Deep learning of spatio-temporal information for visual tracking
Multimedia Tools and Applications(2022)
Abstract
The performance of the tracking task directly depends on target object appearance features. Therefore, a robust method for constructing appearance features is crucial for avoiding tracking failure. The tracking methods based on Convolution Neural Network (CNN) have exhibited excellent performance in the past years. However, the features from each original convolutional layer can usually represent spatial information, but not temporal information. They only use additionally the temporal information at the testing stage. To solve the lacks of prediction in the pretrained networks, we train both the spatial features and the temporal information for training at the pretraining stage. Firstly, the spatial features are trained by a domain-wise learning with the augmented data to prepare the training data to learn the temporal information. Secondly, the posterior probability maps are calculated by the particle filter and the above pretrained model. The posterior probability maps are used as the prior and the posterior respectively corresponding to the input and the output of the final network at the next stage. Thirdly, the temporal information is trained by using the augmented image sequences and the probability maps. The experimental results demonstrate that the proposed tracking method outperforms the state-of-the-art tracking methods.
MoreTranslated text
Key words
Visual tracking,Spatial features,Temporal information,Augmented data,Particle filter
AI Read Science
Must-Reading Tree
Example
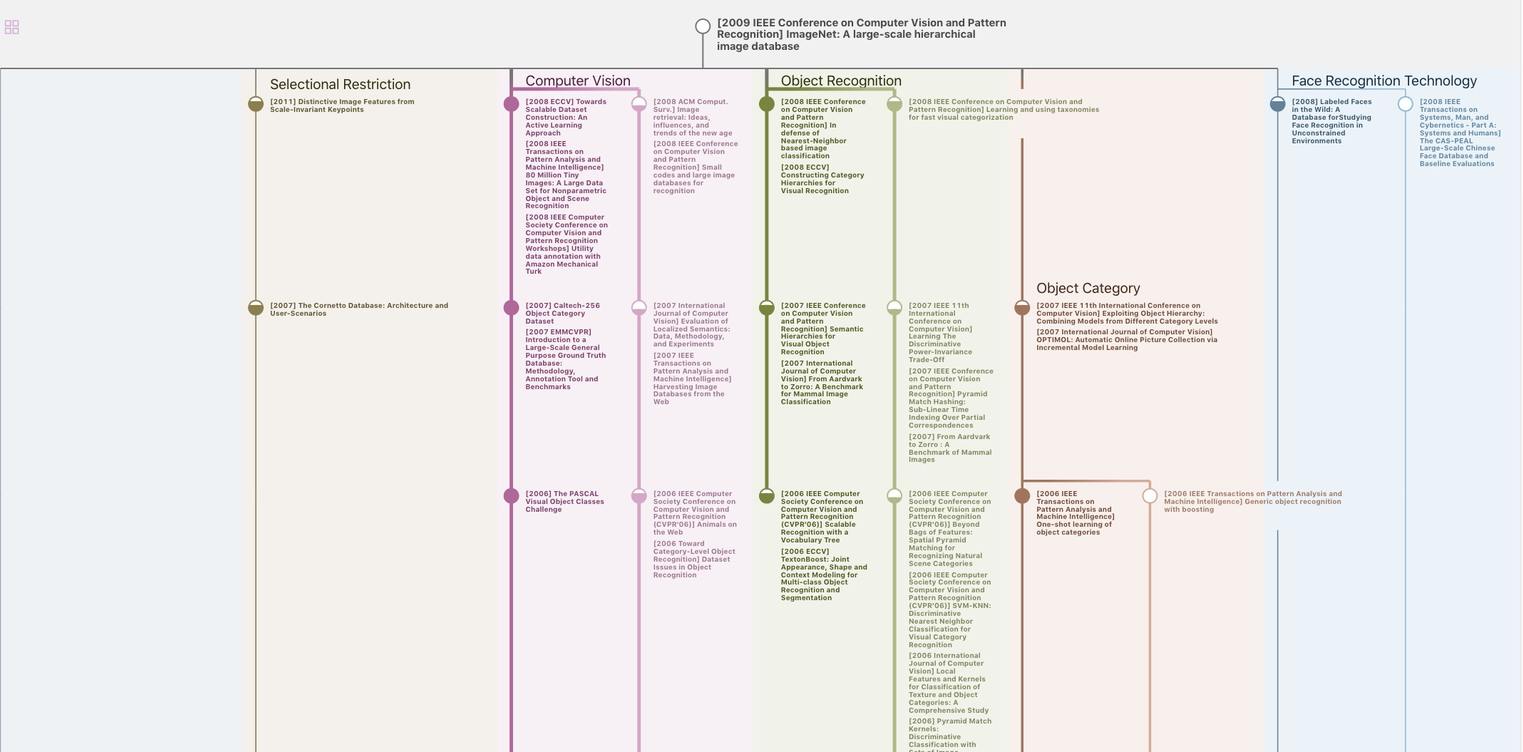
Generate MRT to find the research sequence of this paper
Chat Paper
Summary is being generated by the instructions you defined