Data Driven Fault Detection of Premixer Centerbody Degradation in a Swirl Combustor
Volume 4A: Combustion, Fuels, and Emissions(2020)
摘要
Abstract This paper introduces a data-driven framework for combustor-focused, performance-based condition monitoring of gas turbines. Commercial condition monitoring systems typically generate huge amounts of data that make efficient onboard monitoring challenging. This paper focuses on quantifying combustor component degradation, using premixer centerbody degradation in a swirl stabilized combustor as a case study. The input for these analyses is acoustic pressure measurements acquired at various locations on the combustor. The diagnosis methodology is based on a classification framework and consists of 3 steps: 1) Data curation, 2) Feature Engineering, and 3) Diagnosis. Data curation ensures good quality of the data that is passed through the algorithm. Feature engineering deals with the extraction of the most informative features, from the most informative sensors, that can accurately capture the introduced fault. To perform diagnosis, the classification model is trained using experimentally acquired data and is then tested on a separate data set. The framework was able to achieve high classification accuracy (>99%) for training size as low as 30% of the total recorded observations. The low number of features required to achieve this accuracy suggests high potential for integration into existing onboard condition monitoring systems.
更多查看译文
关键词
premixer centerbody degradation,fault detection
AI 理解论文
溯源树
样例
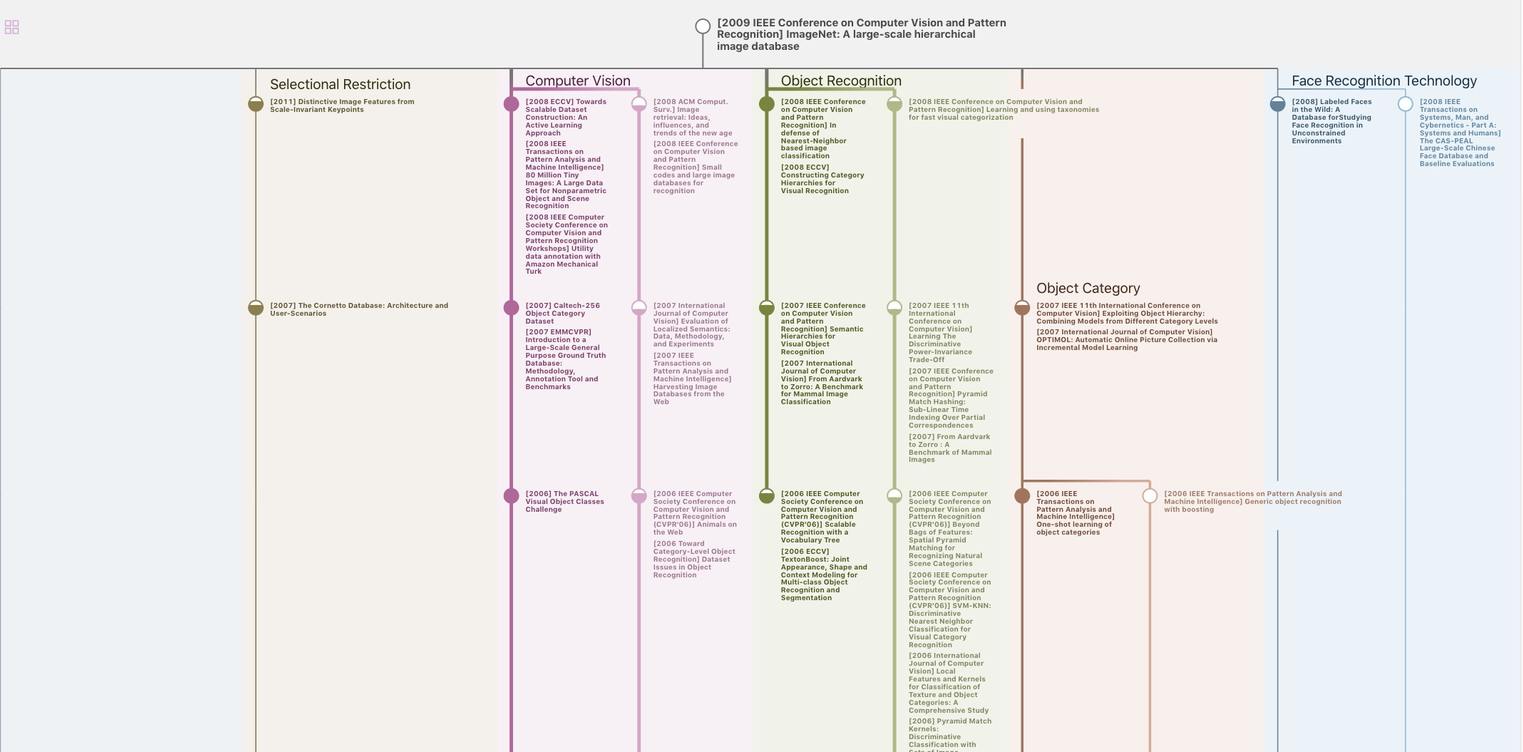
生成溯源树,研究论文发展脉络
Chat Paper
正在生成论文摘要