Graph Neural Network based Root Cause Analysis Using Multivariate Time-series KPIs for Wireless Networks
NOMS 2022-2022 IEEE/IFIP Network Operations and Management Symposium(2022)
摘要
Due to the rapid adoption of 5G networks and the increasing number of devices and base stations (gNBs) connected to it, manually identifying malfunctioning machines or devices that cause a part of the networks to fail becomes more challenging. Furthermore, data collected from the networks are not always sufficient. To overcome these two issues, we proposed a novel root cause analysis (RCA) framework that integrates graph neural networks (GNNs) with graph structure learning (GSL) to infer hidden dependencies from available data. The learned dependencies are the graph structure utilized to predict the root cause machines or devices. We found that despite the fact that the data is often incomplete, the GSL model can infer fairly accurate hidden dependencies from data with a large number of nodes and generate informative graph representation for GNNs to identify the root cause. Our experimental results showed that higher accuracy of identifying a root cause and victim nodes can be achieved when the number of nodes in an environment is increased.
更多查看译文
关键词
5G networks,anomaly detection,fault localization,root cause analysis,graph neural networks
AI 理解论文
溯源树
样例
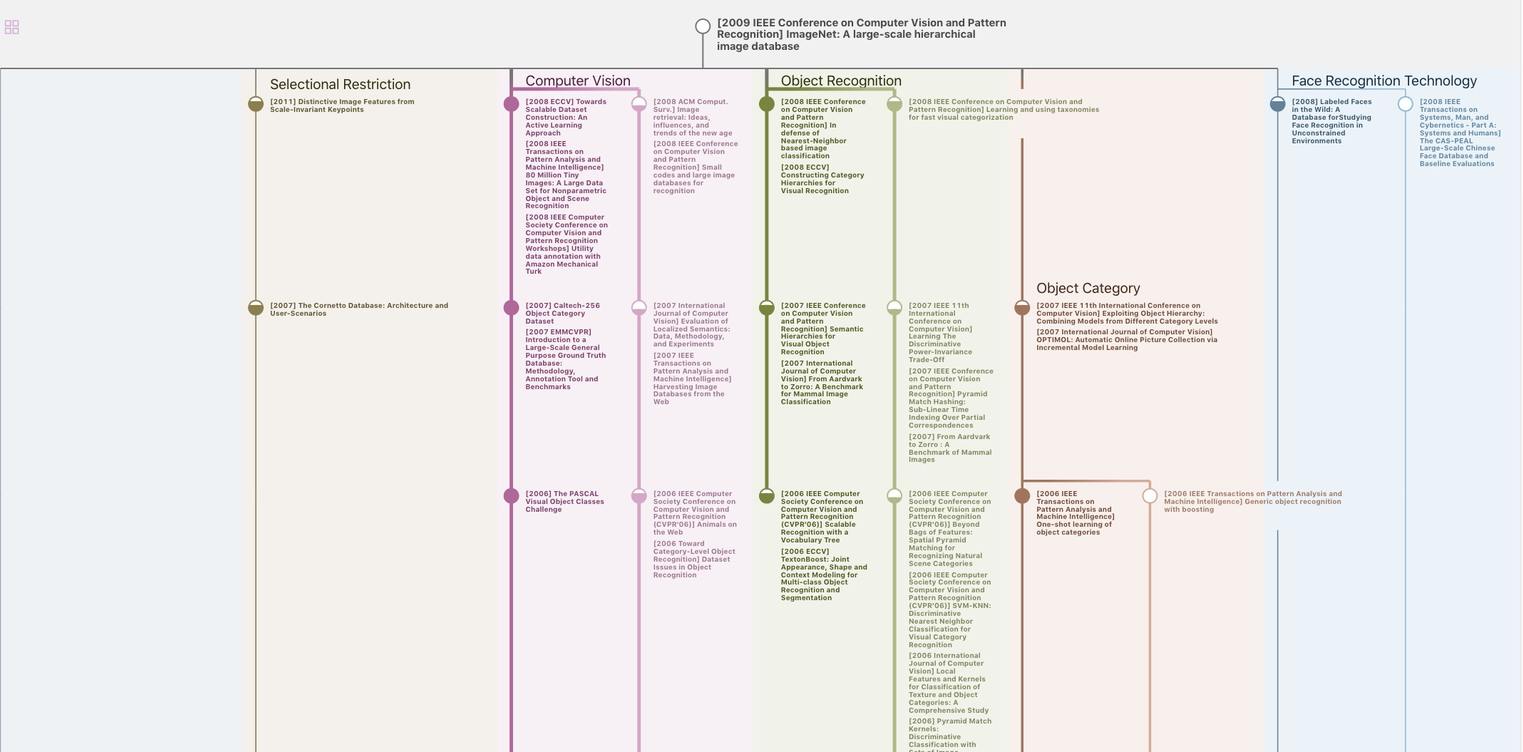
生成溯源树,研究论文发展脉络
Chat Paper
正在生成论文摘要