A Random Forest approach to predict geology from geophysics in the Pontiac subprovince, Canada
CANADIAN JOURNAL OF EARTH SCIENCES(2022)
Abstract
Current "visual" integration approaches to map geology from geophysics are challenging, biased toward user assumptions, and time consuming. Applying a supervised machine learning Random Forest method to airborne geophysical data sets is an alternative solution to quickly generate interpretativemaps, which can later be validated through targeted and therefore more efficient field campaigns. The approach is tested in the Malartic-Cadillac area of the Pontiac subprovince, Canada. Available airborne geophysical data sets include magnetic, frequency-domain electromagnetic, and radiometric data. Unlike the western part of the area, many studies have been done in the eastern part where the world-class Canadian Malartic gold deposit is located. The eastern part has abundant field observations and well-documented geology that are used as training data for Random Forest learning. A predicted map is built after preprocessing the data, gridding the area into a mesh of nodes and training using known geological knowledge. The results provide new information about the spatial distribution of lithological units, including diabase dikes, felsic-intermediate igneous rocks, mafic-ultramafic igneous rocks, and sedimentary rocks. Comparison between the predicted map and previous geological maps could suggest locations where future field mapping efforts should focus in the Malartic-Cadillac area.
MoreTranslated text
Key words
Canadian Malartic,lithological prediction,machine learning methods,radiometric,magnetic and electromagnetic methods
AI Read Science
Must-Reading Tree
Example
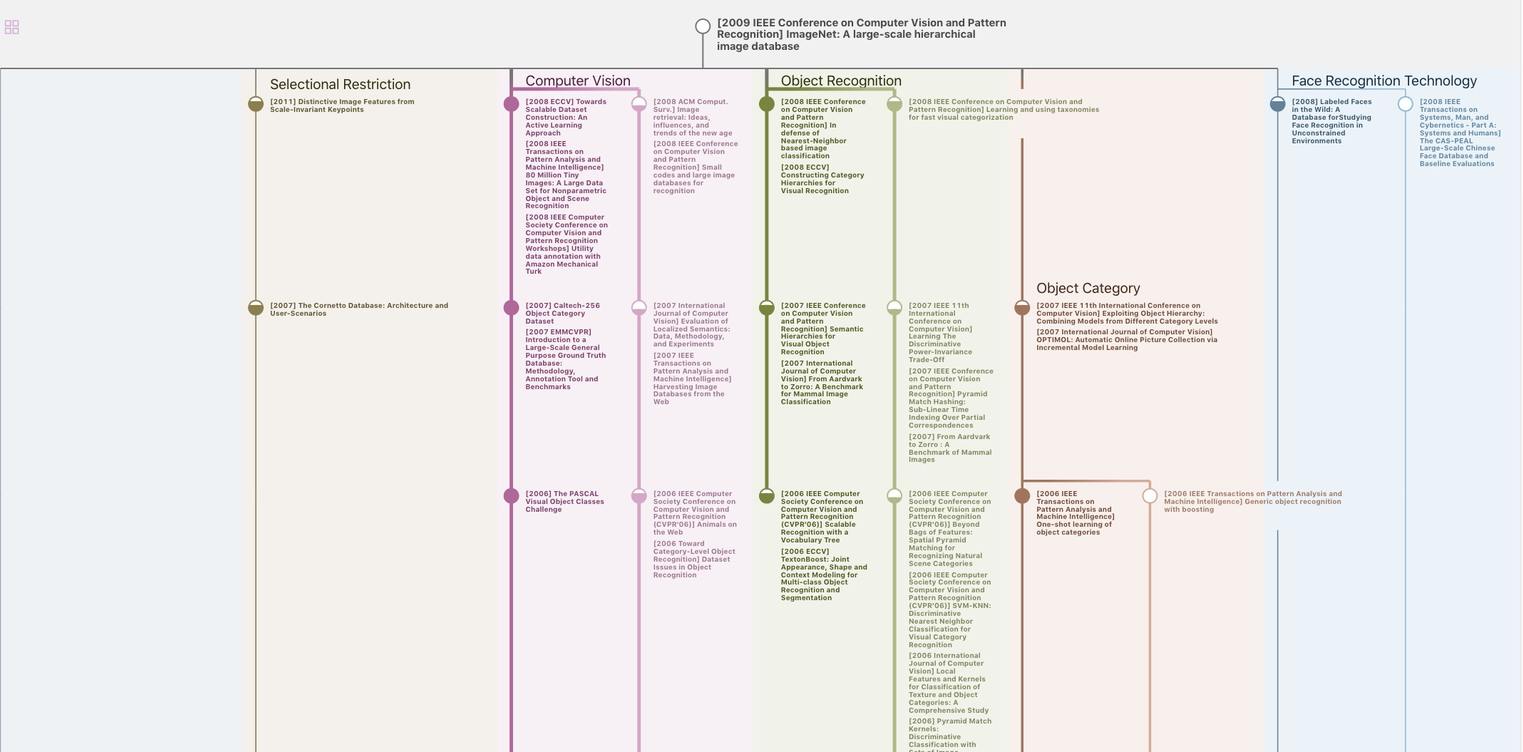
Generate MRT to find the research sequence of this paper
Chat Paper
Summary is being generated by the instructions you defined