Generalized Fiducial Inference on Differentiable Manifolds
arXiv (Cornell University)(2022)
摘要
We introduce a novel approach to inference on parameters that take values in a Riemannian manifold embedded in a Euclidean space. Parameter spaces of this form are ubiquitous across many fields, including chemistry, physics, computer graphics, and geology. This new approach uses generalized fiducial inference to obtain a posterior-like distribution on the manifold, without needing to know a parameterization that maps the constrained space to an unconstrained Euclidean space. The proposed methodology, called the constrained generalized fiducial distribution (CGFD), is obtained by using mathematical tools from Riemannian geometry. A Bernstein-von Mises-type result for the CGFD, which provides intuition for how the desirable asymptotic qualities of the unconstrained generalized fiducial distribution are inherited by the CGFD, is provided. To demonstrate the practical use of the CGFD, we provide three proof-of-concept examples: inference for data from a multivariate normal density with the mean parameters on a sphere, a linear logspline density estimation problem, and a reimagined approach to the AR(1) model, all of which exhibit desirable coverages via simulation. We discuss two Markov chain Monte Carlo algorithms for the exploration of these constrained parameter spaces and adapt them for the CGFD.
更多查看译文
关键词
generalized fiducial inference,differentiable manifolds
AI 理解论文
溯源树
样例
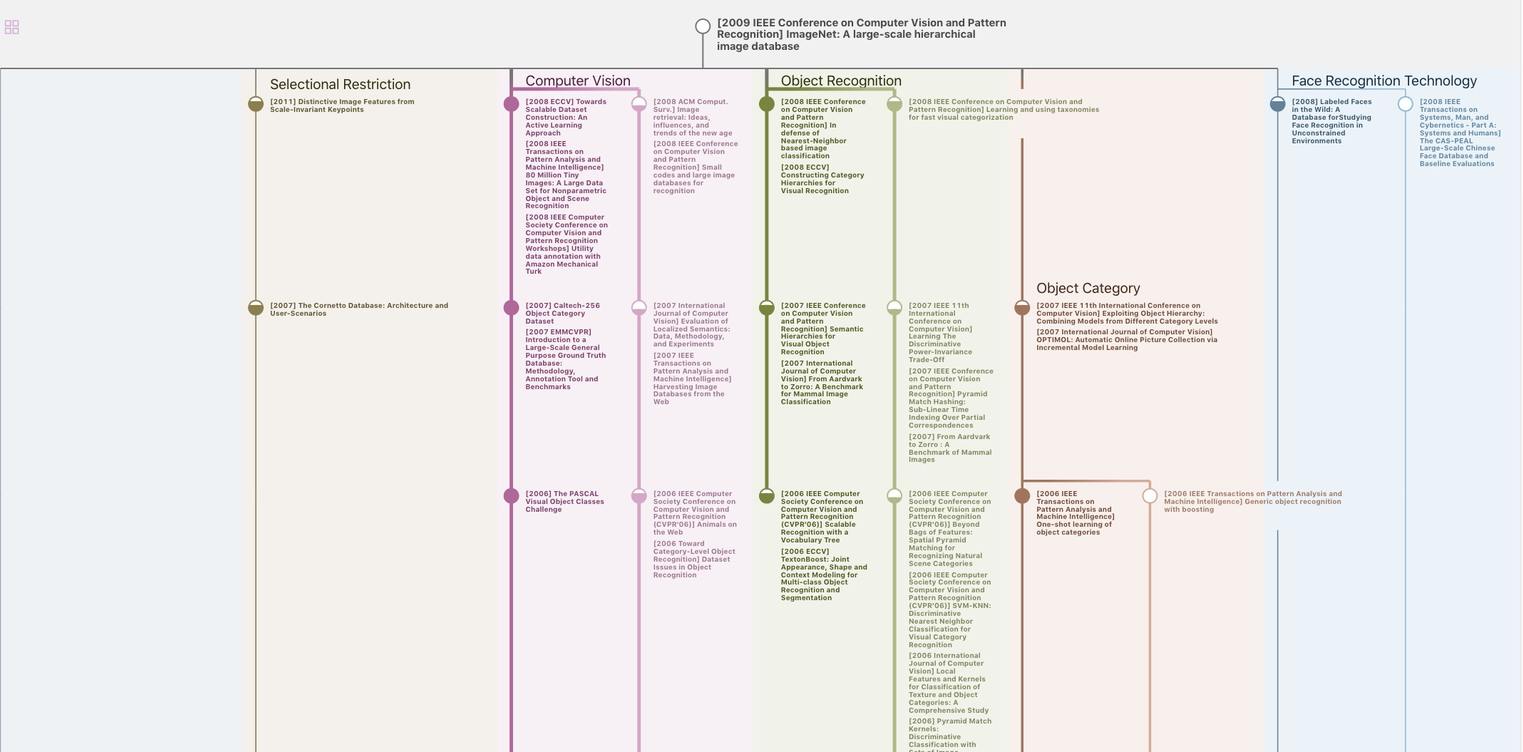
生成溯源树,研究论文发展脉络
Chat Paper
正在生成论文摘要