Towards High-Quality Thermal Infrared Image Colorization Via Attention-Based Hierarchical Network
NEUROCOMPUTING(2022)
摘要
Colorization is an effective technology to improve the imaging quality of thermal infrared sensors, which is of great importance to environmental perception systems. Recently, colorization for thermal infrared images has realized obvious improvement with the development of deep learning. However, the performance of current designs is not adequate to achieve satisfied colorization quality. This is mainly because that thermal infrared image colorization requires the abilities of both color prediction and grayscale detail recovery, which poses severe challenges for feature extraction due to the poor quality of thermal infrared images. Furthermore, most of the current networks are based on generative adversarial network, suffering the risks of training instability and mode collapse. In this paper, we propose a high-quality colorization network for thermal infrared images. By leveraging hierarchical architecture, attention mechanism, and proposing a composite loss function, our network not only significantly improves the quality of colorized thermal infrared images but also achieves a speed of 32 fps on NVIDIA 2080Ti GPU platform. In evaluation experiments, PSNR and SSIM can be increased by at least 0.87 dB and 0.0277 compared with previous methods on publicly available datasets. Experimental results demonstrate that the proposed network can produce state-of-the-art colorized thermal infrared images both in objective metrics and subjective quality.(c) 2022 Elsevier B.V. All rights reserved.
更多查看译文
关键词
Thermal infrared image,Colorization,Deep learning,Hierarchical architecture,Attention mechanism
AI 理解论文
溯源树
样例
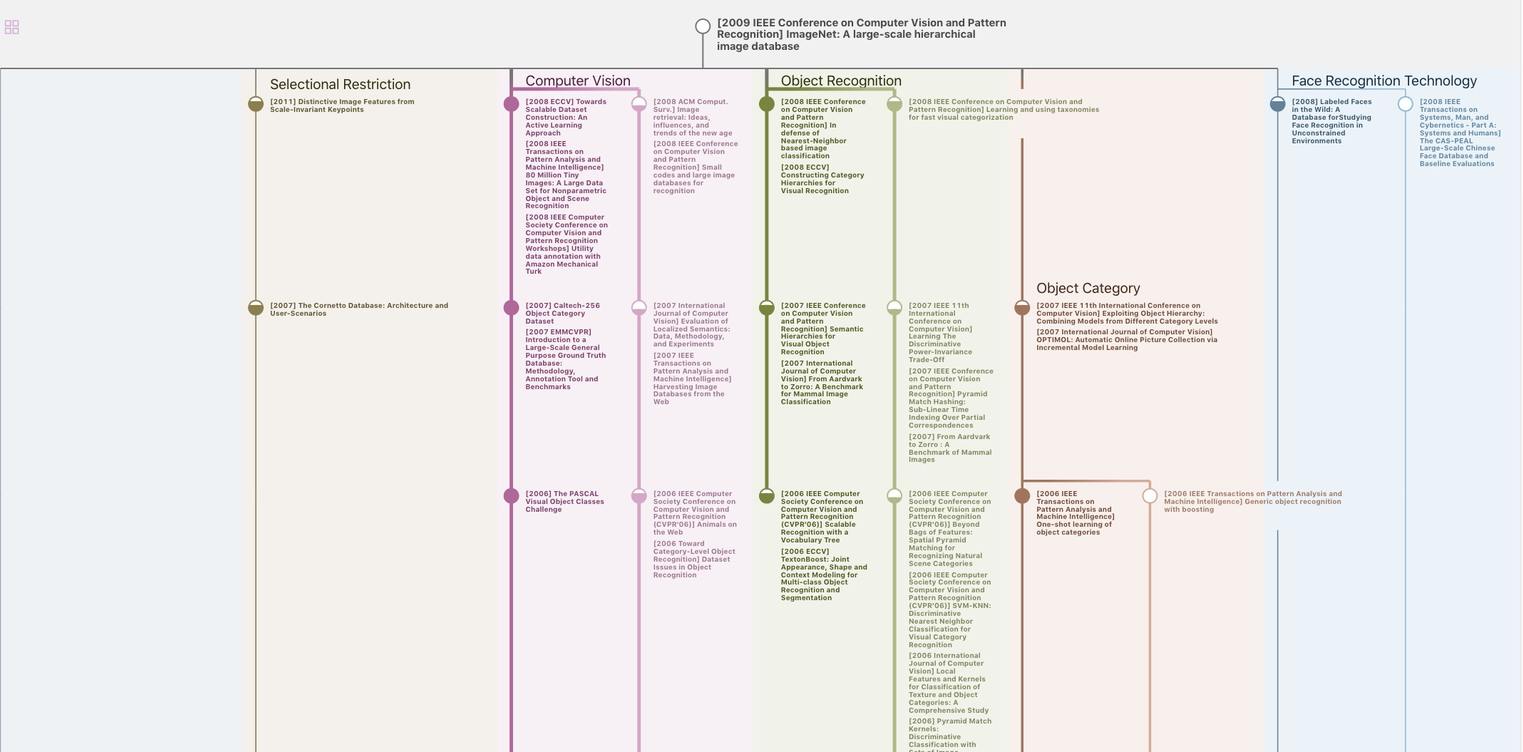
生成溯源树,研究论文发展脉络
Chat Paper
正在生成论文摘要