A Comparative Study of Datasets for Cyber-attacks Detection in Wireless Sensor Networks
2024 IEEE 3rd International Conference on Computing and Machine Intelligence (ICMI)(2024)
Abstract
Machine Learning (ML) is one of the effective security approaches to build cyber-attacks detection systems in Wireless Sensor Networks (WSNs). ML leverages the power of data analysis and pattern recognition to detect and classify various types of cyber-attacks to enhance the security of WSN s. A well-constructed dataset is one of the key factors that significantly impact the performance and generalization capabilities of any ML classifier trained on it. In this paper, we evaluate the effectiveness of two datasets: WSN-DS and WSN-BFSF which are specialized for Denial-of-Service (DoS) attacks targeting WSNs. We compare the two datasets in terms of their key characteristics, dataset quality, and ML classification performance. Mutual Information (MI) and Recursive Feature Elimination (RFE) are used for feature selection. The dataset quality is measured using statistical information calculation. The ML classification performance is investigated for three supervised ensemble techniques: LightGBM, bagging, and stacking using evaluation metrics including probability of detection, probability of false alarm, probability of misdetection, classification accuracy, model size, and processing time.
MoreTranslated text
Key words
Machine Learning,Security,cyber-attacks,Detection,Dataset Quality,Classification Performance
AI Read Science
Must-Reading Tree
Example
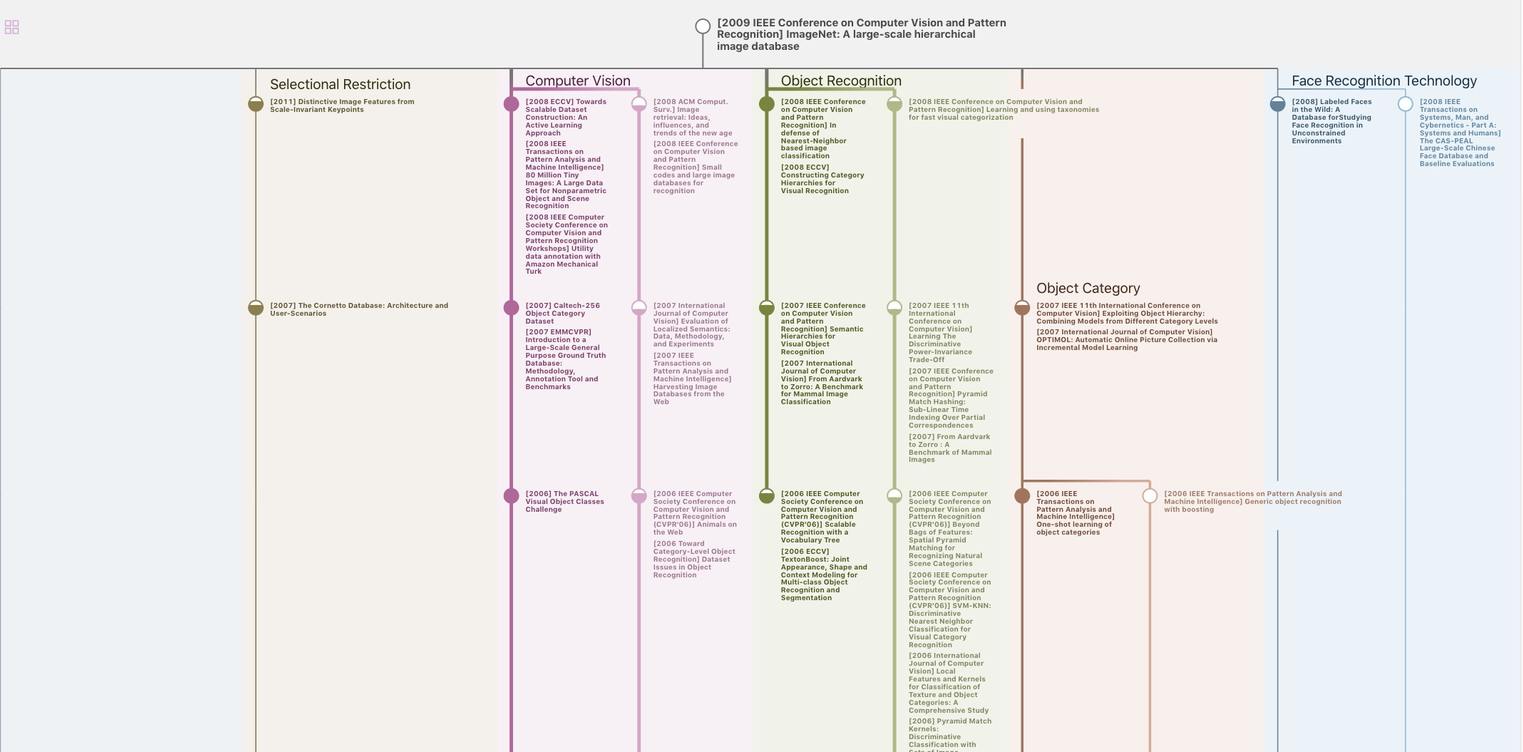
Generate MRT to find the research sequence of this paper
Chat Paper
Summary is being generated by the instructions you defined