Conditionally Factorized Variational Bayes with Importance Sampling
ICASSP 2022 - 2022 IEEE International Conference on Acoustics, Speech and Signal Processing (ICASSP)(2022)
摘要
Coordinate ascent variational inference (CAVI) is a popular approximate inference method; however, it relies on a mean-field assumption that can lead to large estimation errors for highly correlated variables. In this paper, we propose a conditionally factorized variational family with an adjustable conditional structure and derive the corresponding coordinate ascent algorithm for optimization. The algorithm is termed Conditionally factorized Variational Bayes (CVB) and implemented with importance sampling. We show that by choosing a finer conditional structure, our algorithm can be guaranteed to achieve a better variational lower bound, thus providing a flexible trade-off between computational cost and inference accuracy. The validity of the method is demonstrated in a simple posterior computation task.
更多查看译文
关键词
variational Bayes,importance sampling,coordinate ascent variational inference,structured variational inference
AI 理解论文
溯源树
样例
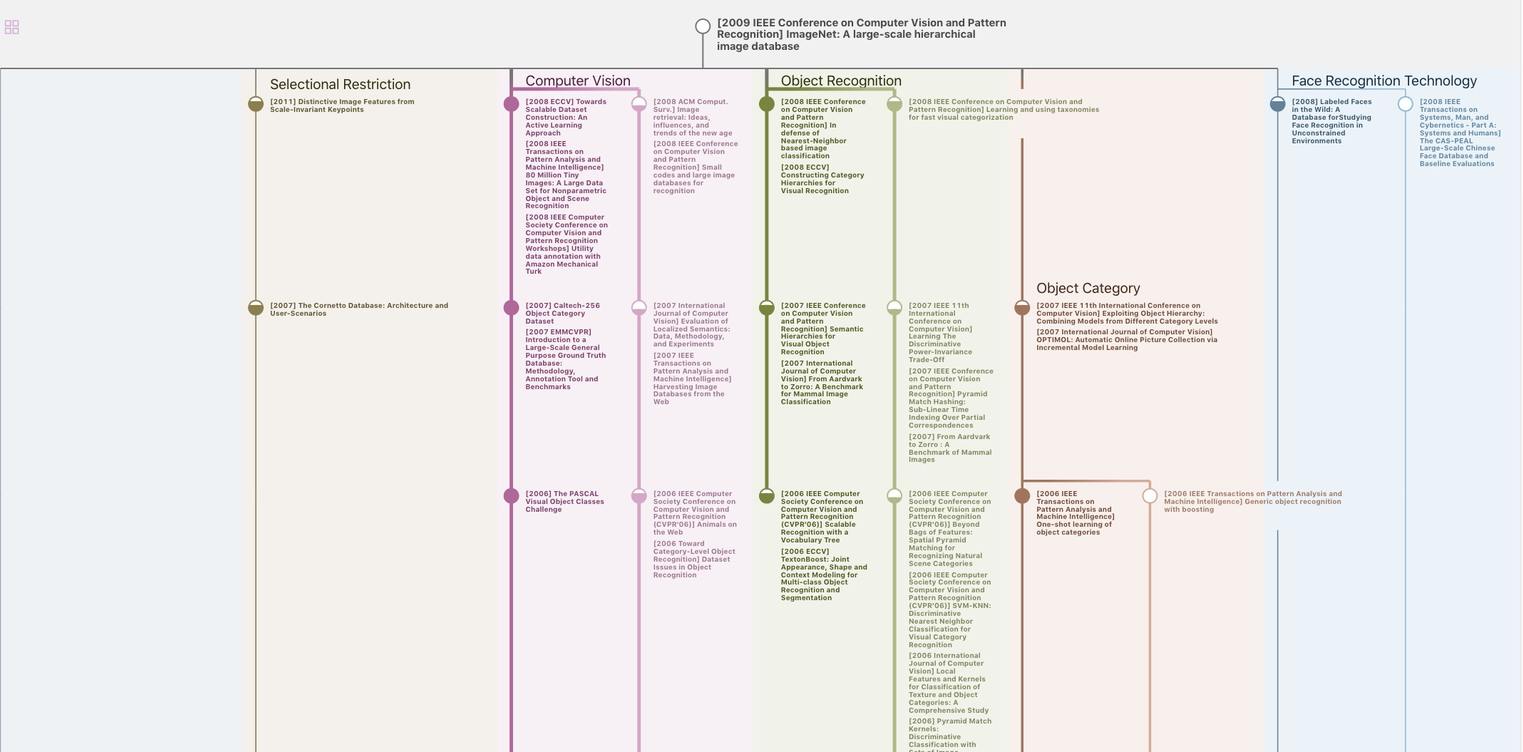
生成溯源树,研究论文发展脉络
Chat Paper
正在生成论文摘要