Additive Logistic Mechanism for Privacy-Preserving Self-Supervised Learning
CoRR(2022)
Abstract
We study the privacy risks that are associated with training a neural network's weights with self-supervised learning algorithms. Through empirical evidence, we show that the fine-tuning stage, in which the network weights are updated with an informative and often private dataset, is vulnerable to privacy attacks. To address the vulnerabilities, we design a post-training privacy-protection algorithm that adds noise to the fine-tuned weights and propose a novel differential privacy mechanism that samples noise from the logistic distribution. Compared to the two conventional additive noise mechanisms, namely the Laplace and the Gaussian mechanisms, the proposed mechanism uses a bell-shaped distribution that resembles the distribution of the Gaussian mechanism, and it satisfies pure $\epsilon$-differential privacy similar to the Laplace mechanism. We apply membership inference attacks on both unprotected and protected models to quantify the trade-off between the models' privacy and performance. We show that the proposed protection algorithm can effectively reduce the attack accuracy to roughly 50\%-equivalent to random guessing-while maintaining a performance loss below 5\%.
MoreTranslated text
Key words
additive logistic mechanism,privacy-preserving,self-supervised
AI Read Science
Must-Reading Tree
Example
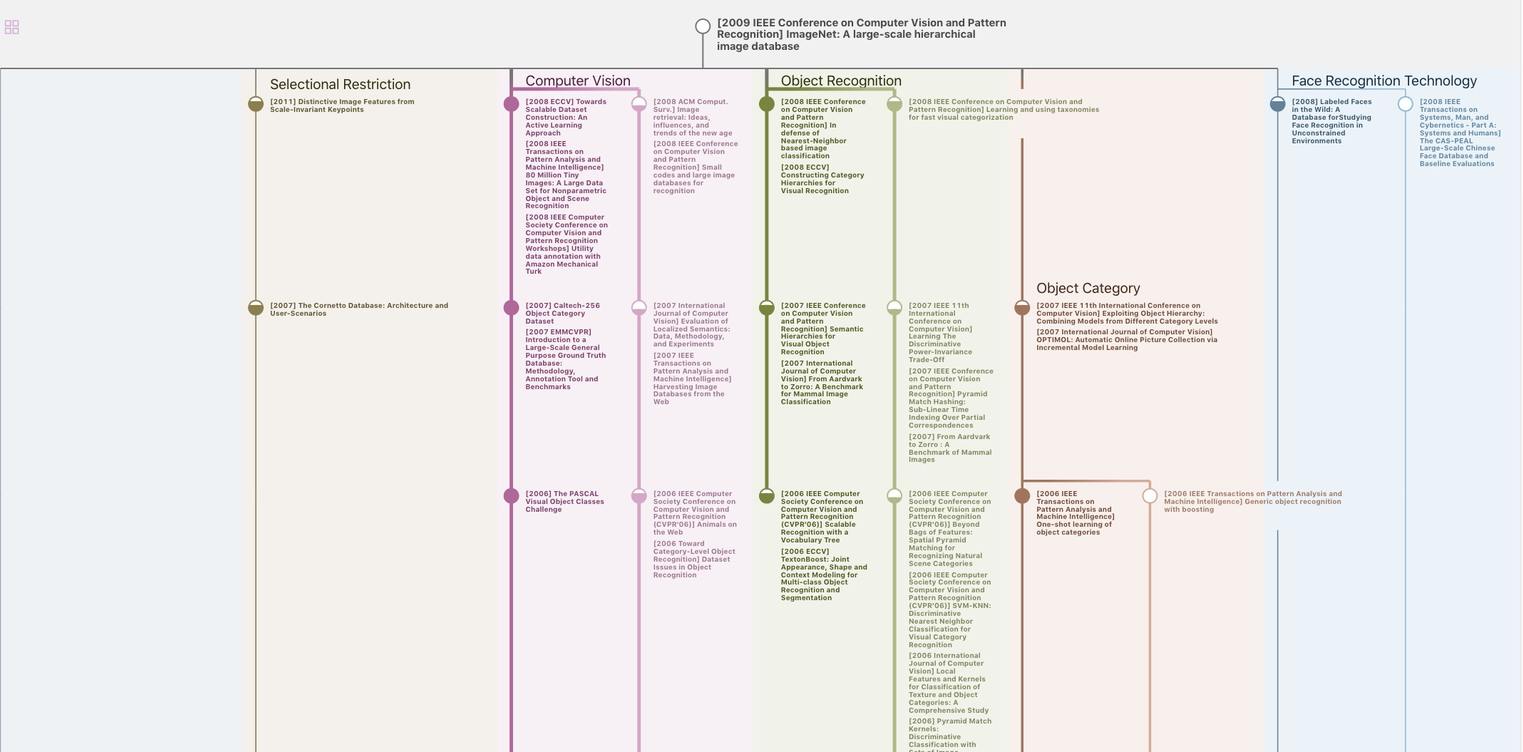
Generate MRT to find the research sequence of this paper
Chat Paper
Summary is being generated by the instructions you defined