PG-VulNet: Detect Supply Chain Vulnerabilities in IoT Devices using Pseudo-code and Graphs
PROCEEDINGS OF THE16TH ACM/IEEE INTERNATIONAL SYMPOSIUM ON EMPIRICAL SOFTWARE ENGINEERING AND MEASUREMENT, ESEM 2022(2022)
摘要
Background: With the boosting development of IoT technology, the supply chains of IoT devices become more powerful and sophisticated, and the security issues introduced by code reuse are becoming more prominent. Therefore, the detection and management of vulnerabilities through code similarity detection technology is of great significance for protecting the security of IoT devices. Aim: We aim to propose a more accurate, parallel-friendly, and realistic software supply chain vulnerability detection solution for IoT devices. Method: This paper presents PG-VulNet, standing for Vulnerability-detection Network based on Pseudo-code Graphs. It is a "multi-model" cross-architecture vulnerability detection solution based on pseudo-code and Graph Matching Network (GMN). PG-VulNet extracts both behavioral and structural features of pseudo-code to build customized feature graphs and then uses GMN to detect supply chain vulnerabilities based on these graphs. Results: The experiments show that PG-VulNet achieves an average detection accuracy of 99.14%, significantly higher than existing approaches like Gemini, VulSeeker, FIT, and Asteria. In addition to this, PG-VulNet also excels in detection overhead and false alarms. In the real-world evaluation, PG-VulNet detected 690 known vulnerabilities in 1,611 firmwares. Conclusions: PG-VulNet can effectively detect the vulnerabilities introduced by software supply chain in IoT firmwares and is well suited for large-scale detection. Compared with existing approaches, PG-VulNet has significant advantages.
更多查看译文
关键词
Binary Code Similarity,IoT Software Supply Chain,Vulnerability Detection,Graph Neural Network
AI 理解论文
溯源树
样例
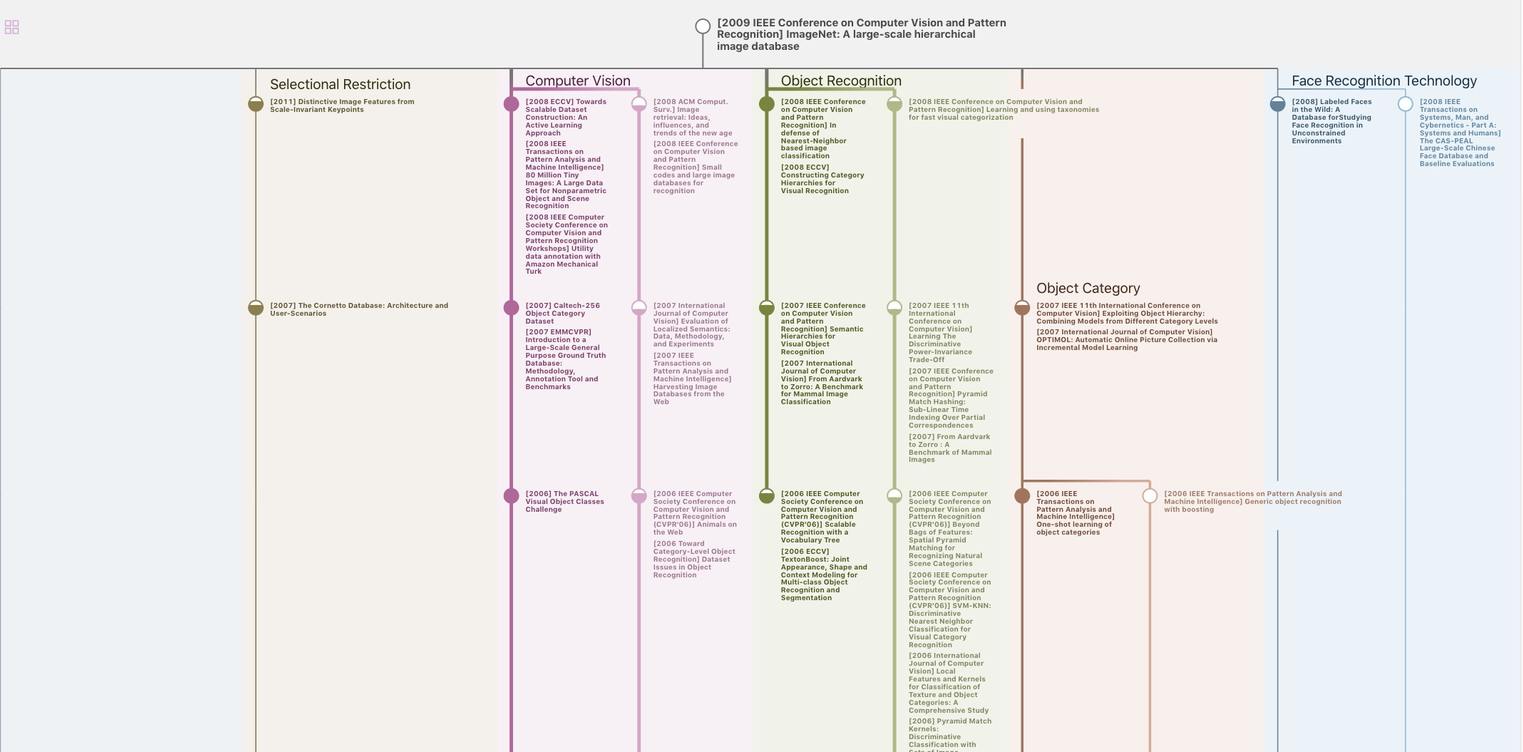
生成溯源树,研究论文发展脉络
Chat Paper
正在生成论文摘要