Urban Multiple Route Planning Model Using Dynamic Programming in Reinforcement Learning
IEEE transactions on intelligent transportation systems(2022)
Abstract
With the development of the economy and the acceleration of urbanization, traffic congestion has become a worldwide problem. Advances in mobile Internet and sensor technologies have increased real-time data sharing, providing a new opportunity for urban route planning. However, due to the difficulty of handling complex global information, making correct decisions in large-scale and complex traffic environments is a problem that urgently needs to be solved. In this paper, a multiple route planning model (multi-route dynamic programming (DP) model) is proposed to solve the urban route planning problem with traffic flow information. In particular, we adopt the DP algorithm in this model, design a reward function suitable for urban path planning problems, and generate multiple routes based on the Q values. In addition, we design different scenarios using real-world road networks to test our model. Through the experiments, we demonstrate that our model has the potential to yield optimal results under large-scale scenarios with high efficiency. The advantages of integrating the distance contribution index (DCI) in the reward function are also elaborated. Moreover, our model can provide alternative routes to divert traffic from the optimal route, thus mitigating the congestion drift problem.
MoreTranslated text
Key words
Dynamic programming,model-based,multiple route planning reinforcement learning,route planning
AI Read Science
Must-Reading Tree
Example
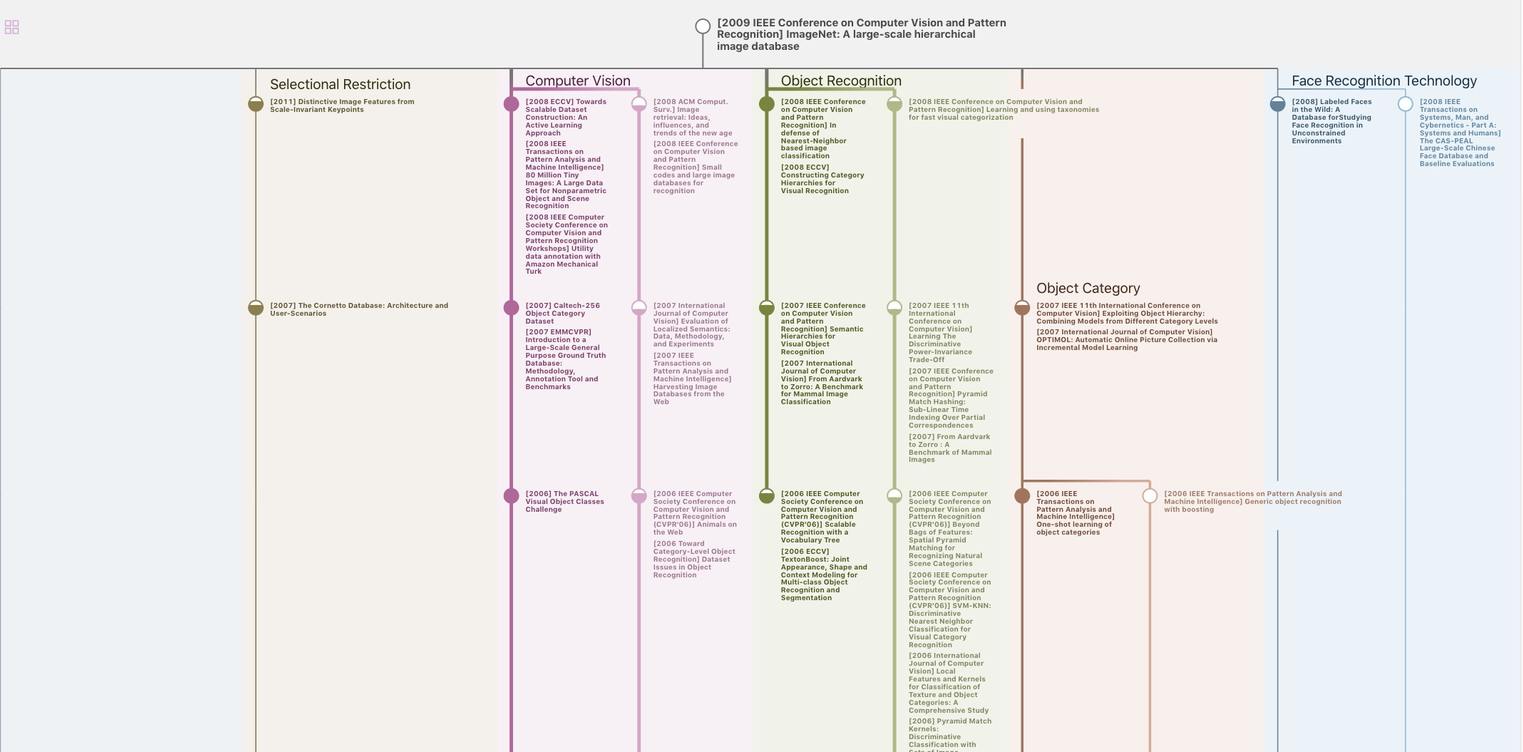
Generate MRT to find the research sequence of this paper
Chat Paper
Summary is being generated by the instructions you defined