An alternative class of models to position social network groups in latent spaces
BRAZILIAN JOURNAL OF PROBABILITY AND STATISTICS(2021)
摘要
Identifying key nodes, estimating the probability of connection between them, and distinguishing latent groups are some of the main objectives of social network analysis. In this paper, we propose a class of blockmodels to model stochastic equivalence and visualize groups in an unobservable space. In this setting, the proposed method is based on two approaches: latent distances and latent dissimilarities at the group level. The projection proposed in the paper is performed without needing to project individuals, unlike the main approaches in the literature. Our approach can be used in undirected or directed graphs and is flexible enough to cluster and quantify between and within-group tie probabilities in social networks. The effectiveness of the methodology in representing groups in latent spaces was analyzed under artificial datasets and in two case studies.
更多查看译文
关键词
Blockmodel, social networks, multidimensional scaling, latent space, visualization
AI 理解论文
溯源树
样例
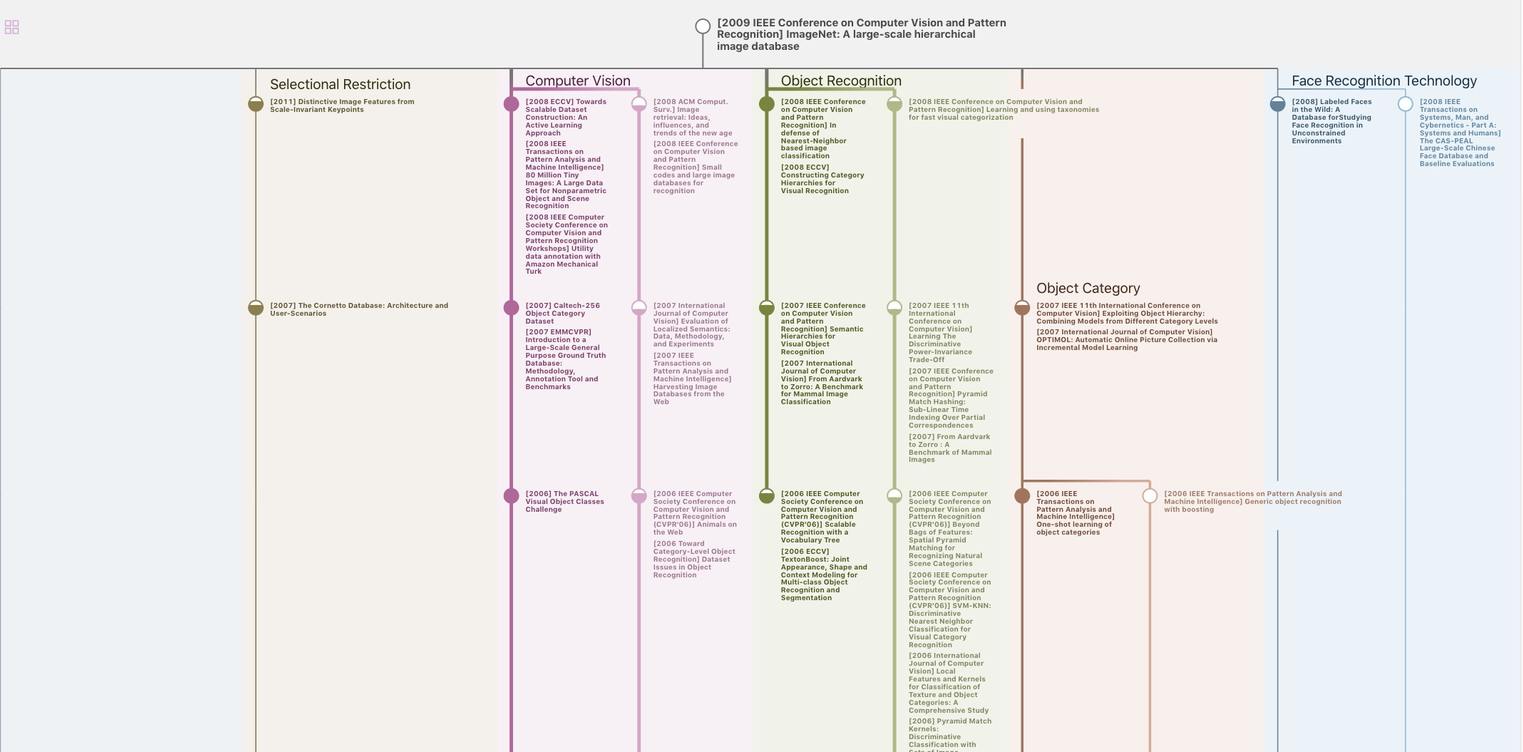
生成溯源树,研究论文发展脉络
Chat Paper
正在生成论文摘要