Expert-level Detection of Pathologies from Unannotated Chest X-ray Images Via Self-Supervised Learning.
Nature biomedical engineering(2022)
Abstract
In tasks involving the interpretation of medical images, suitably trained machine-learning models often exceed the performance of medical experts. Yet such a high-level of performance typically requires that the models be trained with relevant datasets that have been painstakingly annotated by experts. Here we show that a self-supervised model trained on chest X-ray images that lack explicit annotations performs pathology-classification tasks with accuracies comparable to those of radiologists. On an external validation dataset of chest X-rays, the self-supervised model outperformed a fully supervised model in the detection of three pathologies (out of eight), and the performance generalized to pathologies that were not explicitly annotated for model training, to multiple image-interpretation tasks and to datasets from multiple institutions.
MoreTranslated text
Key words
Health care,Medical imaging,Biomedicine,general,Biomedical Engineering/Biotechnology
AI Read Science
Must-Reading Tree
Example
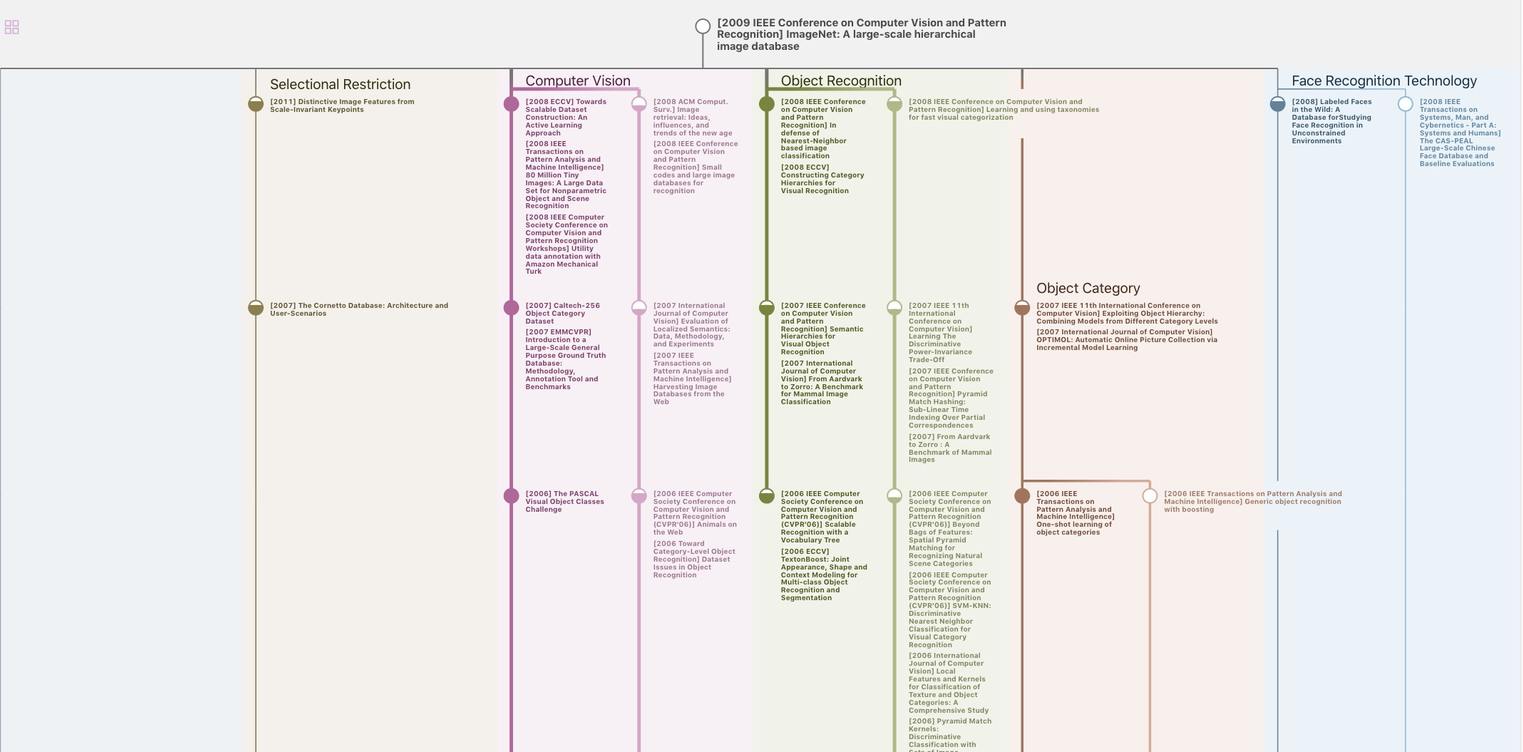
Generate MRT to find the research sequence of this paper
Chat Paper
Summary is being generated by the instructions you defined