Flexible Chip Placement Via Reinforcement Learning: Late Breaking Results
Proceedings of the 59th ACM/IEEE Design Automation Conference(2022)
Abstract
Recently, successful applications of reinforcement learning to chip placement have emerged. Pretrained models are necessary to improve efficiency and effectiveness. Currently, the weights of objective metrics (e.g., wirelength, congestion, and timing) are fixed during pretraining. However, fixed-weighed models cannot generate the diversity of placements required for engineers to accommodate changing requirements as they arise. This paper proposes flexible multiple-objective reinforcement learning (MORL) to support objective functions with inference-time variable weights using just a single pretrained model. Our macro placement results show that MORL can generate the Pareto frontier of multiple objectives effectively.
MoreTranslated text
AI Read Science
Must-Reading Tree
Example
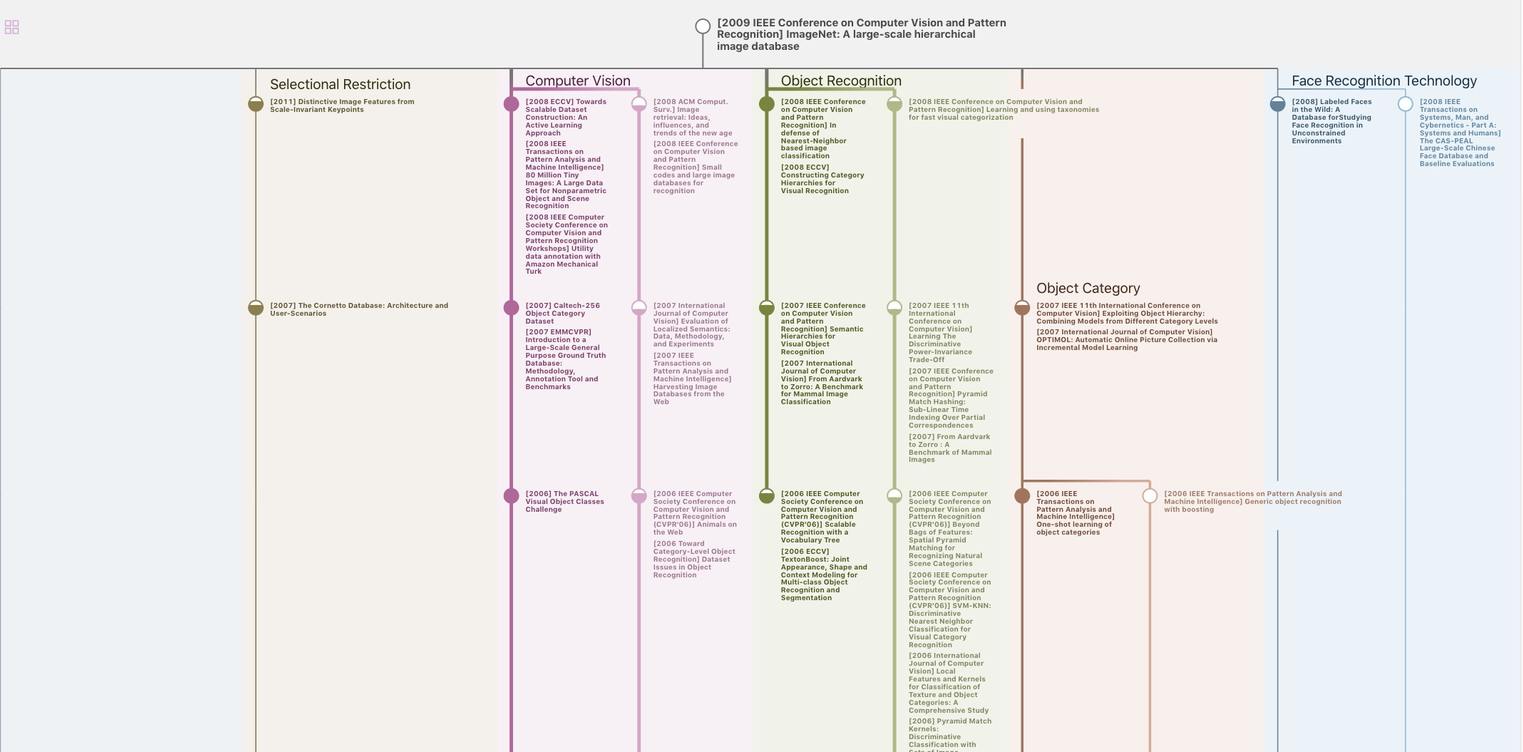
Generate MRT to find the research sequence of this paper
Chat Paper
Summary is being generated by the instructions you defined