Wiener Graph Deconvolutional Network Improves Graph Self-Supervised Learning
AAAI'23/IAAI'23/EAAI'23 Proceedings of the Thirty-Seventh AAAI Conference on Artificial Intelligence and Thirty-Fifth Conference on Innovative Applications of Artificial Intelligence and Thirteenth Symposium on Educational Advances in Artificial Intelligence(2023)
摘要
Graph self-supervised learning (SSL) has been vastly employed to learn representations from unlabeled graphs. Existing methods can be roughly divided into predictive learning and contrastive learning, where the latter one attracts more research attention with better empirical performance. We argue that, however, predictive models weaponed with powerful decoder could achieve comparable or even better representation power than contrastive models. In this work, we propose a Wiener Graph Deconvolutional Network (WGDN), an augmentation-adaptive decoder empowered by graph wiener filter to perform information reconstruction. Theoretical analysis proves the superior reconstruction ability of graph wiener filter. Extensive experimental results on various datasets demonstrate the effectiveness of our approach.
更多查看译文
关键词
ML: Graph-based Machine Learning,ML: Unsupervised & Self-Supervised Learning
AI 理解论文
溯源树
样例
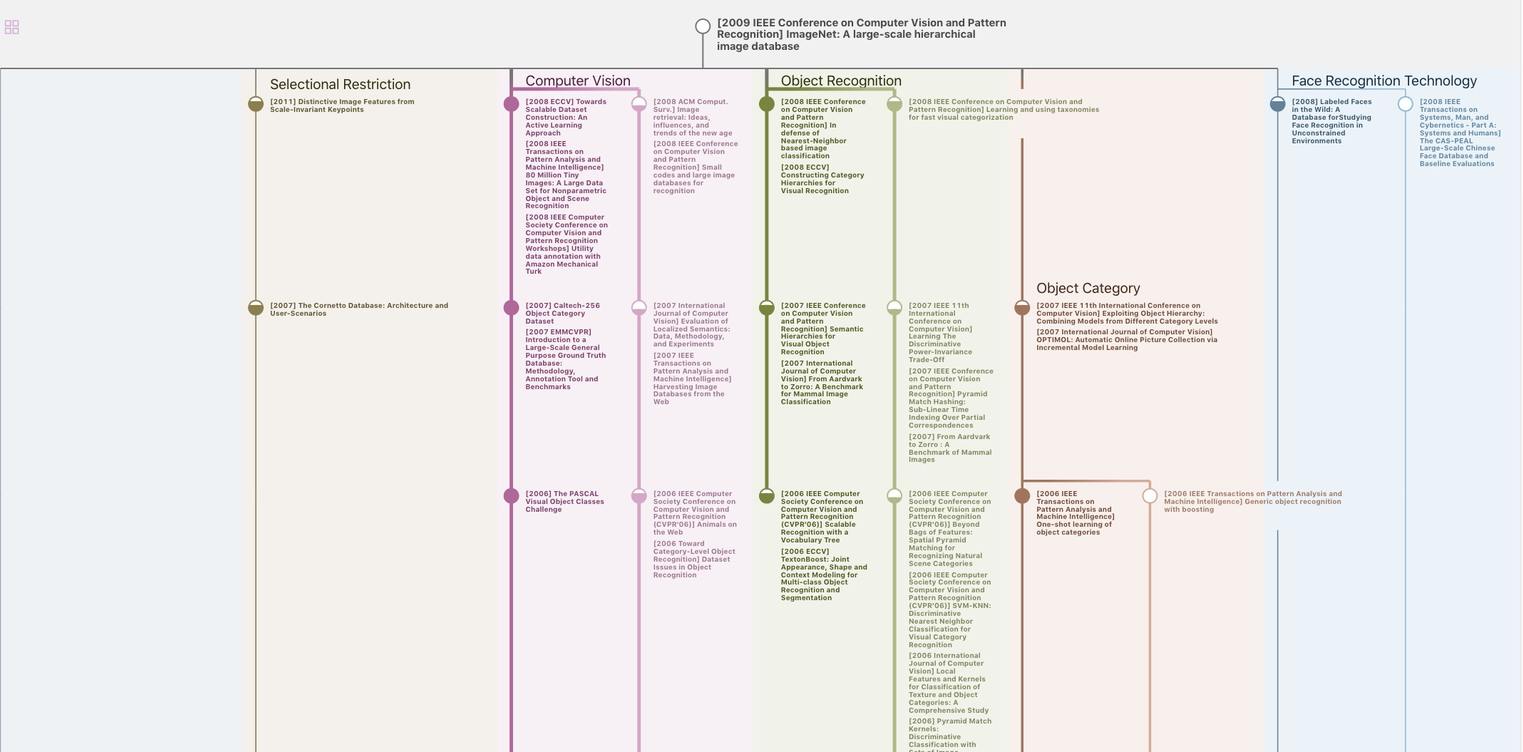
生成溯源树,研究论文发展脉络
Chat Paper
正在生成论文摘要