Eicab: A Novel Deep Learning Pipeline for Circle of Willis Multiclass Segmentation and Analysis
NeuroImage(2022)
摘要
Background: The accurate segmentation, labeling and quantification of cerebral blood vessels on MR imaging is important for basic and clinical research, yet results are not generalizable, and often require user intervention. New methods are needed to automate this process.Purpose: To automatically segment, label and quantify Circle of Willis (CW) arteries on Magnetic Resonance Angiography images using deep convolutional neural networks.Materials and methods: MRA images were pooled from three public and private databases. A total of 116 subjects (mean age 56 years +/- 21 [standard deviation]; 72 women) were used to make up the training set (N = 101) and the testing set (N = 15). In each image, fourteen arterial segments making up or surrounding the CW were manually annotated and validated by a clinical expert. Convolutional neural network (CNN) models were trained on a training set to be finally combined in an ensemble to develop eICAB. Model performances were evaluated using (1) quantitative analysis (dice score on test set) and (2) qualitative analysis (external datasets, N = 121). The reliability was assessed using multiple MRAs of healthy participants (ICC of vessel diameters and volumes on test-retest).Results: Qualitative analysis showed that eICAB correctly predicted the large, medium and small arteries in 99 +/- 0.4%, 97 +/- 1% and 88 +/- 7% of all images, respectively. For quantitative assessment, the average dice score coefficients for the large (ICAs, BA), medium (ACAs, MCAs, PCAs-P2), and small (AComm, PComm, PCAs-P1) vessels were 0.76 +/- 0.07, 0.76 +/- 0.08 and 0.41 +/- 0.27, respectively. These results were similar and, in some cases, statistically better (p < 0.05) than inter-expert annotation variability and robust to image SNR. Finally, test-retest analysis showed that the model yielded high diameter and volume reliability (ICC = 0.99).Conclusion: We have developed a quick and reliable open-source CNN-based method capable of accurately seg-menting and labeling the CW in MRA images. This method is largely independent of image quality. In the future, we foresee this approach as a critical step towards fully automated analysis of MRA databases in basic and clinical research.
更多查看译文
关键词
Magnetic Resonance Angiography,Circle of Willis,Semantic segmentation,Deep learning,Magnetic Resonance Angiography,Circle of Willis,Semantic segmentation,Deep learning,Magnetic Resonance Angiography,Circle of Willis,Semantic segmentation,Deep learning
AI 理解论文
溯源树
样例
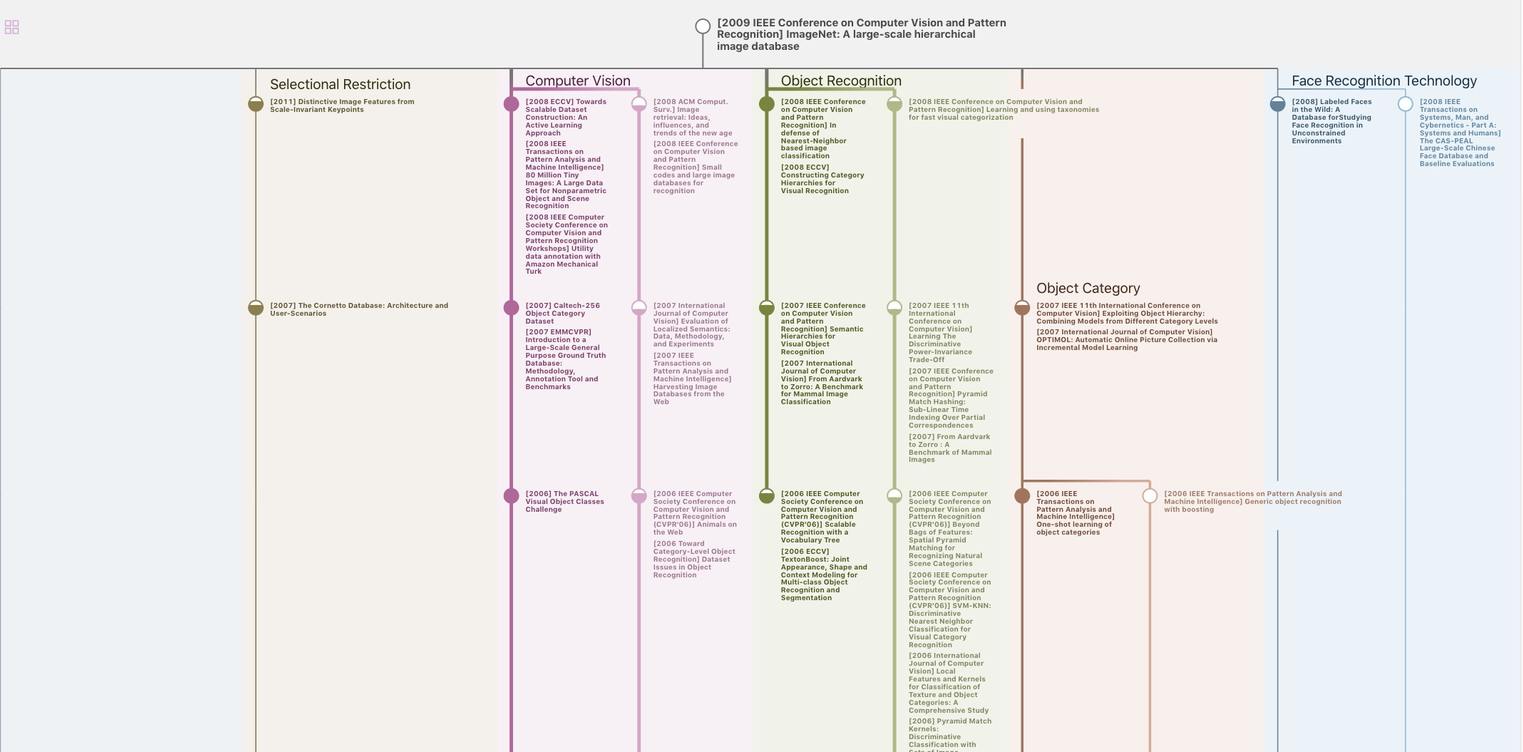
生成溯源树,研究论文发展脉络
Chat Paper
正在生成论文摘要