Humans parsimoniously represent auditory sequences by pruning and completing the underlying network structure
ELIFE(2023)
摘要
Successive auditory inputs are rarely independent, their relationships ranging from local transitions between elements to hierarchical and nested representations. In many situations, humans retrieve these dependencies even from limited datasets. However, this learning at multiple scale levels is poorly understood. Here, we used the formalism proposed by network science to study the representation of local and higher-order structures and their interaction in auditory sequences. We show that human adults exhibited biases in their perception of local transitions between elements, which made them sensitive to high-order network structures such as communities. This behavior is consistent with the creation of a parsimonious simplified model from the evidence they receive, achieved by pruning and completing relationships between network elements. This observation suggests that the brain does not rely on exact memories but on a parsimonious representation of the world. Moreover, this bias can be analytically modeled by a memory/efficiency trade-off. This model correctly accounts for previous findings, including local transition probabilities as well as high-order network structures, unifying sequence learning across scales. We finally propose putative brain implementations of such bias. Editor's evaluation This paper communicates important findings on the learning of local and higher order structures in auditory sequences and will be of interest to researchers studying statistical learning, learning of graph structures, and auditory learning. The strength of the evidence is convincing, including a compelling demonstration that humans do not encode objective transition probabilities and the implementation of a wide range of sequence learning models that have been proposed in the literature.
更多查看译文
关键词
network science,graph learning,statistical learning,human cognition,community structure,Human
AI 理解论文
溯源树
样例
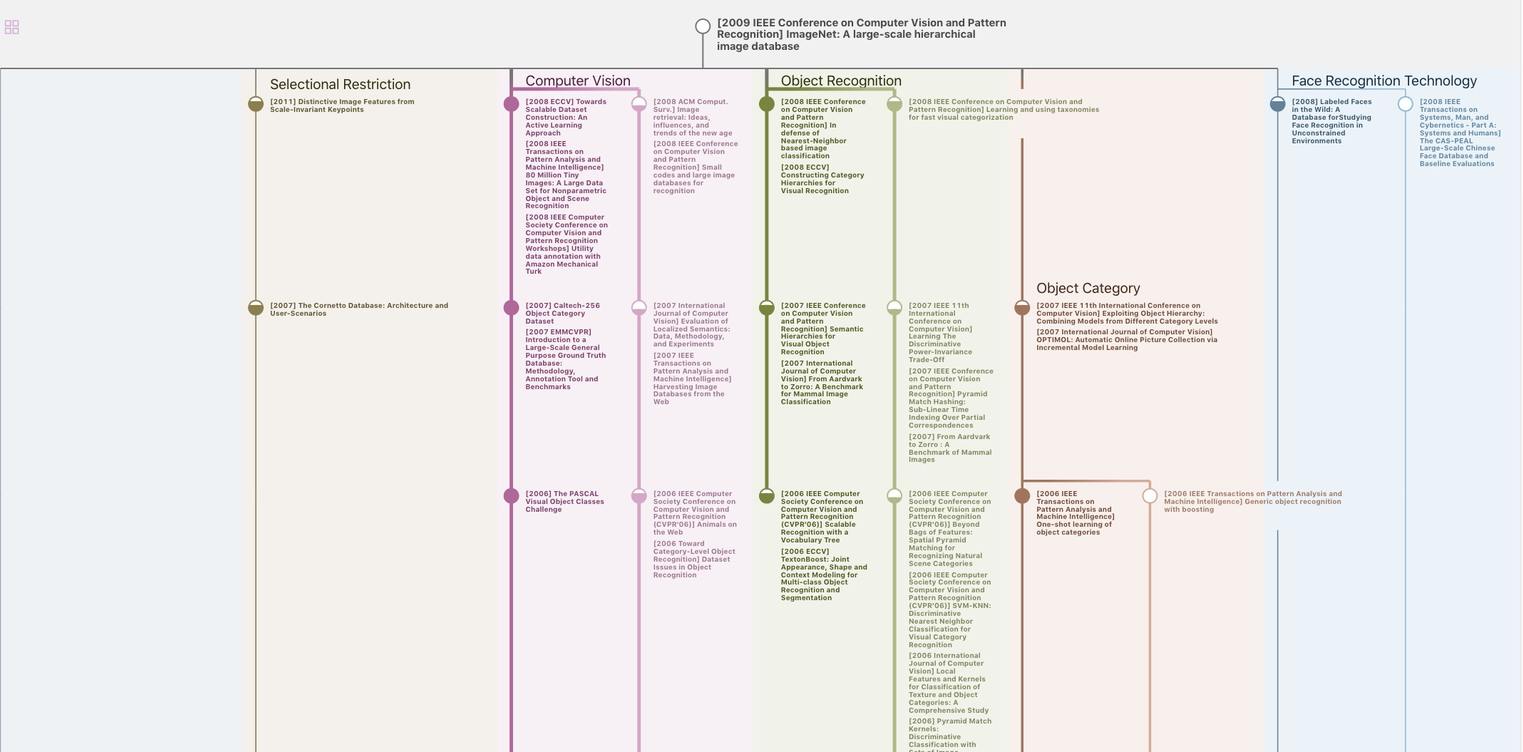
生成溯源树,研究论文发展脉络
Chat Paper
正在生成论文摘要