From Model Selection to Maps: A Completely Design‐based Data‐driven Inference for Mapping Forest Resources
Environmetrics(2022)
摘要
A completely data‐driven, design‐based sampling strategy is proposed for mapping a forest attribute within the spatial units tessellating a survey region. Based on sample data, a model is selected, and model parameters are estimated using least‐squares criteria for predicting the attribute of interest within units as a linear function of a set of auxiliary variables. The spatial interpolation of residuals arising from model predictions is performed by inverse distance weighting. The leave‐one‐out cross validation procedure is adopted for selecting the smoothing parameter used for interpolation. The densities of the attributes of interest within units are estimated by summing predictions and interpolated residuals. Finally, density estimates are rescaled to match the total estimate over the survey region obtained by the traditional regression estimator with the total estimate obtained from the map as the sum of the density estimates within units. A bootstrap procedure accounts for the uncertainty. The consistency of the strategy is proven by incorporating previous results. A simulation study is performed and an application for mapping wood volume densities in the forest estate of Rincine (Central Italy) is described.
更多查看译文
关键词
density estimation,harmonization,model selection,predictions,pseudopopulation bootstrap,regression estimator,residuals,smoothing parameter,spatial interpolation
AI 理解论文
溯源树
样例
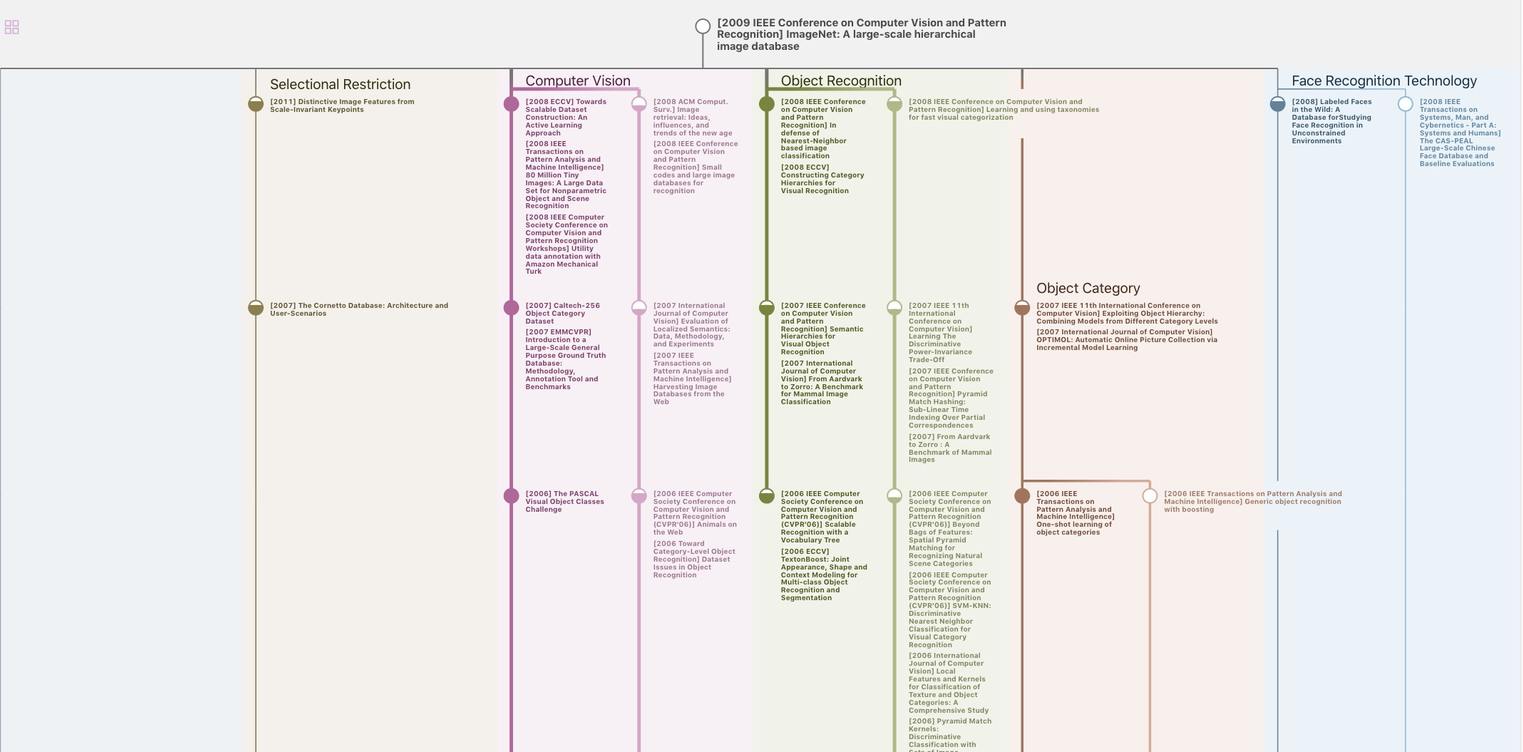
生成溯源树,研究论文发展脉络
Chat Paper
正在生成论文摘要