Federated Naive Bayes under Differential Privacy
SECRYPT : PROCEEDINGS OF THE 19TH INTERNATIONAL CONFERENCE ON SECURITY AND CRYPTOGRAPHY(2022)
Abstract
Growing privacy concerns regarding personal data disclosure are contrasting with the constant need of such information for data-driven applications. To address this issue, the combination of federated learning and differential privacy is now well-established in the domain of machine learning. These techniques allow to train deep neural networks without collecting the data and while preventing information leakage. However, there are many scenarios where simpler and more robust machine learning models are preferable. In this paper, we present a federated and differentially-private version of the Naive Bayes algorithm for classification. Our results show that, without data collection, the same performance of a centralized solution can be achieved on any dataset with only a slight increase in the privacy budget. Furthermore, if certain conditions are met, our federated solution can outperform a centralized approach.
MoreTranslated text
Key words
Federated Learning,Naive Bayes,Differential Privacy
AI Read Science
Must-Reading Tree
Example
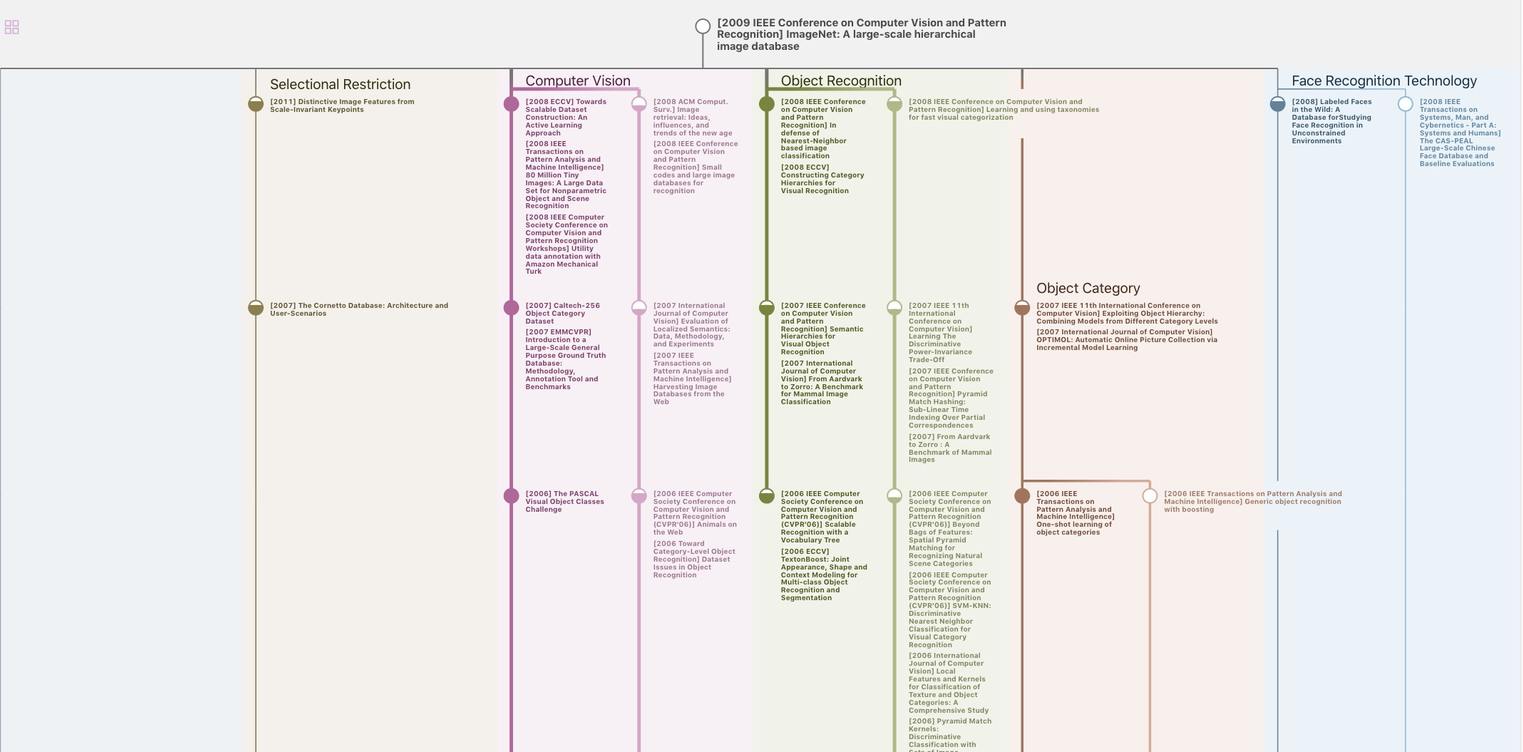
Generate MRT to find the research sequence of this paper
Chat Paper
Summary is being generated by the instructions you defined