Big Gaps Seismic Data Interpolation Using Conditional Wasserstein Generative Adversarial Networks with Gradient Penalty
EXPLORATION GEOPHYSICS(2022)
摘要
Regular sampled seismic data is important for seismic data processing. However, seismic data is often missing due to natural or economic reasons. Especially, when encountering big obstacles, the seismic data will be missing in big gaps, which is more difficult to be reconstructed. Conditional generative adversarial networks (cGANs) are deep-learning models learning the characteristics of the seismic data to reconstruct the missing data. In this paper, we use a conditional Wasserstein generative adversarial network (cWGAN) to interpolate the missing seismic data in big gaps. We use the Wasserstein loss function to train the network and use a gradient penalty in the WGAN (cWGAN-GP) to enforce the Lipschitz constraint. We use a pre-stack seismic dataset to assess the performance. The interpolated results and the calculated recovered signal-to-noise ratios indicate that the cWGAN-GP can recover the missing seismic traces in portions or the entire regions, and the cWGAN-GP based interpolation is more accurate than the cGAN.
更多查看译文
关键词
Seismic data interpolation,big gaps,conditional generative adversarial networks,Wasserstein distance,gradient penalty
AI 理解论文
溯源树
样例
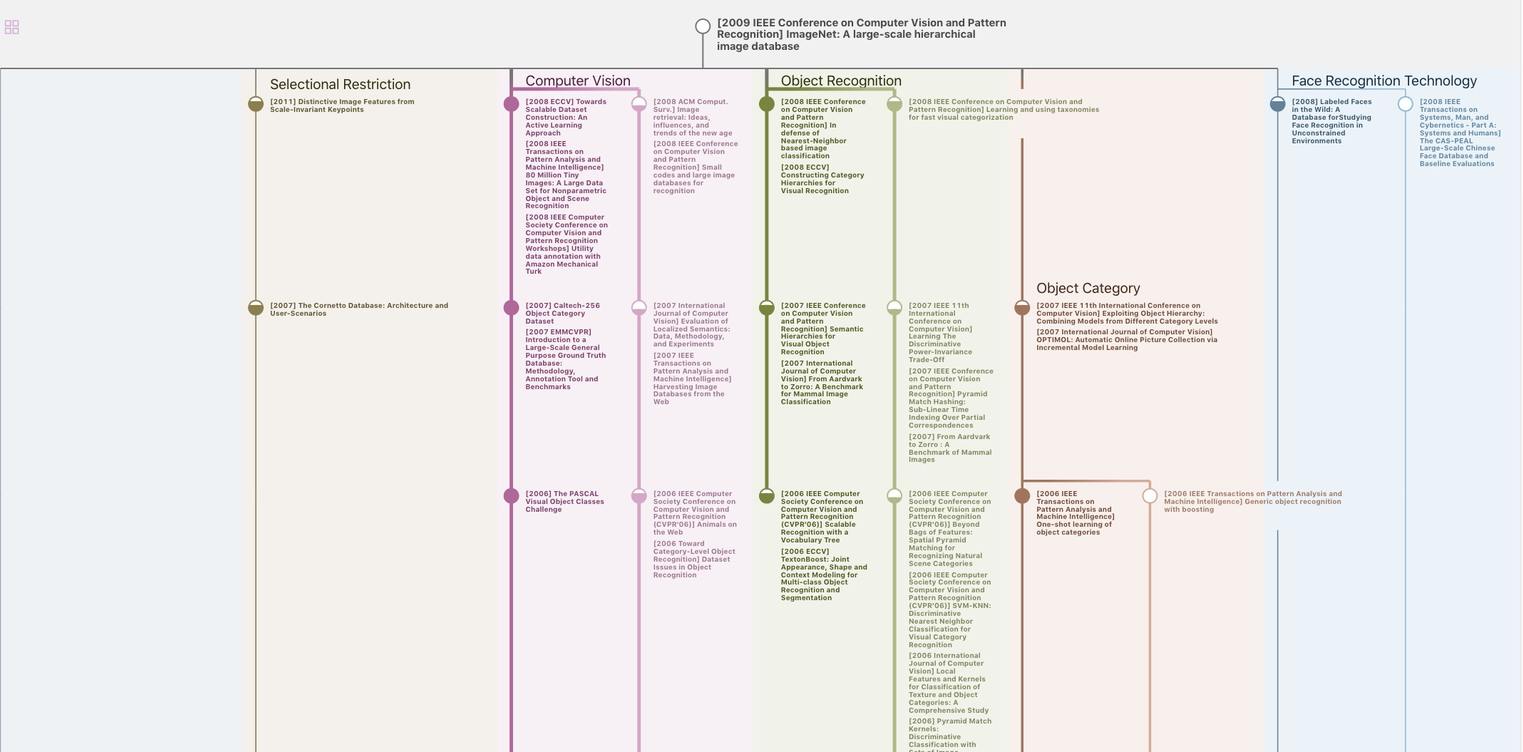
生成溯源树,研究论文发展脉络
Chat Paper
正在生成论文摘要