PET Image Denoising Using a Deep-Learning Method for Extremely Obese Patients
IEEE TRANSACTIONS ON RADIATION AND PLASMA MEDICAL SCIENCES(2022)
摘要
The image quality in clinical PET scan can be severely degraded due to high noise levels in extremely obese patients. Our work aimed to reduce the noise in clinical PET images of extremely obese subjects to the noise level of lean subject images, to ensure consistent imaging quality. The noise level was measured by normalized standard deviation (NSTD) derived from a liver region of interest. A deep learning-based noise reduction method with a fully 3-D patch-based U-Net was used. Two U-Nets, U-Nets A and B, were trained on datasets with 40% and 10% count levels derived from 100 lean subjects, respectively. The clinical PET images of ten extremely obese subjects were denoised using the two U-Nets. The results showed the noise levels of the images with 40% counts of lean subjects were consistent with those of the extremely obese subjects. U-Net A effectively reduced the noise in the images of the extremely obese patients while preserving the fine structures. The liver NSTD improved from 0.13 +/- 0.04 to 0.08 +/- 0.03 after noise reduction (p = 0.01). After denoising, the image noise level of extremely obese subjects was similar to that of lean subjects, in terms of liver NSTD (0.08 +/- 0.03 versus 0.08 +/- 0.02, p = 0.74). In contrast, U-Net B over-smoothed the images of extremely obese patients, resulting in blurred fine structures. In a pilot reader study comparing extremely obese patients without and with U-Net A, the difference was not significant. In conclusion, the U-Net trained by datasets from lean subjects with the matched count level can provide promising denoising performance for extremely obese subjects while maintaining image resolution, though further clinical evaluation is needed.
更多查看译文
关键词
Deep learning,extremely obese patient,FDG PET,noise reduction
AI 理解论文
溯源树
样例
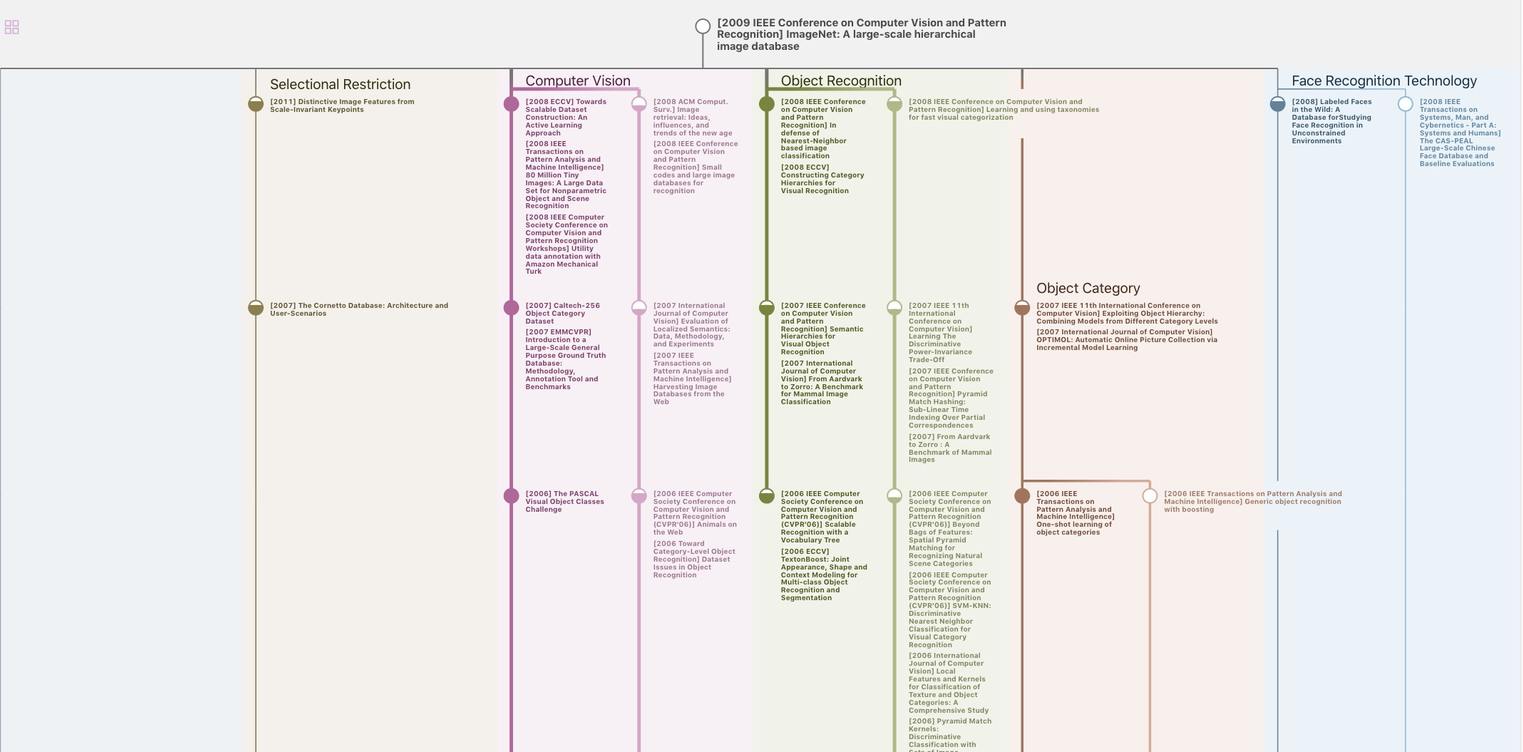
生成溯源树,研究论文发展脉络
Chat Paper
正在生成论文摘要