A Comparison of Neural-Based Visual Recognisers for Speech Activity Detection
International journal of speech technology(2022)
摘要
Existing literature on speech activity detection (SAD) highlights different approaches within neural networks but does not provide a comprehensive comparison to these methods. This is important because such neural approaches often require hardware-intensive resources. In this article, we provide a comparative analysis of three different approaches: classification with still images (CNN model), classification based on previous images (CRNN model), and classification of sequences of images (Seq2Seq model). Our experimental results using the Vid-TIMIT dataset show that the CNN model can achieve an accuracy of 97% whereas the CRNN and Seq2Seq models increase the classification to 99%. Further experiments show that the CRNN model is almost as accurate as the Seq2Seq model (99.1% vs. 99.6% of classification accuracy, respectively) but 57% faster to train (326 vs. 761 secs. per epoch).
更多查看译文
关键词
Visual speech activity recognition,Convolutional neural networks,Recurrent neural networks
AI 理解论文
溯源树
样例
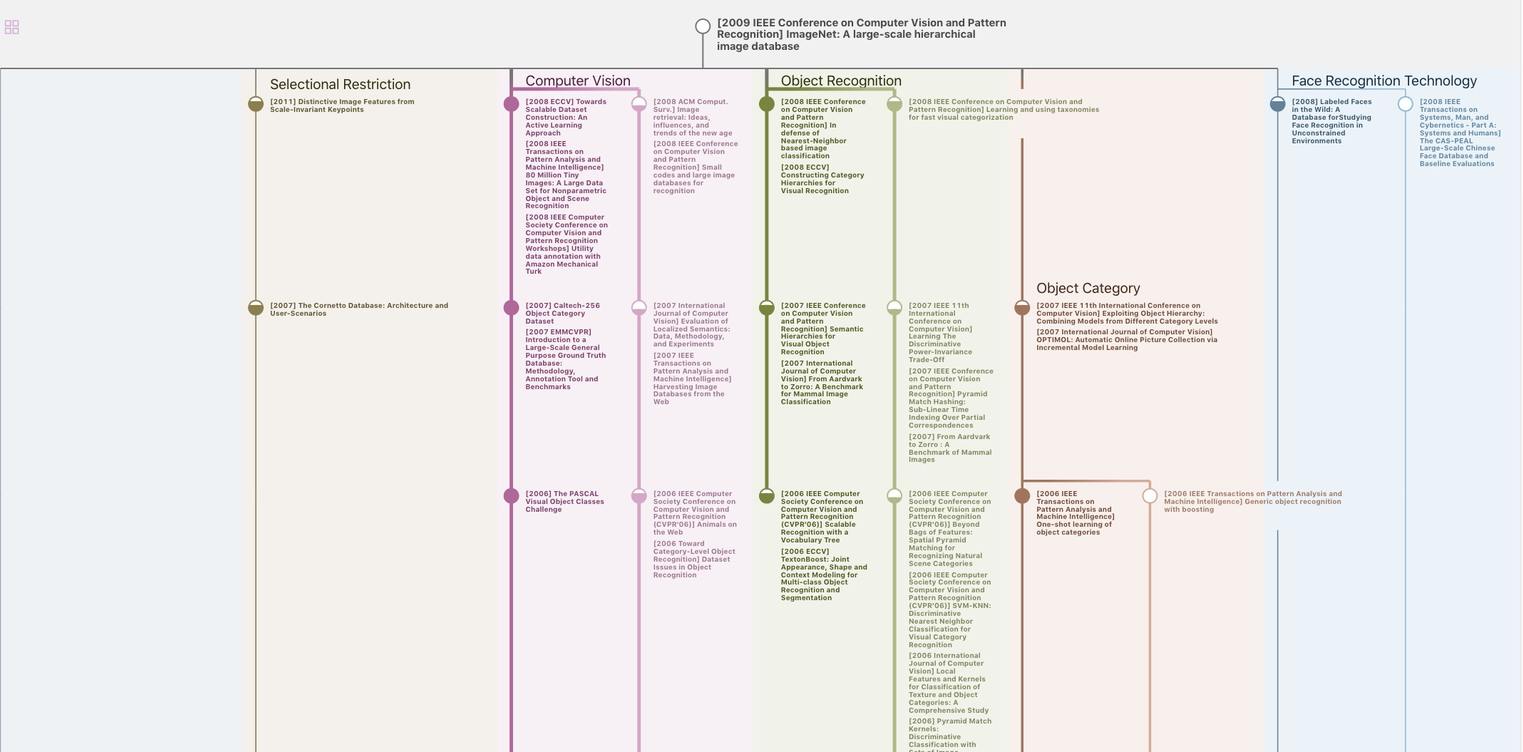
生成溯源树,研究论文发展脉络
Chat Paper
正在生成论文摘要