A machine learning protocol for revealing ion transport mechanisms from dynamic NMR shifts in paramagnetic battery materials
CHEMICAL SCIENCE(2022)
Abstract
Solid-state nuclear magnetic resonance (ssNMR) provides local environments and dynamic fingerprints of alkali ions in paramagnetic battery materials. Linking the local ionic environments and NMR signals requires expensive first-principles computational tools that have been developed for over a decade. Nevertheless, the assignment of the dynamic NMR spectra of high-rate battery materials is still challenging because the local structures and dynamic information of alkali ions are highly correlated and difficult to acquire. Herein, we develop a novel machine learning (ML) protocol that could not only quickly sample atomic configurations but also predict chemical shifts efficiently, which enables us to calculate dynamic NMR shifts with the accuracy of density functional theory (DFT). Using structurally well-defined P2-type Na-2/3(Mg1/3Mn2/3)O-2 as an example, we validate the ML protocol and show the significance of dynamic effects on chemical shifts. Moreover, with the protocol, it is demonstrated that the two experimental Na-23 shifts (1406 and 1493 ppm) of P2-type Na-2/3(Ni1/3Mn2/3)O-2 originate from two stacking sequences of transition metal (TM) layers for the first time, which correspond to space groups P6(3)/mcm and P6(3)22, respectively. This ML protocol could help to correlate dynamic ssNMR spectra with the local structures and fast transport of alkali ions and is expected to be applicable to a wide range of fast dynamic systems.
MoreTranslated text
Key words
Materials Discovery,Materials Informatics
AI Read Science
Must-Reading Tree
Example
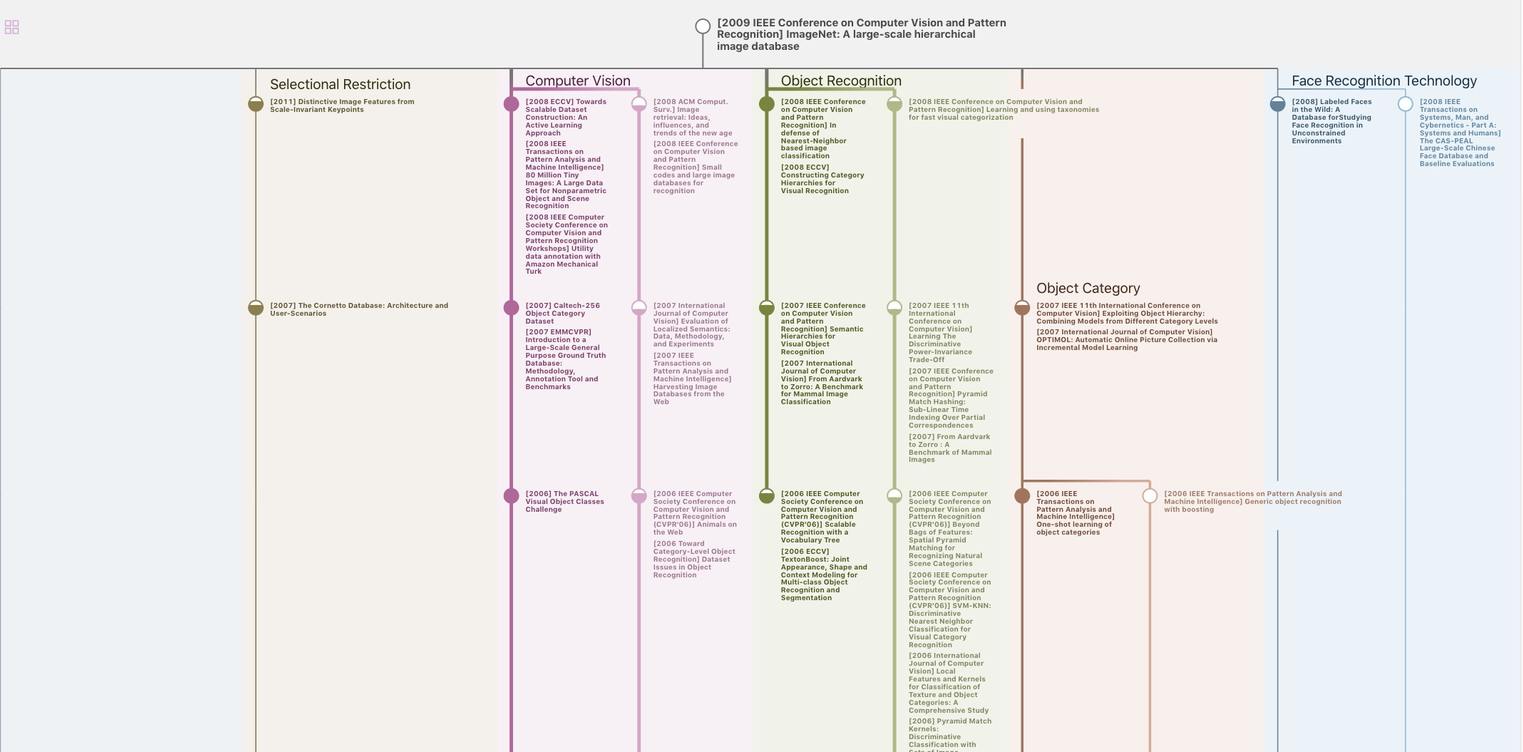
Generate MRT to find the research sequence of this paper
Chat Paper
Summary is being generated by the instructions you defined