Detection of Heart Disease Employing Recurrent CONVoluted Neural Networks (Rec-Convnet) for Effectual Classification Process in Smart Medical Application
2021 4th International Conference on Recent Trends in Computer Science and Technology (ICRTCST)(2022)
摘要
Deep learning has implementations in several disciplines like manufacturing, medical service, farming, and so on. Lately, wearable devices have turned famous with extensive implementations in health monitory system that has accelerated the development of Internet of Medical Things (IoMT). IoMT possesses a significant function to perform in lessening the fatality rate by initial diagnosis of illness. The prediction of heart illness remains the main problem in assessing the medical dataset. The proposed study's goal is to identify key characteristics of heart disease prediction using machine learning techniques. Several research works have concentrated on heart illness detection, yet the precision of the outcome is poor. Hence, for enhancing the prediction precision, an IoMT architecture employing Recurrent CONVoluted neural networks (Rec-CONVnet) is proffered for the detection of heart illness. Rec-CONVnet's learning technique relies on gradient-based learning and is prone to becoming stuck in local minima. Age, sex, chest discomfort, blood sugar, Blood pressure (BP), and other factors are acquired from clinical records to estimate the risk of heart disease. Heart state is recognized by classifying acquired sensor data employing Rec-CONVnet. Simulation and assessment are carried out to exhibit that Rec-CONVnet performs marvelously concerning illness prediction. Outcomes of the simulation indicate that Rec-CONVnet prediction paradigm attains finer precision than the rest of the techniques. The proffered Rec-CONVnet attains an F1-score of 97%, FPR of 64.6%, accuracy rate of 96.4%, and precision 76.2%.
更多查看译文
关键词
Deep neural network,feature selection,classification,heart illness,deep learning
AI 理解论文
溯源树
样例
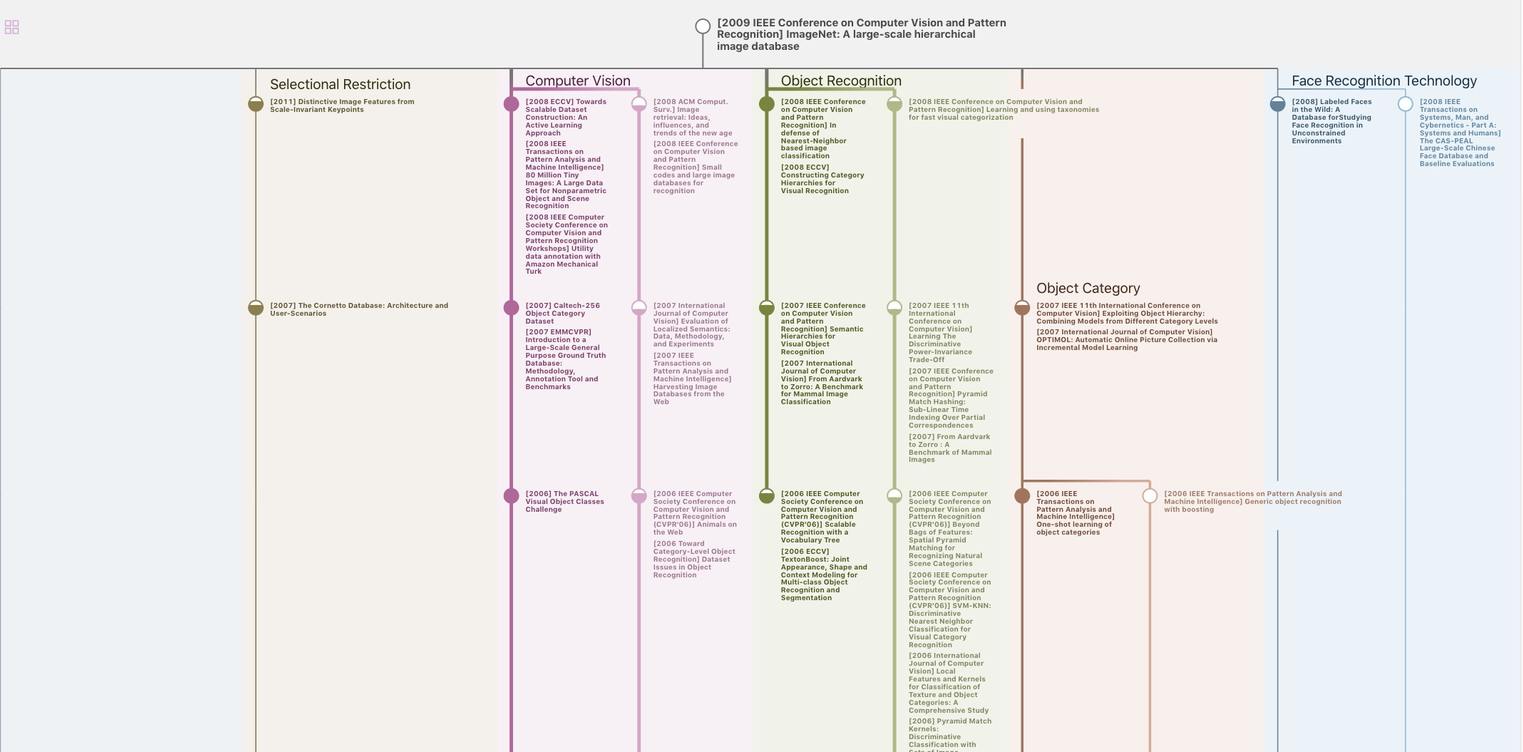
生成溯源树,研究论文发展脉络
Chat Paper
正在生成论文摘要