Generative deep learning model for broadband acoustic metamaterial design
The Journal of the Acoustical Society of America(2021)
Abstract
This study presents a promising data-driven approach for approximating and minimizing acoustic wave scattering in 2D acoustic cloak design, in which the optimal arrangement of cylindrical structures is found via a combination of generative deep learning models and optimization based on data generated by multiple scattering theory. In forward design, a fully connected neural network and convolutional neural network is trained with binary images of planar configurations of rigid cylinders to predict the total scattering cross section (TSCS) at discrete values of normalized wavenumber. In inverse design, generative deep learning models are developed that encode cylindrical structures into a continuous representation called a latent vector, and new structures are generated by decoding random latent values in this representation. In these models, the variational autoencoders, supervised and unsupervised learning, and Gaussian process are combined to predict optimal arrangements of scatterers for the desired minimal TSCS. Comparison of the scattered wavefield between the generated configurations and the numerical result shows the validity and effectiveness of the proposed method.
MoreTranslated text
Key words
metamaterial design,generative deep learning model,broadband acoustic,deep learning
AI Read Science
Must-Reading Tree
Example
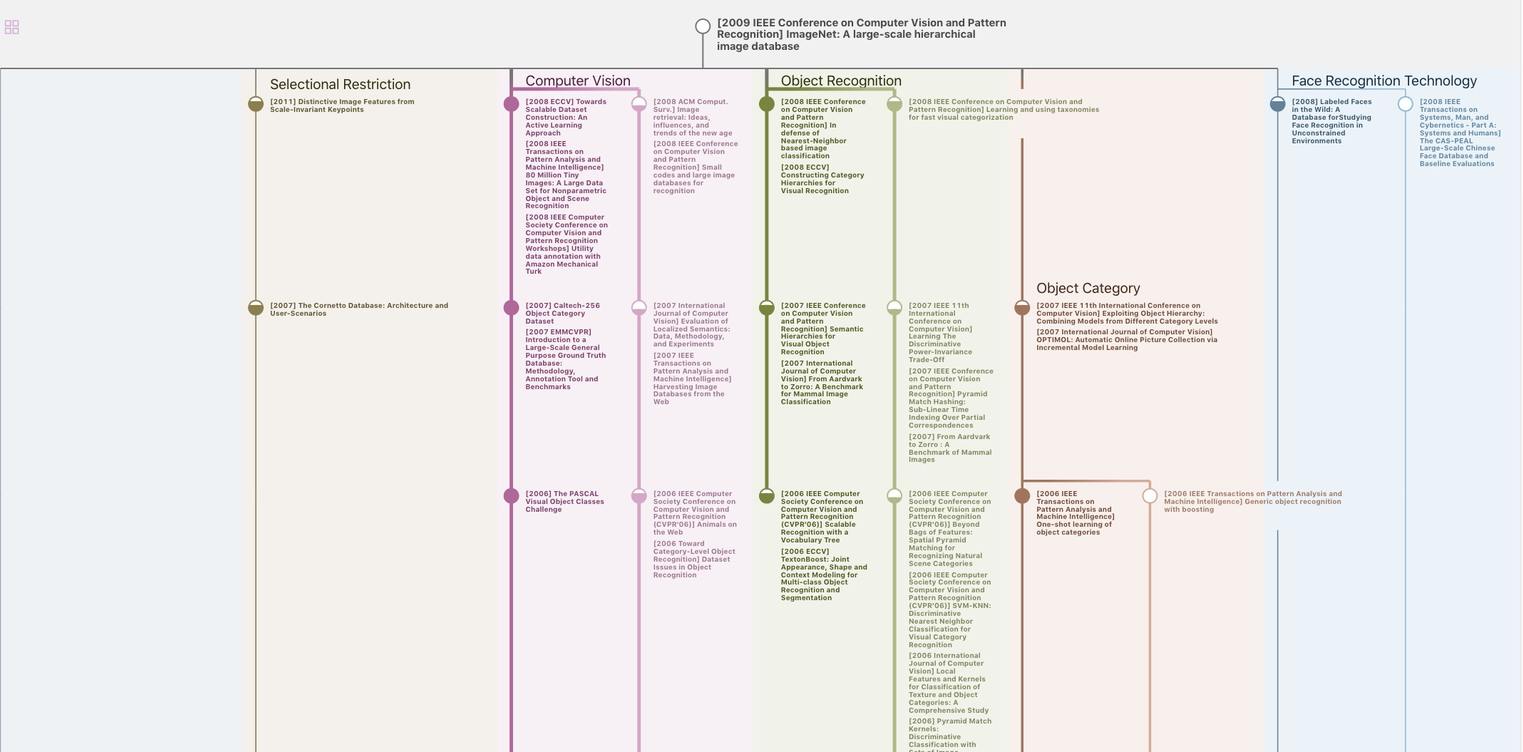
Generate MRT to find the research sequence of this paper
Chat Paper
Summary is being generated by the instructions you defined