On the Angular Update and Hyperparameter Tuning of a Scale-Invariant Network.
European Conference on Computer Vision(2022)
摘要
Modern deep neural networks are equipped with normalization layers such as batch normalization or layer normalization to enhance and stabilize training dynamics. If a network contains such normalization layers, the optimization objective is invariant to the scale of the neural network parameters. The scale-invariance induces the neural network's output to be only affected by the weights' direction and not the weights' scale. We first find a common feature of good hyperparameter combinations on such a scale-invariant network, including learning rate, weight decay, number of data samples, and batch size. Then we observe that hyperparameter setups that lead to good performance show similar degrees of angular update during one epoch. Using a stochastic differential equation, we analyze the angular update and show how each hyperparameter affects it. With this relationship, we can derive a simple hyperparameter tuning method and apply it to the efficient hyperparameter search.
更多查看译文
关键词
Scale-invariant network,normalization,effective learning rate,angular update,hyperparameter tuning
AI 理解论文
溯源树
样例
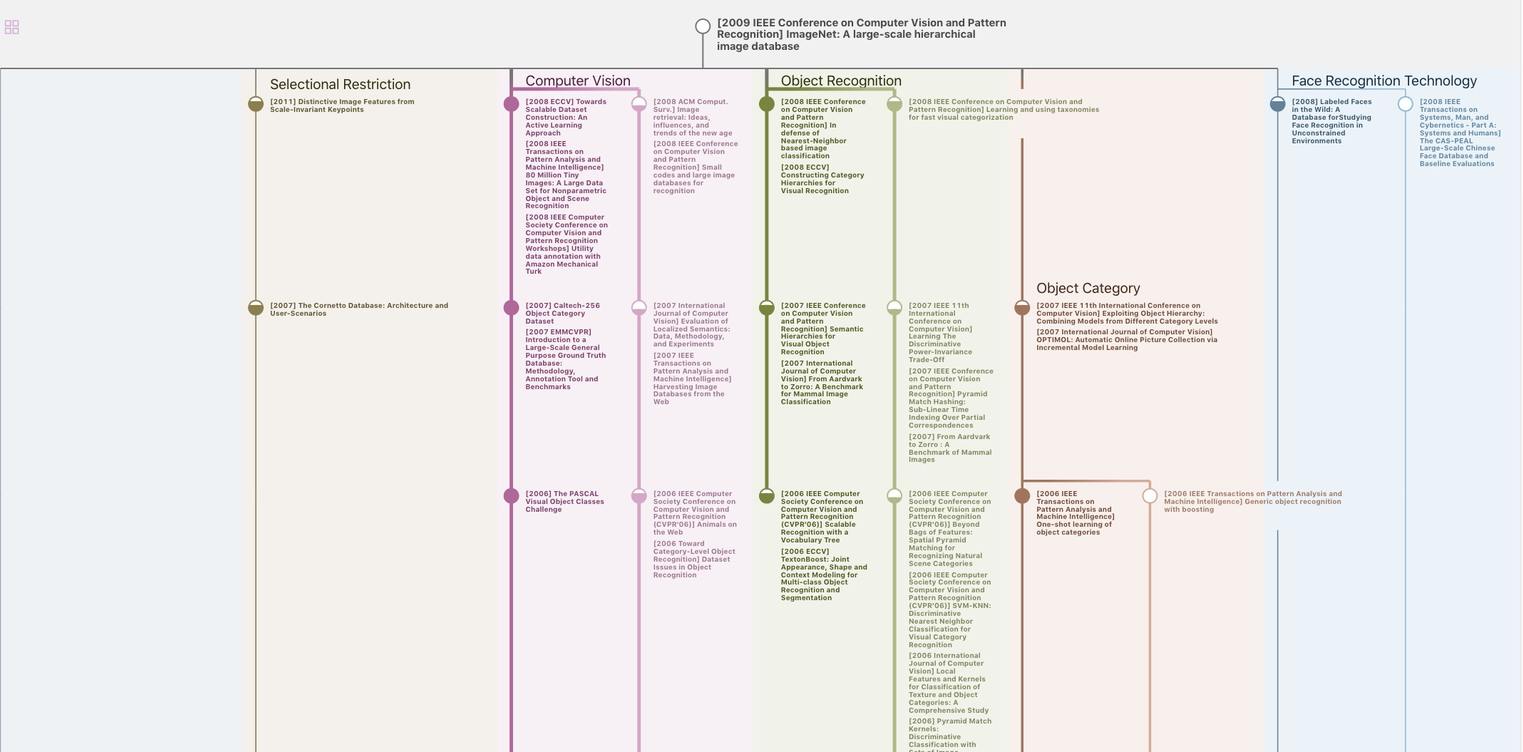
生成溯源树,研究论文发展脉络
Chat Paper
正在生成论文摘要