Deep Reinforcement Learning Applied to Computation Offloading of Vehicular Applications: A Comparison
2022 International Balkan Conference on Communications and Networking (BalkanCom)(2022)
摘要
An observable trend in recent years is the increasing demand for more complex services designed to be used with portable or automotive embedded devices. The problem is that these devices may lack the computational resources necessary to comply with service requirements. To solve it, cloud and edge computing, and in particular, the recent multi-access edge computing (MEC) paradigm, have been proposed. By offloading the processing of computational tasks from devices or vehicles to an external network, a larger amount of computational resources, placed in different locations, becomes accessible. However, this in turn creates the issue of deciding where each task should be executed. In this paper, we model the problem of computation offloading of vehicular applications to solve it using deep reinforcement learning (DRL) and evaluate the performance of different DRL algorithms and heuristics, showing the advantages of the former methods. Moreover, the impact of two scheduling techniques in computing nodes and two reward strategies in the DRL methods are also analyzed and discussed.
更多查看译文
关键词
Deep Reinforcement Learning,Vehicular Applications,Computation Offloading,Edge Computing
AI 理解论文
溯源树
样例
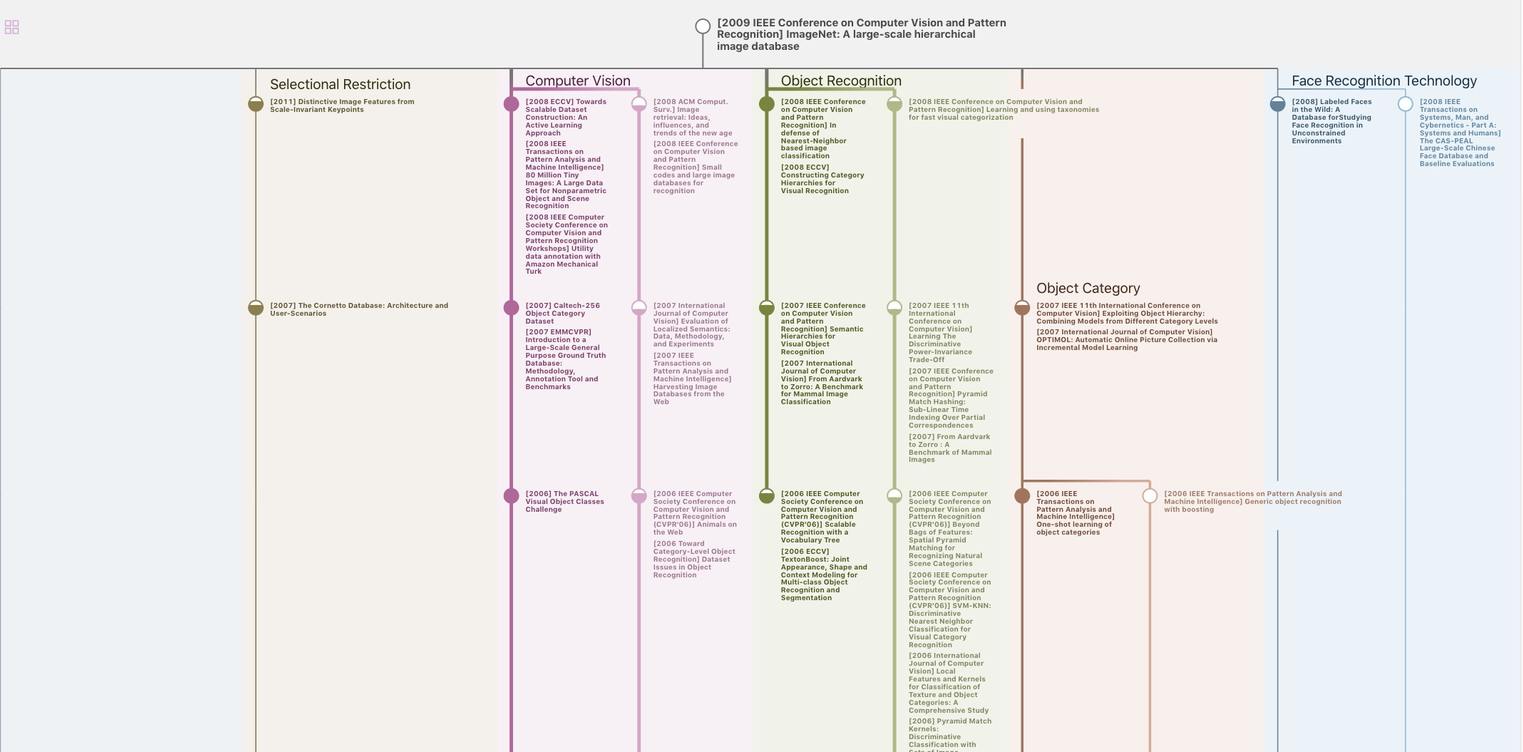
生成溯源树,研究论文发展脉络
Chat Paper
正在生成论文摘要