Trace ratio criterion for multi-view discriminant analysis
Applied Intelligence(2022)
摘要
Learning from heterogeneous views, termed multi-view learning (MvL), is a significant yet challenging problem in computer vision. Many existing MvL methods apply the two-view principle to multi-view scenarios, but this pairwise approach is neither efficient nor optimal and leads to poor performance. Then some multi-view discriminant analysis methods are proposed by simultaneously considering the intra-view and inter-view correlation across multiple views, and the final form of these methods is a trace ratio (TR) problem. Traditionally, the solution to the TR problem is normally approximated by generalized eigenvalue decomposition, failing to obtain the global optimum solution. Although some iterative algorithms are proposed to directly solve the TR problem, these methods are not rigorous in the absence of theoretical discussion. To overcome these weaknesses, we propose a novel supervised MvL model called Trace Ratio Criterion for Multi-view Discriminant Analysis (TRCMvDA) by jointly learning multiple view-specific linear transforms. In particular, we propose an iterative algorithm based on the Newton-Raphson method to directly solve the TR problem and successfully avoid deviation from the original objectives. In addition, we prove the convergence and effectiveness of our algorithm theoretically and empirically. Experiments conducted on five universal datasets reveal that our TRCMvDA achieves better performance than state-of-the-art methods.
更多查看译文
关键词
Trace ratio criterion,Common space projection,Multi-view discriminant analysis,Iteration algorithm
AI 理解论文
溯源树
样例
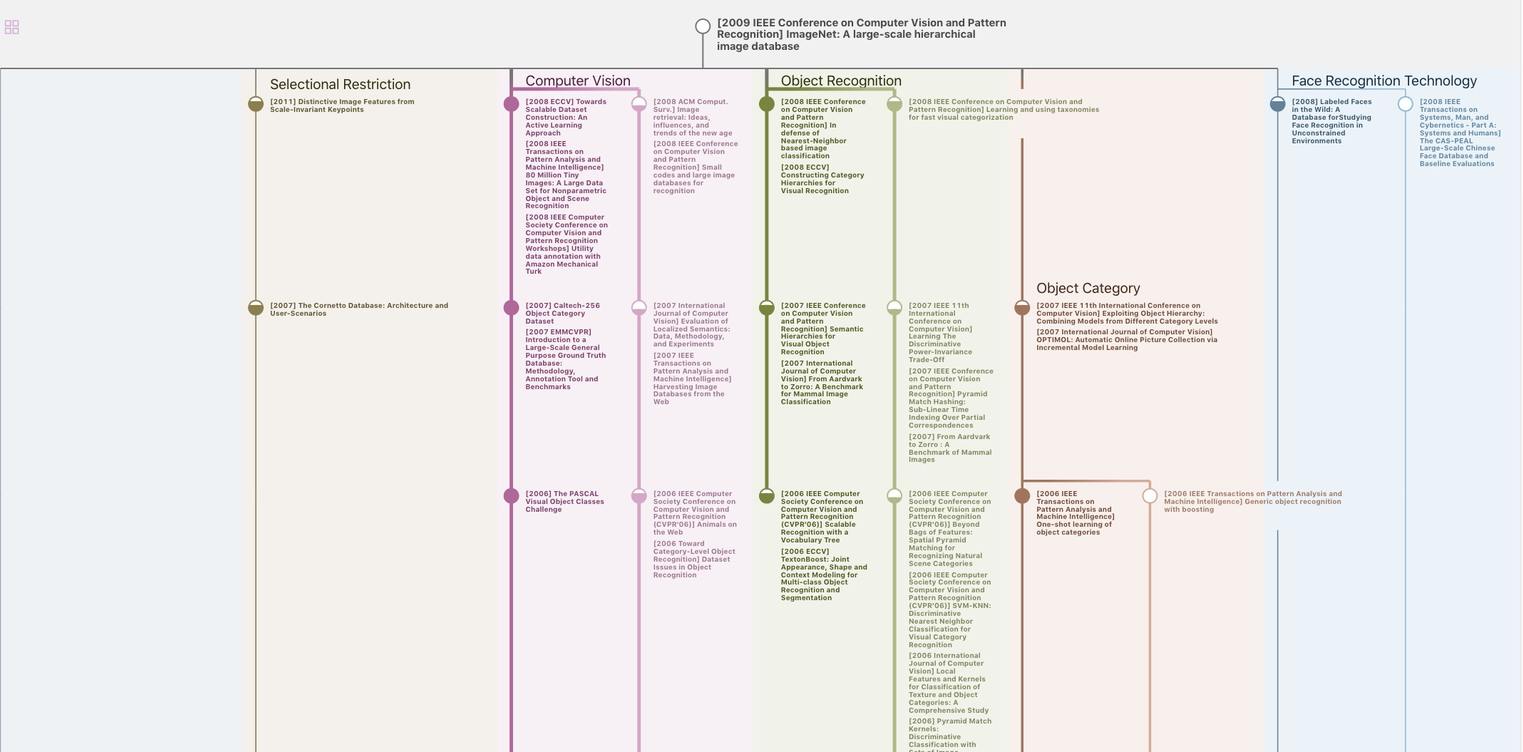
生成溯源树,研究论文发展脉络
Chat Paper
正在生成论文摘要