A Novel Decomposition and Combination Technique for Forecasting Monthly Electricity Consumption
Frontiers in Energy Research(2021)
摘要
With the share of electricity in total final energy consumption increasing quickly, the world is becoming increasingly dependent on electricity, which makes it more and more important to improve the forecasting accuracy of electricity consumption to ensure the normal operation of economic activities. In this paper, a novel decomposition and combination technique to forecast monthly electricity consumption is proposed. First, we use STL decomposition to obtain the trend, season, and residual components of the time series. Second, we use SARIMA, SVR, ANN, and LSTM to forecast trend, season, and residual component, respectively. Third, we use time correlation principle to improve the forecasting accuracy of season component. Fourth, we integrated the residual component predicted by SARIMA, SVR, ANN, and LSTM into a new sequence to improve the forecasting accuracy of residual component. In order to verify the performance of the proposed forecast model, monthly electricity consumption data in China is introduced as an example for empirical analysis. The results show that after STL decomposition, time correlation modification, and residual modification, the forecasting accuracy of each model has been gradually improved. We believe that the proposed forecast model in this paper can also be used to solve other mid- and long-term forecasting problems with obvious seasonal characteristics.
更多查看译文
关键词
monthly electricity consumption,STL,time correlation modification,ANN,LSTM
AI 理解论文
溯源树
样例
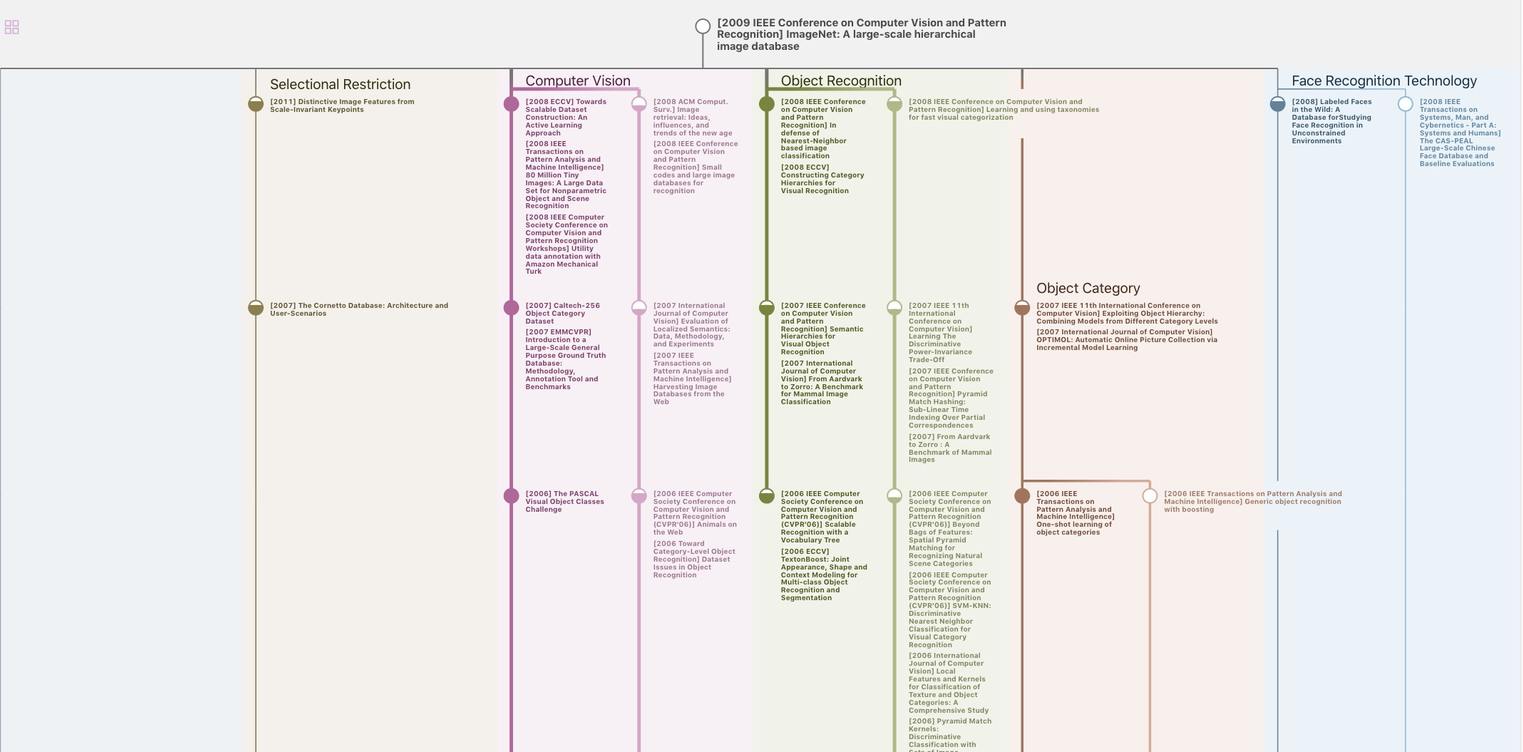
生成溯源树,研究论文发展脉络
Chat Paper
正在生成论文摘要