Deep Reinforcement Learning-based Power Allocation in Uplink Cell-Free Massive MIMO
2022 IEEE WIRELESS COMMUNICATIONS AND NETWORKING CONFERENCE (WCNC)(2022)
摘要
A cell-free massive multiple-input multiple-output (MIMO) uplink is investigated in this paper. We address a power allocation design problem that considers two conflicting metrics, namely the sum rate and fairness. Different weights are allocated to the sum rate and fairness of the system, based on the requirements of the mobile operator. The knowledge of the channel statistics is exploited to optimize power allocation. We propose to employ large scale-fading (LSF) coefficients as the input of a twin delayed deep deterministic policy gradient (TD3). This enables us to solve the non-convex sum rate fairness trade-off optimization problem efficiently. Then, we exploit a use-and-then-forget (UatF) technique, which provides a closed-form expression for the achievable rate. The sum rate fairness trade-off optimization problem is subsequently solved through a sequential convex approximation (SCA) technique. Numerical results demonstrate that the proposed algorithms outperform conventional power control algorithms in terms of both the sum rate and minimum user rate. Furthermore, the TD3-based approach can increase the median of sum rate by 16%-46% and the median of minimum user rate by 11%-60% compared to the proposed SCA-based technique. Finally, we investigate the complexity and convergence of the proposed scheme.
更多查看译文
关键词
Cell-free massive MIMO,deep reinforcement learning,fairness,power control,sequential convex approximation
AI 理解论文
溯源树
样例
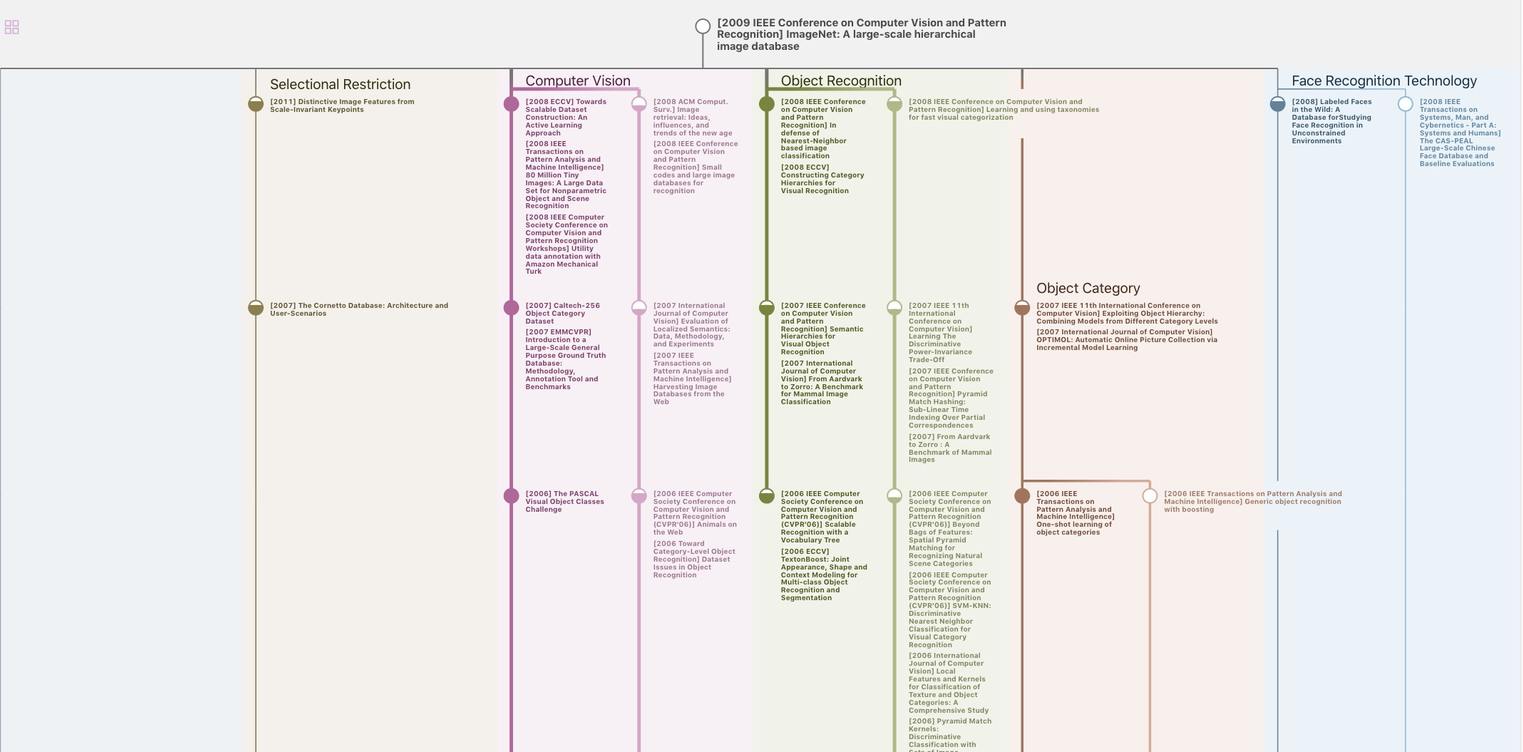
生成溯源树,研究论文发展脉络
Chat Paper
正在生成论文摘要