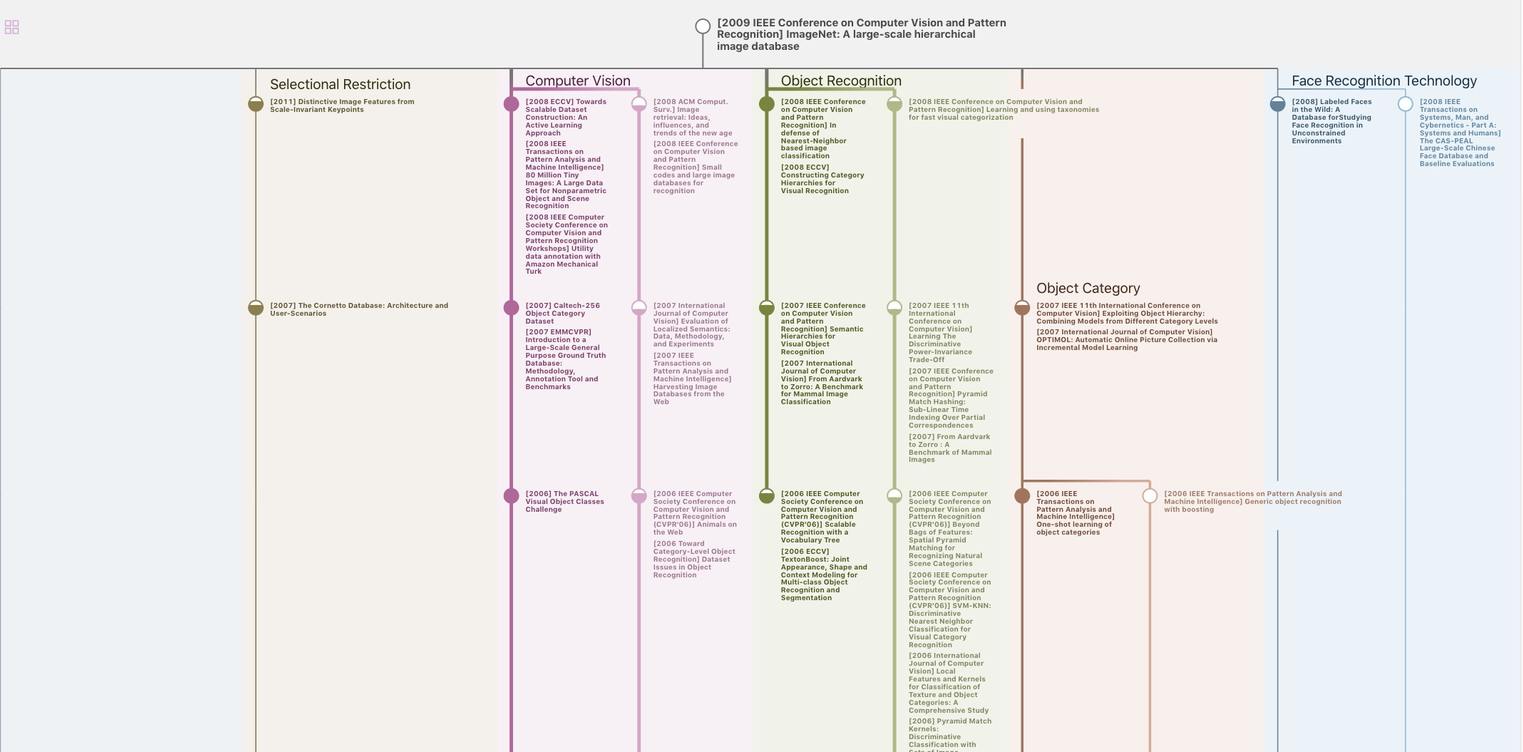
生成溯源树,研究论文发展脉络
Chat Paper
正在生成论文摘要