An Attention-Based Multi-Representational Fusion Method for Social-Media-Based Text Classification
Information(2022)
摘要
There exist various text-classification tasks using user-generated contents (UGC) on social media in the big data era. In view of advantages and disadvantages of feature-engineering-based machine-learning models and deep-learning models, we argue that fusing handcrafted-text representation via feature engineering and data-driven deep-text representations extracted by performing deep-learning methods is conducive to enhancing text-classification capability. Given the characteristics of different deep neural networks, their complementary effect needs to be investigated. Moreover, contributions of these representations need to be adaptively learned when it comes to addressing different tasks or predicting different samples. Therefore, in this paper, we propose a novel fused deep-neural-network architecture with a hierarchical attention mechanism for text classification with social media data. Specifically, in the context that handcraft features are available, we employ the attention mechanism to adaptively fuse totally data-driven-text representation and handcrafted representation. For the generation of the data-driven-text representation, we propose a data-driven encoder that fuses text representations derived from three deep-learning methods with the attention mechanism, to adaptively select discriminative representation and explore their complementary effect. To verify the effectiveness of our approach, we performed two text-classification tasks, i.e., identifying adverse drug reaction (ADR)-relevant tweets from social media and identifying comparative-relevant reviews from an E-commerce platform. Experimental results demonstrate that our approach outperforms other baselines.
更多查看译文
关键词
text classification,social media,feature engineering,deep-learning-based representations,attention
AI 理解论文
溯源树
样例
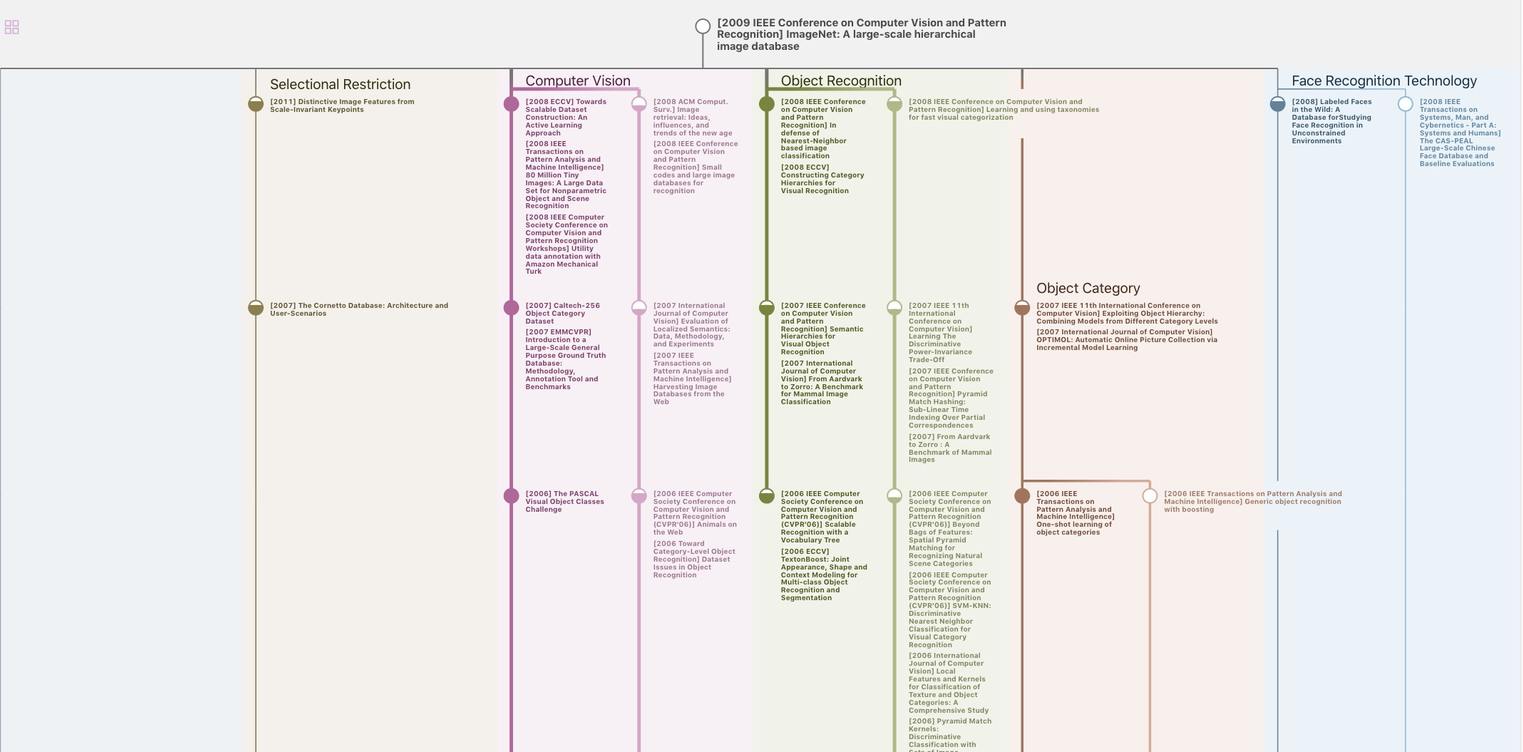
生成溯源树,研究论文发展脉络
Chat Paper
正在生成论文摘要