Sentinel-Guided Zero-Shot Learning: A Collaborative Paradigm Without Real Data Exposure
IEEE transactions on circuits and systems for video technology(2024)
摘要
With increasing concerns over data privacy and model copyrights, especiallyin the context of collaborations between AI service providers and data owners,an innovative SG-ZSL paradigm is proposed in this work. SG-ZSL is designed tofoster efficient collaboration without the need to exchange models or sensitivedata. It consists of a teacher model, a student model and a generator thatlinks both model entities. The teacher model serves as a sentinel on behalf ofthe data owner, replacing real data, to guide the student model at the AIservice provider's end during training. Considering the disparity of knowledgespace between the teacher and student, we introduce two variants of the teachermodel: the omniscient and the quasi-omniscient teachers. Under these teachers'guidance, the student model seeks to match the teacher model's performance andexplores domains that the teacher has not covered. To trade off between privacyand performance, we further introduce two distinct security-level trainingprotocols: white-box and black-box, enhancing the paradigm's adaptability.Despite the inherent challenges of real data absence in the SG-ZSL paradigm, itconsistently outperforms in ZSL and GZSL tasks, notably in the white-boxprotocol. Our comprehensive evaluation further attests to its robustness andefficiency across various setups, including stringent black-box trainingprotocol.
更多查看译文
关键词
Data-Free Knowledge Transfer,Privacy Protection,Zero-Shot Learning
AI 理解论文
溯源树
样例
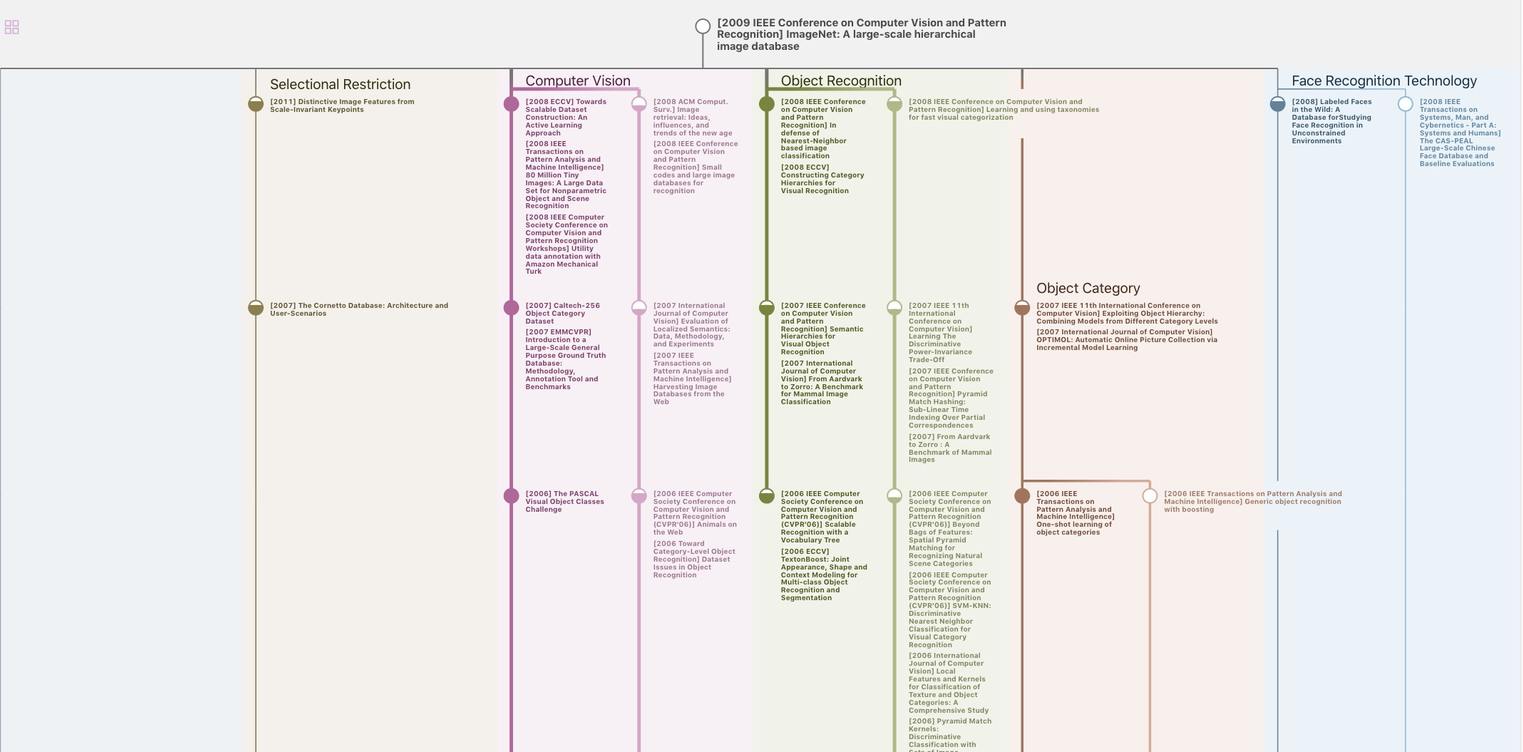
生成溯源树,研究论文发展脉络
Chat Paper
正在生成论文摘要