Multi-frame Abnormality Detection in Video Capsule Endoscopy
PROCEEDINGS OF THE FUTURE TECHNOLOGIES CONFERENCE (FTC) 2021, VOL 2(2022)
摘要
Video capsule endoscopy (VCE) provides a minimally invasive and highly effective screening mechanism for gut illness. Unfortunately, current methods require physicians to manually review the lengthy VCE recordings, which is both tedious and cost-prohibitive. Furthermore, available automation techniques are limited, and there is little publicly available data for developing such tools. This paper presents a novel multi-frame methodology for capsule localization and small bowel abnormality detection in VCE recordings. Unlike existing models, the deep-learning architecture incorporates contextual cues in adjacent frames to identify abnormalities. This approach corresponds with how physicians review VCE to identify disease and results in more efficient and effective models. To support model development, we developed a customized data collection platform and provide a mechanism for efficient annotation. The platform enabled rapid generation of annotated, full-length, VCE recordings. These annotated videos are in contrast to other publicly available VCE data which does not contain annotated video sequences with which we could train and test a multi-frame model. This platform can be easily replicated and provides a means to generate additional data to accelerate progress in machine-assisted VCE screening. our demonstration of the effectiveness of sequence-based architectures can readily be extended to other medical classification tasks.
更多查看译文
关键词
Video Capsule Endoscopy, Deep learning, Annotation tool, VCE
AI 理解论文
溯源树
样例
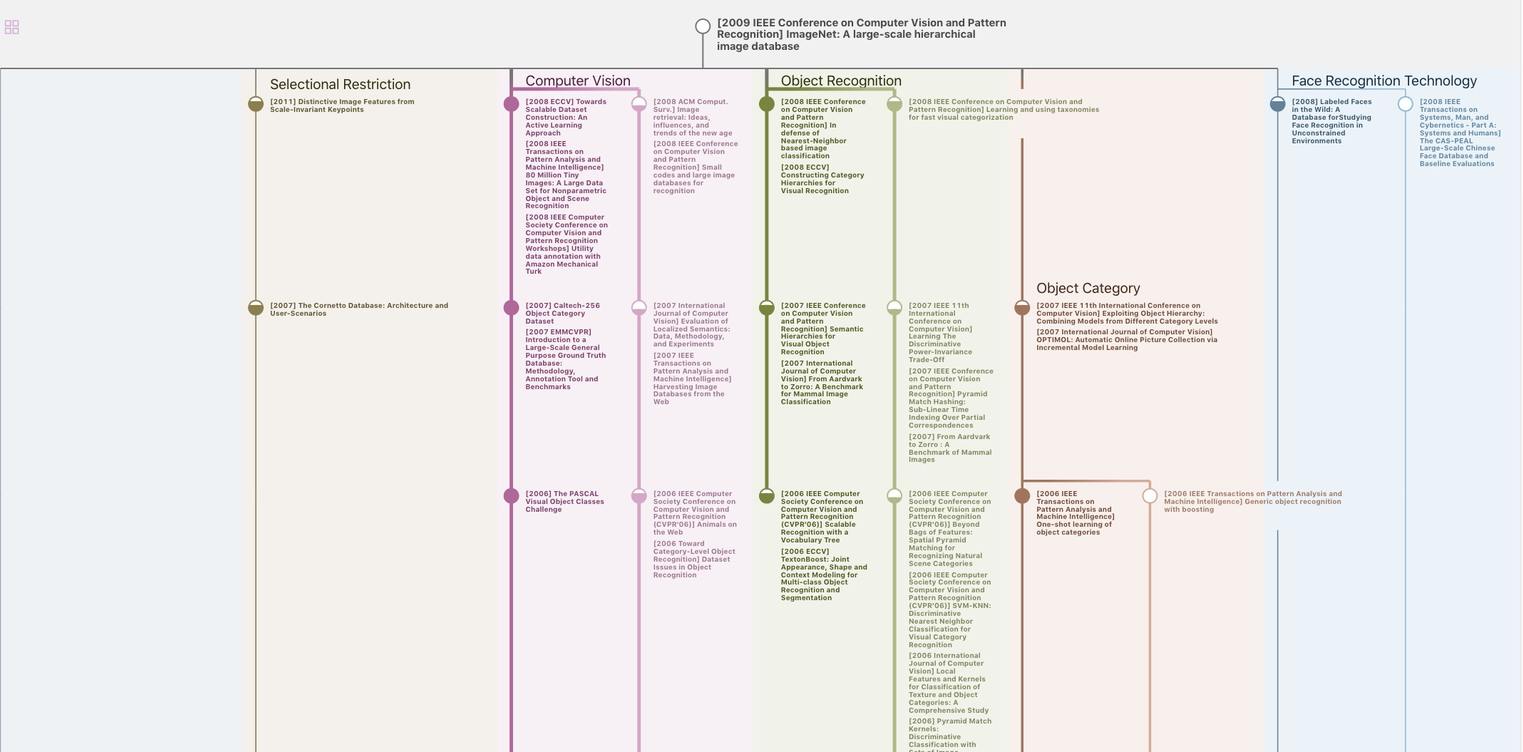
生成溯源树,研究论文发展脉络
Chat Paper
正在生成论文摘要