CTooth: A Fully Annotated 3D Dataset and Benchmark for Tooth Volume Segmentation on Cone Beam Computed Tomography Images
INTELLIGENT ROBOTICS AND APPLICATIONS (ICIRA 2022), PT IV(2022)
摘要
3D tooth segmentation is a prerequisite for computer-aided dental diagnosis and treatment. However, segmenting all tooth regions manually is subjective and time-consuming. Recently, deep learning-based segmentation methods produce convincing results and reduce manual annotation efforts, but it requires a large quantity of ground truth for training. To our knowledge, there are few tooth data available for the 3D segmentation study. In this paper, we establish a fully annotated cone beam computed tomography dataset CTooth with tooth gold standard. This dataset contains 22 volumes (7363 slices) with fine tooth labels annotated by experienced radiographic interpreters. To ensure a relative even data sampling distribution, data variance is included in the CTooth including missing teeth and dental restoration. Several state-of-the-art segmentation methods are evaluated on this dataset. Afterwards, we further summarise and apply a series of 3D attention-based Unet variants for segmenting tooth volumes. This work provides a new benchmark for the tooth volume segmentation task. Experimental evidence proves that attention modules of the 3D UNet structure boost responses in tooth areas and inhibit the influence of background and noise. The best performance is achieved by 3D Unet with SKNet attention module, of 88.04% Dice and 78.71% IOU, respectively. The attention-based Unet framework outperforms other state-of-the-art methods on the CTooth dataset. The codebase and dataset are released here.
更多查看译文
关键词
3D dental dataset,Tooth segmentation,Attention
AI 理解论文
溯源树
样例
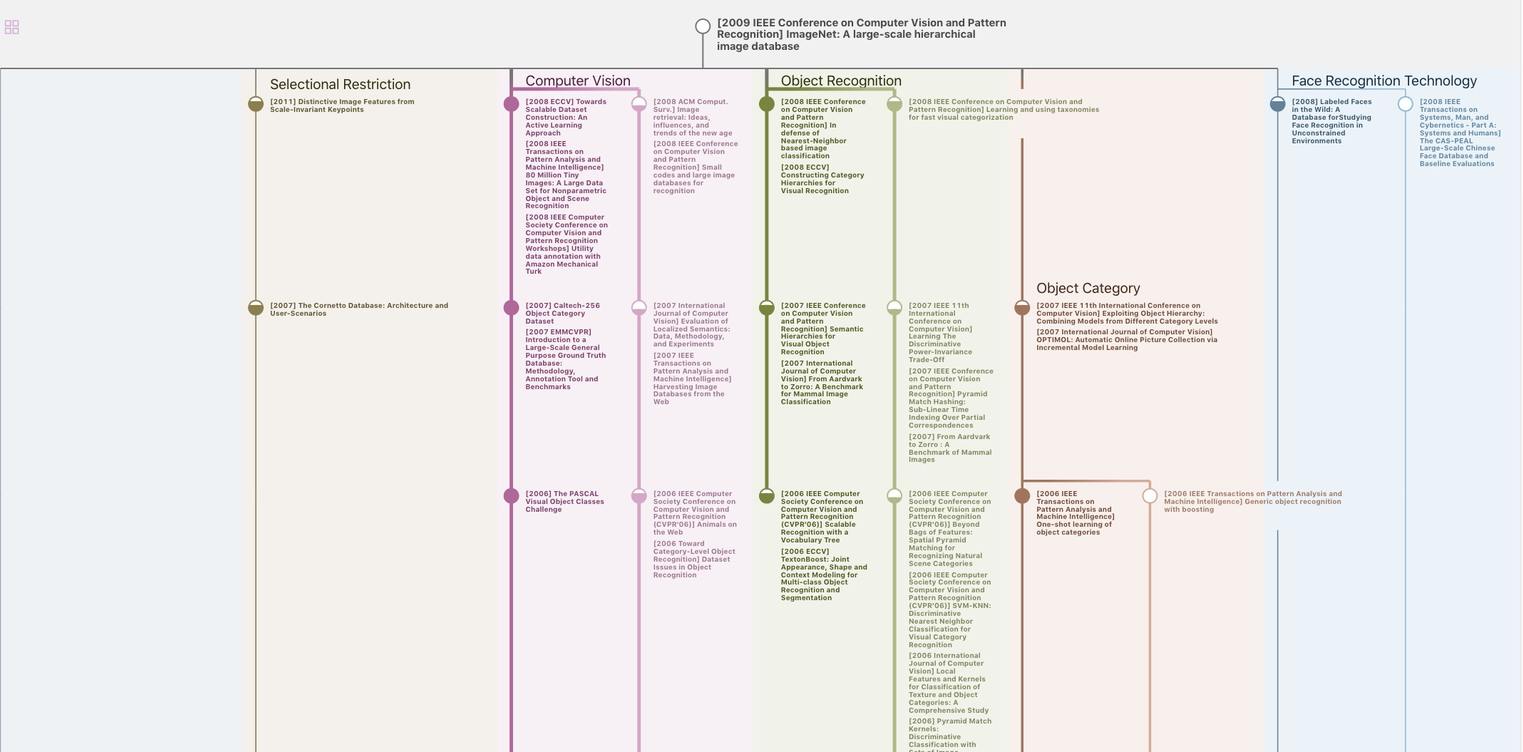
生成溯源树,研究论文发展脉络
Chat Paper
正在生成论文摘要