A Parallel Teacher for Synthetic-to-Real Domain Adaptation of Traffic Object Detection
IEEE Transactions on Intelligent Vehicles(2022)
摘要
Large-scale synthetic traffic image datasets have been widely used to make compensate for the insufficient data in real world. However, the mismatch in domain distribution between synthetic datasets and real datasets hinders the application of the synthetic dataset in the actual vision system of intelligent vehicles. In this paper, we propose a novel synthetic-to-real domain adaptation method to settle the mismatch domain distribution from two aspects, i.e., data level and knowledge level. On the data level, a Style-Content Discriminated Data Recombination (SCD-DR) module is proposed, which decouples the style from content and recombines style and content from different domains to generate a hybrid domain as a transition between synthetic and real domains. On the knowledge level, a novel Iterative Cross-Domain Knowledge Transferring (ICD-KT) module including source knowledge learning, knowledge transferring and knowledge refining is designed, which achieves not only effective domain-invariant feature extraction, but also transfers the knowledge from labeled synthetic images to unlabeled actual images. Comprehensive experiments on public virtual and real dataset pairs demonstrate the effectiveness of our proposed synthetic-to-real domain adaptation approach in object detection of traffic scenes.
更多查看译文
关键词
Computer vision,Unsupervised Domain Adaptation,Teacher-student learning,Traffic object detection
AI 理解论文
溯源树
样例
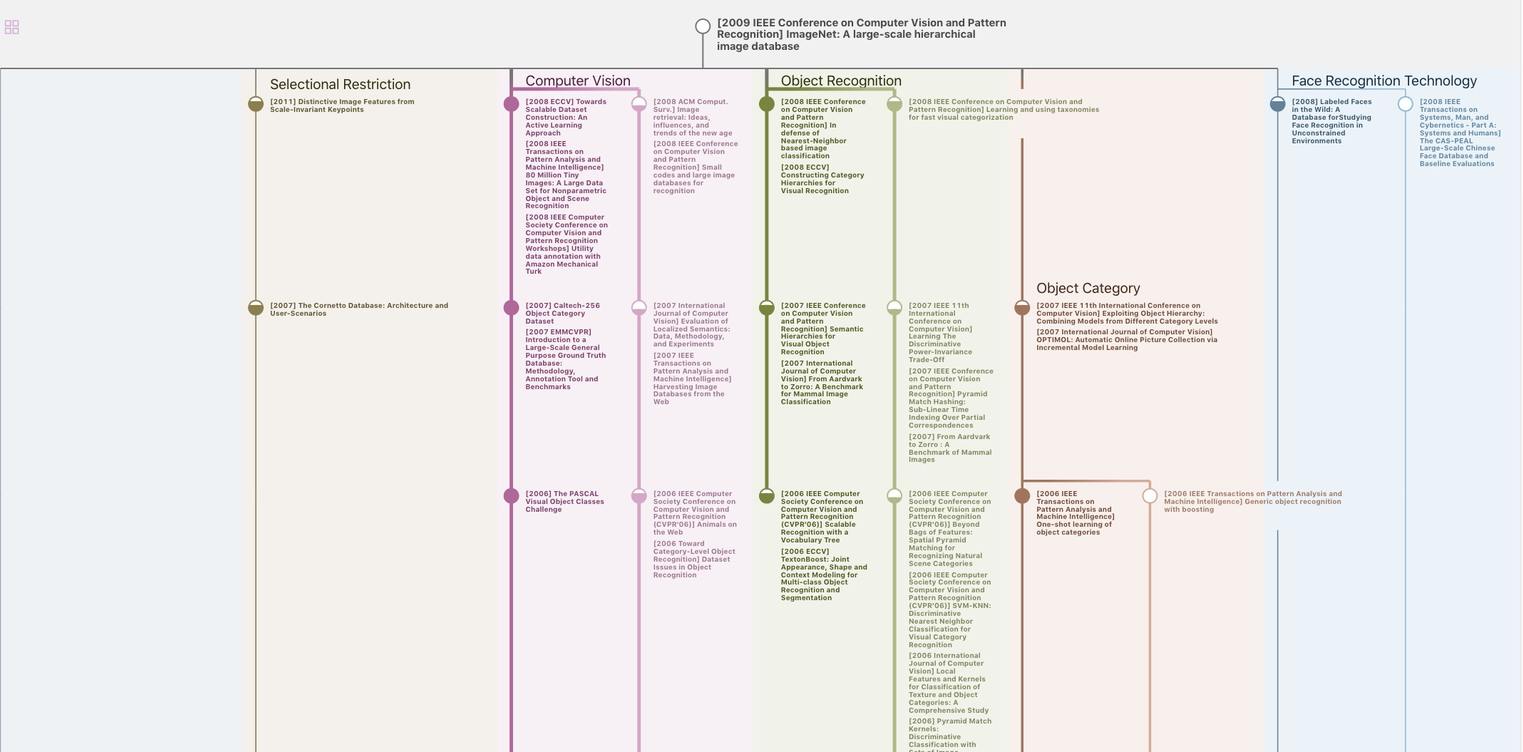
生成溯源树,研究论文发展脉络
Chat Paper
正在生成论文摘要