UAV path design with connectivity constraint based on deep reinforcement learning
Physical Communication(2022)
摘要
Cellular networks are expected to communicate effectively with unmanned aerial vehicles (UAVs) and support various applications. However, existing cellular networks are primarily designed to cover users on the ground; thus, coverage holes in the sky will exist. In this paper, we investigate the problem of path design for cellular-connected UAVs, taking into account the interruption performance throughout the UAV mission to minimize the completion time. Two types of connectivity constraints requirements are assumed to be available. The first is defined as the maximum continuous time interval that the UAV loses connection with base stations (BSs) below a predefined threshold. For the second, we consider the sum outage of UAV is limited during the entire UAV mission. The UAV is tasked with flying from a starting location to a final destination while minimization the mission time, satisfying the two constraints, separately. The formulated path design problem which involves continues variables and a dynamic radio environment, is not convex and thus is extremely difficult to solve directly. To tackle this challenge, a deep reinforcement learning (DRL) based trajectory design algorithm is proposed, where the Dueling Double Deep Q Network(Dueling DDQN) with multi-steps learning method is applied. Simulation results demonstrate the effectiveness of the proposed DRL algorithm and achieve a trade-off between the trajectory length of the UAV and connection quality.
更多查看译文
关键词
Cellular-connected communication,Deep reinforcement learning,Trajectory optimization
AI 理解论文
溯源树
样例
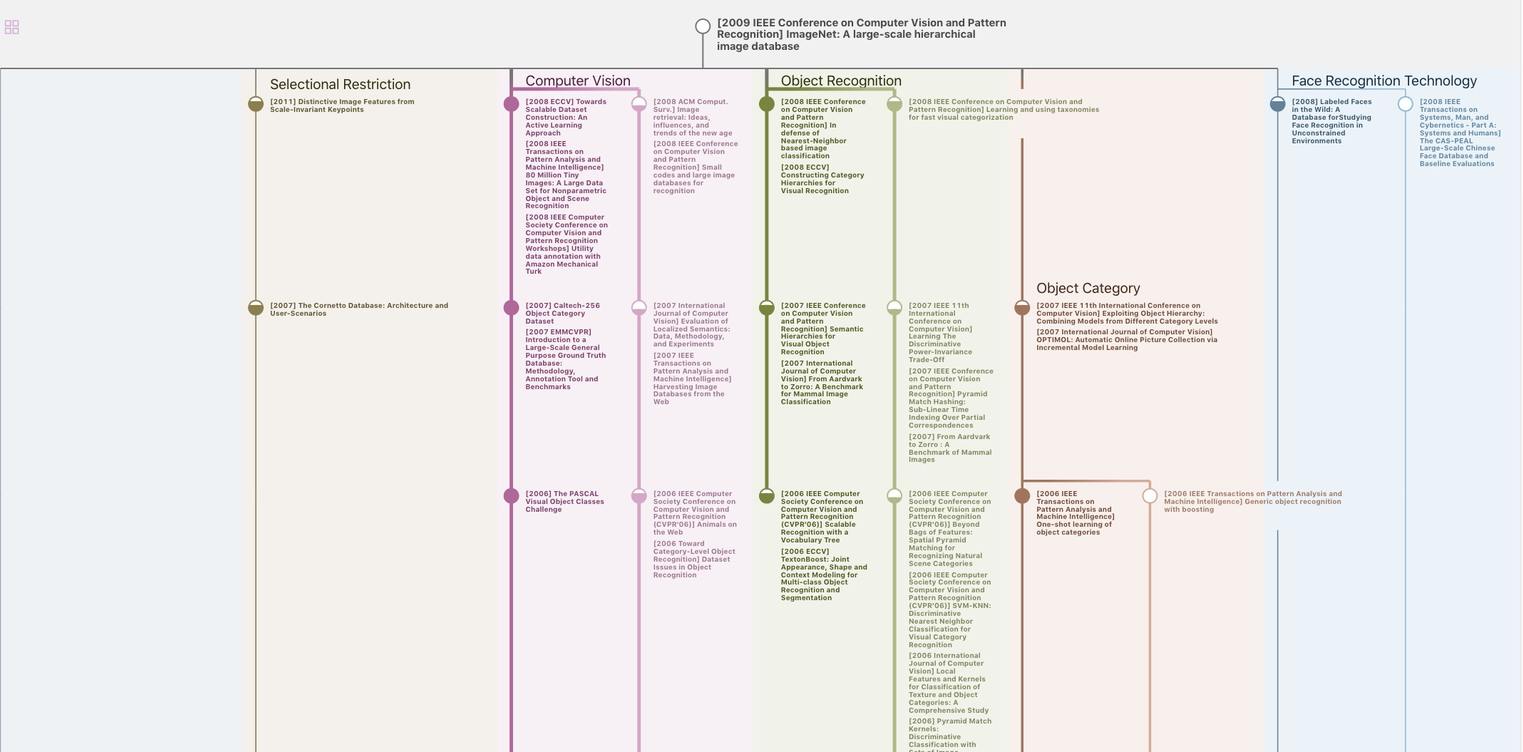
生成溯源树,研究论文发展脉络
Chat Paper
正在生成论文摘要