A Fault Diagnosis Method for Complex Chemical Process Based on Multi-Model Fusion
Process safety and environmental protection/Transactions of the Institution of Chemical Engineers Part B, Process safety and environmental protection/Chemical engineering research and design/Chemical engineering research & design(2022)
摘要
Deep learning methods have become the mainstream research direction in the field of chemical process fault detection and diagnosis, which have great application and research value. However, the existing deep fault diagnosis methods are faced with challenges such as missing data, high-dimensional redundancy and difficulty in fault feature mining, which limits their application in industry. Based on this, a fault diagnosis method for complex chemical process based on multi-model fusion is proposed. This approach avoids over-fitting the model due to excessive redundant data by introducing a FunkSVD matrix decomposition model to augment the missing data without changing the data distribution and then inputting an extreme gradient boosting tree model to learn key features. Finally, the model memory and generalization capability are improved by training a very deep factor decomposer diagnostic model to extract and fuse linear, low-order interaction and high-order interaction features in an all-round way to adaptively establish the correlation between fault features and fault conditions. To validate the model effectiveness, extensive experiments were conducted on the Tennessee Eastman Process dataset and Fluidized Catalytic Cracker fractionation unit dataset, and the results showed that the proposed method has significant performance advantages over existing diagnostic methods in terms of precision and recall metrics.
更多查看译文
关键词
Deep learning,Chemical process,Fault detection and diagnosis,Multi -model fusion,Feature extraction
AI 理解论文
溯源树
样例
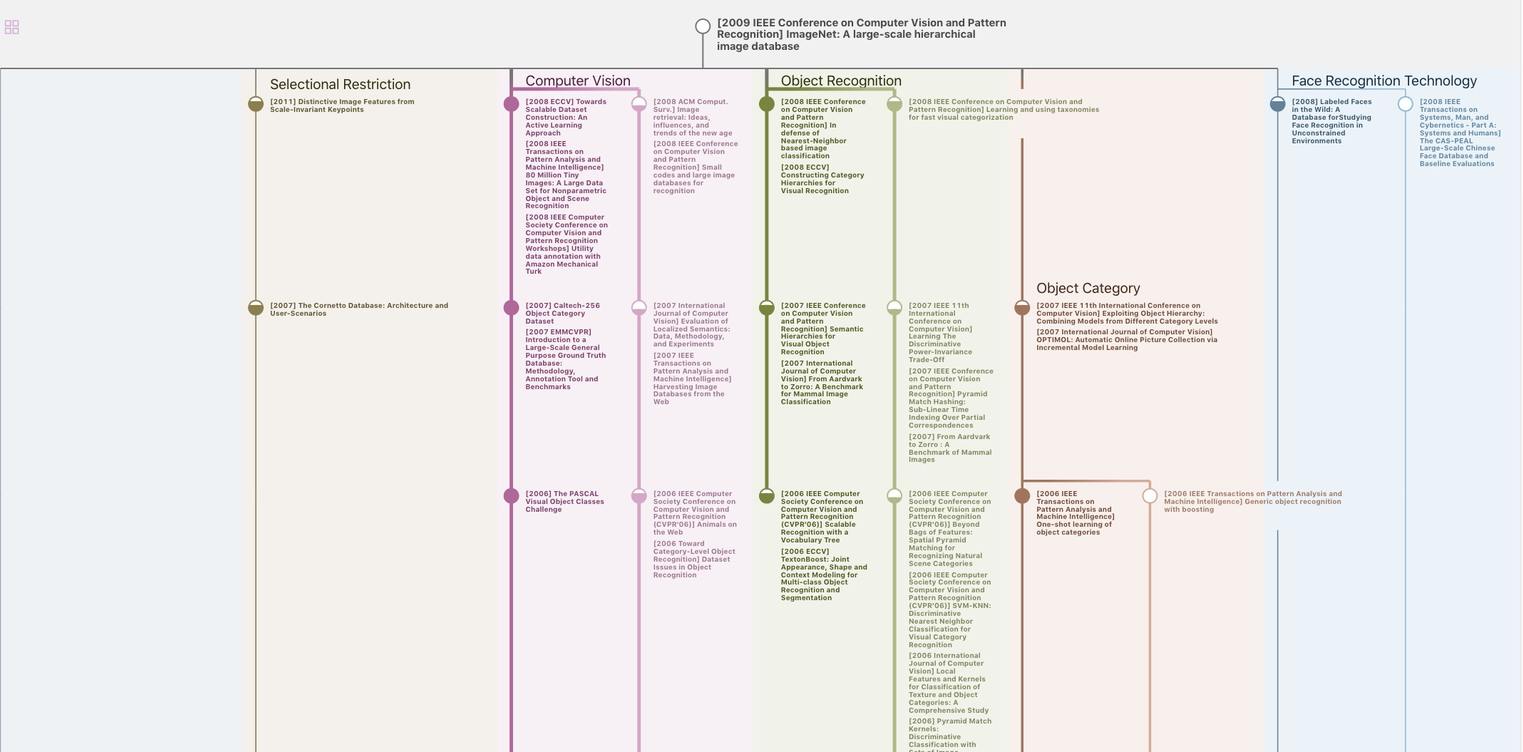
生成溯源树,研究论文发展脉络
Chat Paper
正在生成论文摘要